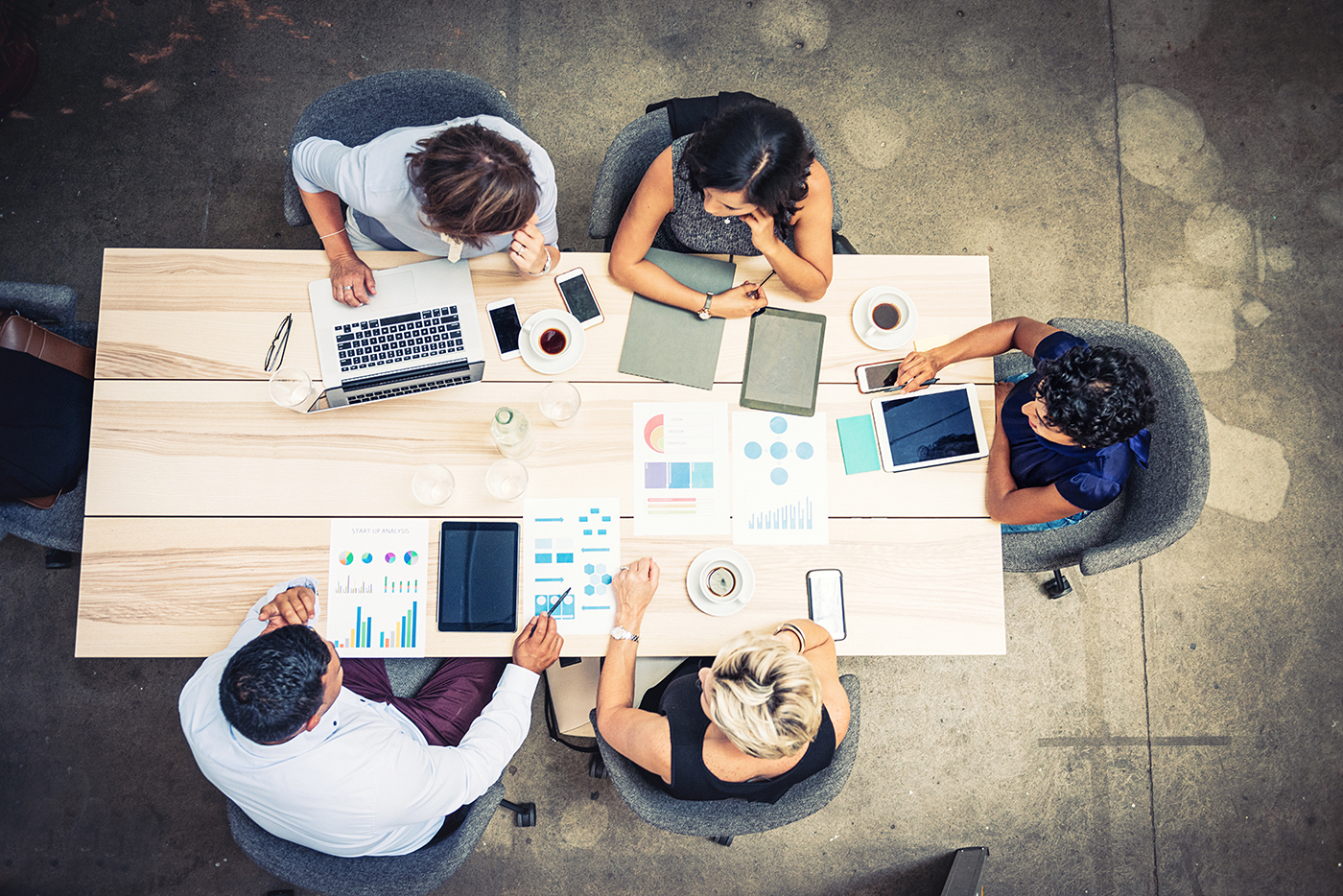
Some of us dream of Olympic glory. Others, of making it big by backing the next meme stock before it goes to the moon.
For the past three decades or so, financial and corporate strategy teams have had a somewhat more prosaic dream: integrated, enterprise-wide risk management (IERM). As the quantification of risk has progressed, moreover, the desire to turn this dream into reality has become more realistic.
Alla Gil
You can find great advice on how to design and build an IERM framework from industry experts who approach this task from an organizational perspective. But one problem is that senior executives often see risk management as the “check-the-box” part of their project development – a viewpoint that could isolate risks from the business.
Despite this potential roadblock, the push for risk integration remains an alphabet soup, gushing from all directions. Recent regulations and their updated versions, like FRTB (the Fundamental Review of the Trading Book) or IRRBB (Interest Rate Risk in the Banking Book), continue what was started by Basel II and III, LCR/NSFR, and, of course, CCAR.
Investors and rating agencies want to see the full picture of risks and benefits, too. Combining analytics with a holistic approach to balance sheet management can help capture business opportunities and improve performance.
Integration Challenges
In the past, risks were assessed in silos: treasury systems, trading desks’ risks, and credit/lending exposures, for example, were often measured using inconsistent methodologies or data sources. Even today, many organizations do not view their liquidity and capital needs in line with profitability and other strategic decisions.
