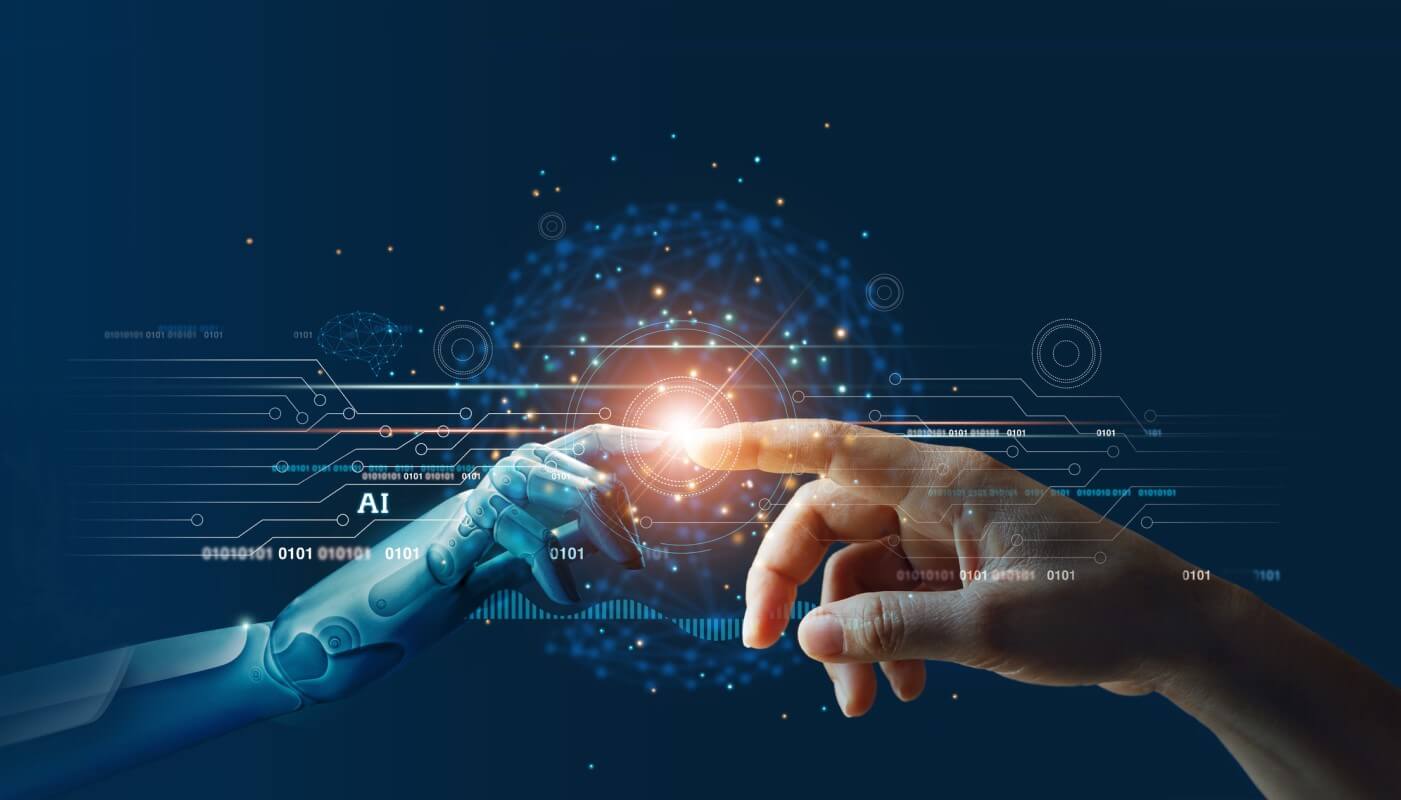
COVID-19 has yielded a crisis of confidence in models employed by financial institutions. Too many proved over-reliant on historical data in a year of unprecedented circumstances, and even well-validated capital and credit-loss models were found wanting.
