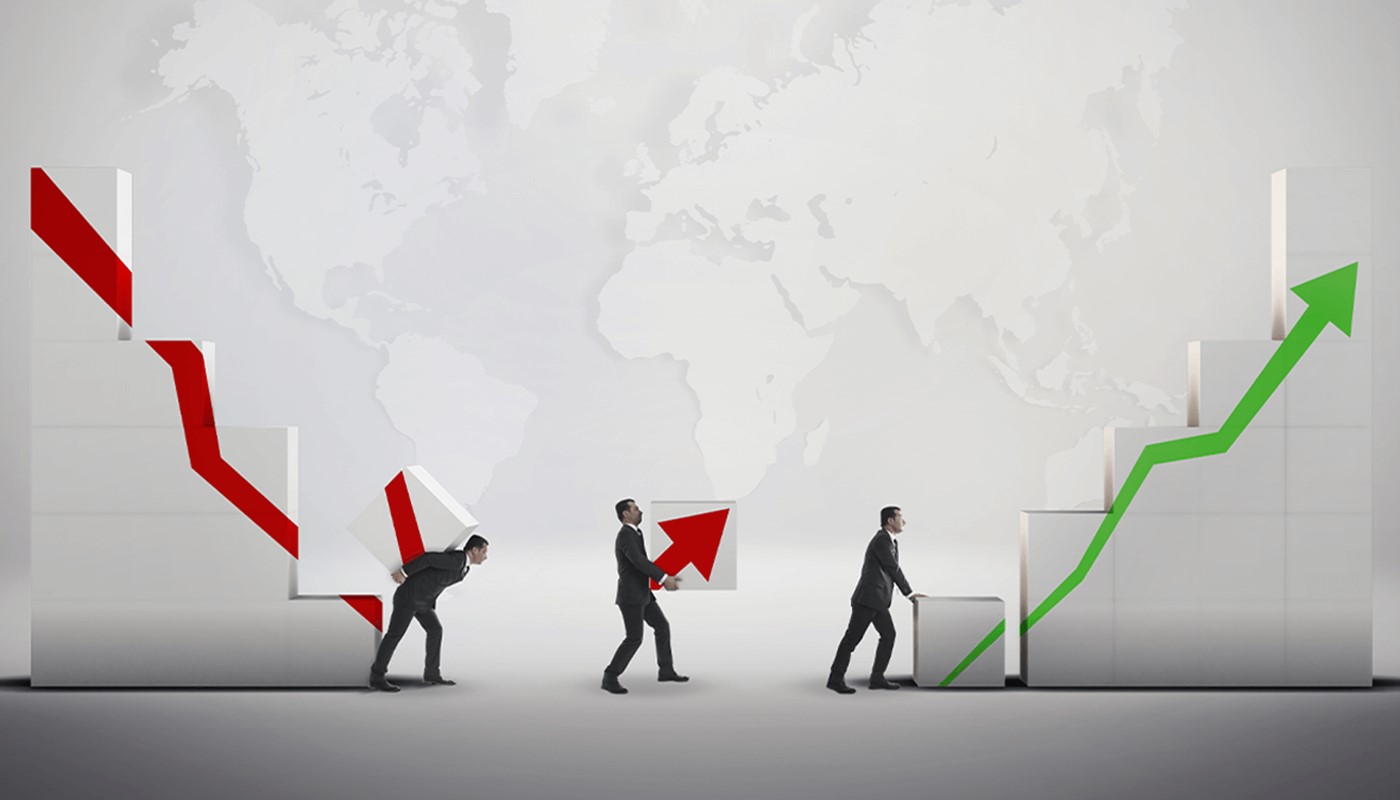
Every investor who's ever read the small print knows that past performance doesn't indicate future performance. Today, in a world in which the relationship between established drivers and revenue has been destroyed by the pandemic, this investment disclaimer seems more relevant than ever.
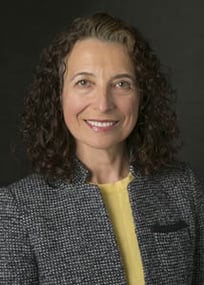
What this means for risk modelers, is that - contrary to tradition - we can no longer rely on financial revenue projections that are based on historical trends. Consequently, everyone must revisit and (most likely) readjust revenue projection models developed prior to pandemic. But how can this be achieved if enough data for this new normal has not yet been accumulated? The answer may not come naturally to financial planners: data mining.
Traditionally, data mining is used in areas like marketing, algorithmic trading, cyber risk and AML. Risk managers, for their part, don't trust models (even smart ones) to select what drives business outcomes.