Breaking these silos and creating a truly integrated approach is incredibly difficult. This is especially the case in big, well-established organizations, where to do so means changing systems, reorganizing data and retraining users. It’s also a challenge for small and fairly agile organizations, as they often lack the data and resources needed to build models and analyze results.
To become a true partner at the decision-making table, risk managers must constantly supply the “how” – i.e., they must be adept at quantifying, justifying and articulating the impact of risk (especially unprecedented tail risk) on the firm’s strategy.
Since the results must be delivered to senior decision-makers, the process of developing and implementing IERM must be quick. It should use top-down, high-level analysis, and produce intuitive, insightful and verifiable results. This is where machine-learning (ML) techniques can help.
Leveraging Machine Learning
Explainable ML (like regression with regularization) can generate hundreds of regression-style equations within minutes, quickly linking a firm’s revenue segments to macroeconomic and market environments. It does not require an a-priori hypothesis; rather, out of all potential variables, it seeks and selects a few that will optimally explain each business segment.
ML’s automatically-generated equations can then be edited by business experts. In short, these equations immediately clarify which explanatory variables affect most of the business segment, integrating such dependencies at the enterprise level. They are, moreover, particularly helpful when risk managers find themselves is the uncharted territory of an unprecedented business environment.
In Figure 1, we see an example of an ML-driven IERM exercise. It depicts a case study of an international bank’s monthly revenue data for the past three years, and shows that one-third of all of the bank’s (global) business segments (from, e.g., capital markets revenues to fees from issuance to securitization) have exposure to currency exchange rates in Asia. This kind of analysis represents the first step in a potential partnership between the business and risk groups – and can be achieved very quickly, without a major overhaul of a firm’s infrastructure.
Figure 1: The Impact of Scenario Variables on a Firm’s Performance
Source: Straterix case study of an international bank’s monthly revenue data, 2019 – 2021.
The next step in this process is to generate the full range of scenarios with all explanatory variables, and to then apply the generated equations to all business segments. This will ultimately yield a high-level picture of earnings distribution.
Through this approach, a firm can immediately understand which business segments are the largest contributors to success, and which ones add the most to adverse tails.
Figure 2: Relative Contribution of Business Segments to Success
To build the type of report depicted in Figure 2 (see above), one needs to have the full distribution of the firm’s total earnings.
The weight of each segment in the good outcomes (scenarios around the best 95th percentiles) and the bad ones (scenarios around the worst 5th percentile) must also be identified. Subsequently, for each segment, the ratio of “good” weight to “bad” can be calculated.
The neutral segments will have a ratio close to 100%, which means their contribution to good and bad outcomes is roughly the same, and most likely similar to the expected outcomes.
The winners have the highest ratio and the losers the lowest. (In Figure 2, for example, the business segment B3 has a ratio above 200%, while B2 has a ratio of only 50%.)
Benefits of Comprehensive IERM
Firms that want to have a consistent view of their strategic planning and potential risks should implement ML-driven IERM. The advantages of this approach are obvious: all stakeholders get a comprehensive picture of potential outcomes; risk and finance team members collaborate on a “no-surprises” strategy, including a contingency plan that will allow them to avoid nightmare scenarios; and communication between different businesses gets better, leading to improvements in an organization’s culture.
As we’ve discussed, to build a comprehensive IERM framework, explainable ML must be combined with full-range scenario analysis.
When this is achieved, a firm will have quantifiable measures for both business owners and risk managers to discuss. They can talk about strategic direction, and which businesses to grow. Moreover, they can discuss whether it’s possible to turn “bad” segments into good (or neutral) ones by mitigating some of the tail risks they pose, as well as how all these insights should affect risk appetite and other policies.
In short, ML-driven IERM enables a firm to have a complete picture of its earnings, credit losses, ALM outcomes, and distributions of capital and liquidity ratios – all consistently linked to the same set of macroeconomic and market environments.
Just as importantly, it allows risk managers to explain clearly to senior decision-makers the big picture of what might go wrong, enabling them to see which business segments pose the biggest threats and which indicators could double-up as early warning signals.
Parting Thoughts
IERM is the way to overcome the stereotype that risk managers are there to impose restrictions on the business. When it’s implemented successfully, it can make risk management a true partner in achieving an organization’s goals.
The suggested top-down, ML-based approach to IERM can be implemented quickly and overlaid on top of the existing infrastructure. But this can be achieved only when one employs the full distribution of risks and results, incorporating dynamic relationships between the risk drivers.
It's time to embrace ML strategies that go way beyond big data processing.
Alla Gil is co-founder and CEO of Straterix, which provides unique scenario tools for strategic planning and risk management. Prior to forming Straterix, Gil was the global head of Strategic Advisory at Goldman Sachs, Citigroup, and Nomura, where she advised financial institutions and corporations on stress testing, economic capital, ALM, long-term risk projections and optimal capital allocation.
Article Updated: May 20, 2024