In short, these models failed financial institutions in 2020 - despite the fact that they had been validated according to well-developed principles encompassing governance (independence, ownership and controls), design, process, data validation and ongoing monitoring.
Model defenders have reasonably argued that it was impossible to anticipate data as anomalous as what we saw during the pandemic based on past trends. On the other hand, weren't these models specifically created to prepare the banking industry for the next unknown?
Thirteen years ago, right before the global financial crisis (GFC) of 2008, capital models across the spectrum left banks with very thin capital buffers. Modern stress testing (based on longer-term, through-the-cycle scenarios) was then introduced to prevent this from happening again: models were, in fact, designed and validated to ensure they worked well in unprecedented scenarios.
But in 2020, amid the pandemic, models “missed the target,” and had to be quickly replaced with subjective management overlays. Indeed, even one of the key requirements of model validation - backtesting - failed to reveal the weakness of these models.
Structural Problems
COVID-19 made historical trends irrelevant, as it was a crisis “unlike any other in modern times.” Consequently, models that were trained on historical data - such as the regression-driven models that many firms employed in 2020 - didn't help at all during the pandemic.
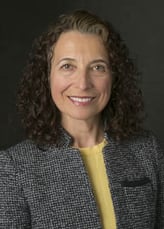
Driven by data from the past 10 (or even 20) years, models that rely on a regression approach suffer from a structural problem: an inability to predict when historical trends abruptly change. They are therefore ill-equipped to evaluate a firm's capital needs and loss allowances over the longer-term horizon which is bound to include turning points
The good news is that thanks to massive government intervention, we've so far managed to avoid catastrophe. Indeed, the latest stress tests show the banking industry stayed well capitalized.
But have government- and regulatory-support measures - such as stimulus packages, payment holidays, and loan forgiveness - stopped potential defaults (and respective capital depletion) or just delayed them? This is an open question that cannot be answered immediately, but the industry must prepare itself for these potential emerging risks.
What we know now is that there is an urgent need to verify whether existing capital adequacy, CECL and IFRS 9 models will be able to realistically assess the impact of post-pandemic events on banks' exposures and credit loss projections.
Retraining existing models on different variables to fit 2020 data won't help, as the future is likely to be quite different from the anomalous events and trends of 2020. So, the structural flaw with the regression approach will remain.
Moreover, we're also faced with the realization that there's no time to redo all these models, and to then validate the revisions. Instead, banks should rely on a top-down, challenger approach to quickly identify which models need to be redone and which are robust enough to survive the next unknown.
How to Improve Model Validation
To revamp validation and reduce model risk amid a rapidly changing environment, we need to step back and look at the big picture, with an eye on resolving three major challenges:
First, existing scenarios that are used for business-as-usual and stress testing are incomplete. Second, a reliable backtesting procedure should be established. Third, regression models must be adjusted to be able to deal with broken trends and data outliers.
There is a wide recognition of the need for a tremendous increase in the number of scenarios, along with a lively debate as to how best to do it: e.g., should we rely on humans or computer algorithms? Each approach has its pros and cons.
Scenarios built by humans are more intuitive, but they are constrained by experience and imagination. Indeed, even scenarios crowdsourced from thousands of experts would be biased.
Machine-generated scenarios, on the other hand, can be much more diverse - but can still miss the tails of risk if simulated using a standard Monte Carlo approach. What we really need is a scenario generator that can run thousands of scenarios. This generator should incorporate tail risks, unconstrained by past experience or imagination.
To get the best of both approaches and generate the necessary number of scenarios, a cyborg approach works best. This hybrid machine-human strategy consists of a Monte Carlo foundation, where the standard steps are overlaid with potential shocks (e.g., pandemics, natural disasters and geopolitical events) that impact all variables in the scenarios.
In this type of approach, the shocks implicitly change the correlations between variables by affecting their values upon impact, allowing us to generate realistic, fat-tailed distributions that overcome the major flaw of standard simulation. The probabilities and severities of such shocks can be estimated using historical data, machine learning or crowdsourcing.