Modelers are supposed to select risk drivers based on their intuition. This means that data has to fit the modeler's preconceptions - but modeler intuition about what explanatory variables to choose might not capture the big picture, especially during regime shifts.
Machine Learning vs. Modeler Intuition
Explainable machine learning (ML) takes an opposite approach to modeler intuition: it finds a model that fits the data, even when historical trends are broken. Regression-like ML methods, like Lasso or Kernel Ridge Regression, automatically select explanatory variables - effectively performing data mining.
These algorithms find the optimal balance between accommodating outliers (that are ignored by standard regressions) and robust forecasting. The core technique underlying this methodology is based on exhaustive cross validation - i.e., the process in which some (regularization) model parameters are fit by doing repetitive out-of-sample testing.
These methods reduce the potential list of dozens (or even hundreds) of explanatory variables down to a reasonably limited amount (usually, three to five) - making it much easier for business experts to review which variables were selected and deselect the ones that are suspected of spurious correlations.
Cross-validation error is a critical indicator of robust projections, including stress scenarios. It can help modelers to either confirm or expand their intuition in the following way: if this indicator isn't significantly affected by eliminating unintuitive variables, it makes sense to give up a few basis points of the fit for a good narrative. But if eliminating a particular variable causes a substantial increase in cross-validation error, this raises a question of whether modeler intuition misses changed regimes or the causes of outliers.
Let's consider, for example, a revenue segment composed of transactions that are priced based on interest rates. Such a segment has two components: fees from each deal (which depend on the rates' levels) and the number of the deals - i.e., the volume of this segment driven by customers' behavior.
When interest rates are higher, the former component represents a higher proportion of the total revenue, and the modeler's intuition is focused on yield-curve factors as the explanatory variables. Contrastingly, when interest rates drop practically to zero, the fees from each deal represent a small fraction of total revenue - and a modeler must consider very different risk drivers.
Pros and Cons of Data Mining
But what if business modelers have not yet developed the intuition to make smart revenue projections during volatile times? Well, that's where ML-driven data mining can come in quite handy - via, for example, giving them ideas as to which variables can explain the volume of transactions. Data mining, moreover, expands their limited experience in the new market regime, while still offering them the flexibility to test, accept or reject the drivers suggested by the ML algorithm.
At the same time, we have to keep in mind that all such methods still rely on deterministic and formulaic dependency between variables, whereas an organization's revenue may be driven by idiosyncratic factors. This means the correlations with the underlying macroeconomic and market variables are dynamic.
Regression-style dependencies may not be able to capture these correlations - and, consequently, the aforementioned data-mining technique might show poor backtesting or cross-validation results. But even under circumstances where correlations are not robust, it is still critical to understand both the sensitivity of revenue streams and the entire balance sheet (relative to surrounding market conditions) - for planning purposes and stress testing,
This is where the full scenario-analysis distribution method, based on multiple scenarios, comes in handy. Using advanced simulation techniques that incorporate market, credit, climate and operational shocks, scenarios with underlying drivers can be consistently generated for internal, bank-specific segments that don't have a good regression fit. This allows for the incorporation of their intrinsic growth rates and scenario-dependent variations and correlations.
Modelers can subsequently use cluster analysis to find revenue projections (as well as other balance sheet and income statement items) that match specific market conditions. Due to dynamic correlations, implicitly and empirically generated by these scenarios, they might find that in very different market environments (e.g., expected, optimistic and adverse), there are different explanatory drivers for the same revenue segment.
Ideally, discovered risk drivers should be interpreted by business experts using an interactive “cyborg” approach that combines the power of machine learning and human judgment.
Parting Thoughts
Revenue projection is an important challenge for the risk community these days, as was reiterated many times at the recent American Banking Association risk conference. As we have seen, the risk drivers selected for projecting revenues often reflect modelers' intuition on fees and cashflows from an average transaction - but trade volumes or shifts in supply/demand might be more important indicators to track.
Augmented data mining that complements risk managers' intuition allows them to implement an optimal blend of key drivers, specific business knowledge and insights, and state-of the-art, explainable, ML-based data analysis. Risk managers can use this technique to identify the right data and project revenues in a more robust fashion.
Alla Gil is co-founder and CEO of Straterix, which provides unique scenario tools for strategic planning and risk management. Prior to forming Straterix, Gil was the global head of Strategic Advisory at Goldman Sachs, Citigroup and Nomura, where she advised financial institutions and corporations on stress testing, economic capital, ALM, long-term risk projections and optimal capital allocation.
Article Updated: May 20, 2024