This cyborg technique allows us to generate thousands of scenarios with tractable impacts. It uses explainable and controllable machine-learning techniques for a few specific steps in the algorithmic process.
Moreover, it provides seamless integration of human and machine, expanding human intuition into previously unthinkable scenarios. Consequently, the humans vs. machines dilemma turns into man-plus-machine symbiosis.
This brings us to the second challenge: backtesting. Wide ranging, scenario-based, back-tested models were the only ones that didn't fail during the pandemic. Obviously, they didn't predict the future (no one can!) - but the most effective models included pandemic scenarios.
The models highlighted in the chart below were calibrated a year before the pandemic struck. As you can see, the spike in credit spreads observed in March 2020 was well captured by projected scenarios.
Backtesting of BBB-rated Credit Spreads
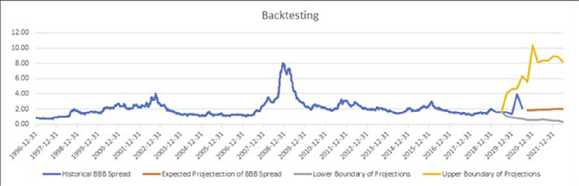
To further improve model validation, existing regression models (hundreds of which are still used by large banks) need to be challenged. Financial institutions need to get ready now for the “new normal,” and, to do so in a timely fashion, model validators can use regression with regularization.
This approach allows us to select the explanatory variables for the equations automatically, with an optimal tradeoff between incorporating outliers and avoiding overfitting. Exhaustive cross-validation and smart variable reduction are used to help us achieve these objectives.
Challenger models can calculate all necessary credit losses and capital and liquidity ratios, and should be used to project balance sheet and income statement segments that are granular enough to have similar behavior and risk patterns. The results should be compared both with internally-designed scenarios and existing model outcomes on respective scenarios.
Models that produce results that significantly deviate from the challenger should be investigated further. Firms that follow this practice can achieve the speedy, high-level model validation necessary for the upcoming, uncertain future.
Standard Model Validation: A Quick History Lesson
Model validation is the core of model risk management (MRM). In the past 10 years, it's received serious attention from senior bankers, both because it was required by regulators and because model errors can be extremely expensive.
Model validation in the 1990s was mainly focused on pricing, trading and structuring. Then, in 2008, Basel II brought about the need for economic capital model validation. However, before this capital accord was fully implemented, the global financial crisis hit.
Structural problems in capital models - including one-year time horizon, point-in-time stress testing and static correlations in standard formulas - were consequently revealed. Indeed, the crisis proved that even models that had passed the Basel II validation process were not fit for purpose.
Regulatory stress testing and new accounting regimes subsequently came along, requiring banks to calculate credit losses for the life of their loans. This led to exponential growth in the number of material models that needed to be validated, especially in capital provisioning, stress testing, loss allowance, liquidity management and strategic planning.
Parting Thoughts
During the global financial crisis of 2008-09, models failed and financial institutions had inadequate capital and liquidity levels. In contrast, amid the pandemic, even the “failed” models enabled us to come through with a well-capitalized banking system - at least, for now. This demonstrates the power of the Federal Reserve's through-the-cycle scenarios in comparison to point-in-time stress testing and one-year economic capital provisions.
Moving forward, the superpower of multi-scenario cyborg model validation should provide us with an accurate glimpse of the future - namely, the width of the cone of uncertainty. This will stop the vicious “paradigm change” cycle, during which accurate and perfectly validated and backtested models fail as soon as an unprecedented event occurs.
Just as cyborgs are hard to kill, shocks will always return (albeit, perhaps, in new and different forms), and we need to make sure that our models are better prepared for the great unknowns of the future.
Alla Gil is co-founder and CEO of Straterix, which provides unique scenario tools for strategic planning and risk management. Prior to forming Straterix, Gil was the global head of Strategic Advisory at Goldman Sachs, Citigroup and Nomura, where she advised financial institutions and corporations on stress testing, economic capital, ALM, long-term risk projections and optimal capital allocation.