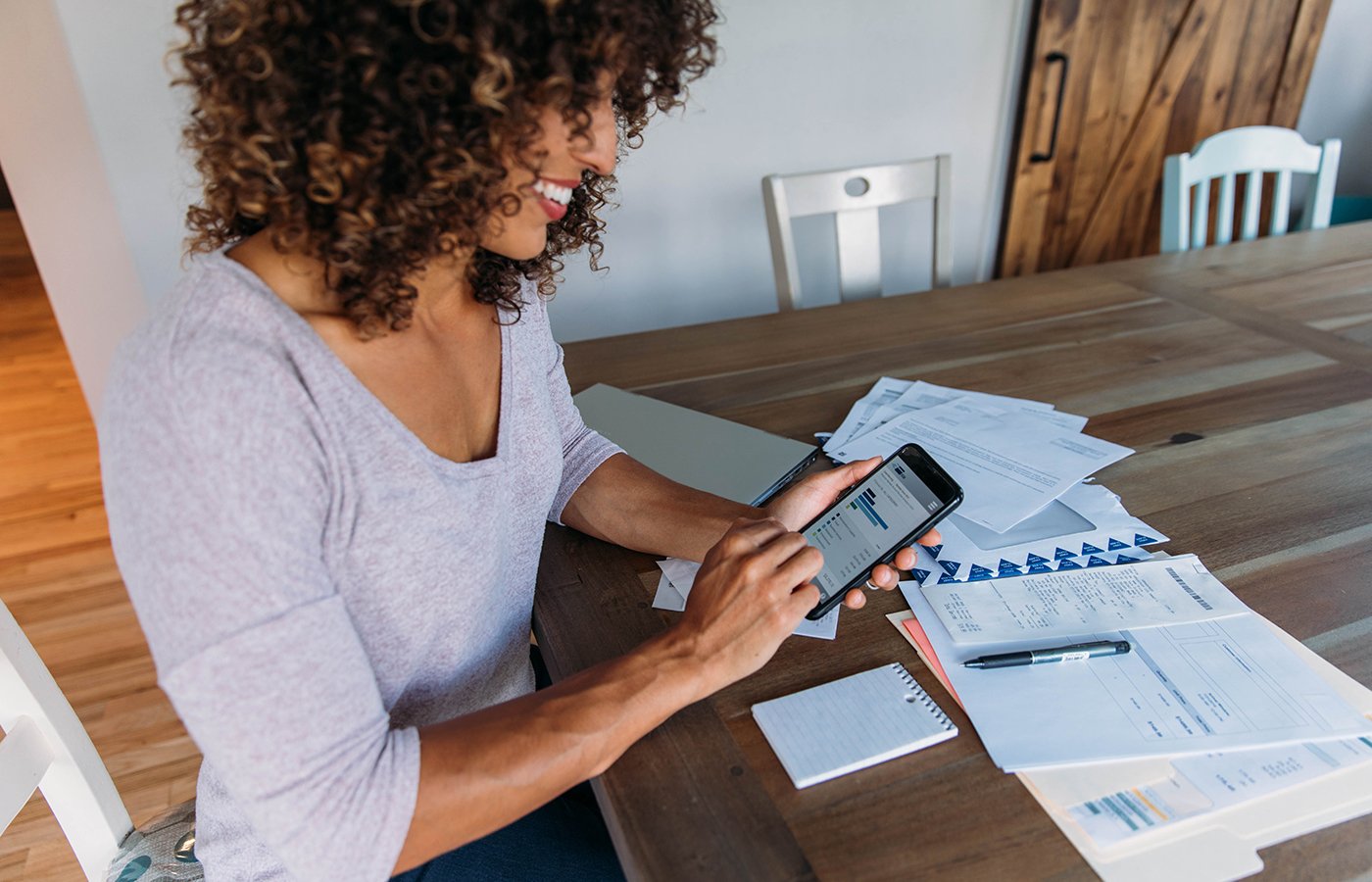
What’s the best approach for assessing a bank’s capital and liquidity adequacy? Moreover, what tools can we use to more accurately forecast tail risk, and do traditional stress tests still have value? These are among the key questions being asked in the wake of sudden and historic bank runs.
There are no simple answers, but there’s one thing we know: a bank is a complex institution with a level of vulnerability commensurate with its specific assets and liabilities. Indeed, each has its own business model and risk profile, with an individual level of exposure to different asset classes.
So, why do we continue to apply standardized regulatory stress tests, and why was there a belief, at least in some circles, that Silicon Valley Bank and Signature bank may have survived if they had been subject to stricter capital adequacy regulation?
Alla Gil
Based on all the facts we now have about the recent bank failures, it’s clear that they would not have been saved even if they had been subject to either of the Fed’s stress-testing exercises: DFAST or the even more rigorous CCAR. That’s because the specific weaknesses that brought about their demise are not fully covered in either test.
For example, SVB's default was driven by its exposure to rising interest rates – but most stress tests over the past decade have only evaluated scenarios with sharply declining interest rates. What’s more, without a deposit outflow, SVB’s exposure to rising interest rates would have remained concealed in their held-to-maturity investments.
Clearly, there is a need for more diverse scenarios covering a wider range of market environments, as well as for comprehensive and consistent capital adequacy measurement.
Both of these issues can be addressed by automatically generating full-range scenarios and by then linking those scenarios to banks’ exposures to project them consistently. The generated scenarios should not only incorporate macroeconomic, market and operational shocks but also take into account their ripple effects, with implied dynamic correlations between the risk drivers.
To discover stress scenarios specific to the institution, all interactions across risks must be captured, and it is therefore necessary to calculate all of an institution’s key performance indicators (KPIs). Through the full range of scenarios, one can naturally obtain the empirical distribution of these KPIs and expand risk measures to incorporate tail risks.
To understand the steps we need to take to better account for tail risks in the future, let’s consider a few of today’s most common risk measures.
One is economic capital, which should cover the losses above and beyond expected loss for reasonably foreseeable amounts. It can be easily estimated from the full-range distribution by (1) combining market, credit and operational losses on all scenarios at each point in time; (2) constructing a respective empirical distribution at the desired time horizon; and (3) obtaining the difference between expected and the 99th (or other specified) percentile of such a loss distribution.
Risk-adjusted return on capital (RAROC) and value-at-risk (VaR) are two other traditional risk measures. RAROC should incorporate the tails of return distributions, which can subsequently be used for strategic analysis. VaR, meanwhile, must consider historically unprecedented outcomes.
Learning From the FRTB
The Basel Committee on Banking Supervision’s Fundamental Review of the Trading Book (FRTB) regulation aims to addresses the limitations of the current VaR methodology by including stressed scenarios in risk measurement and management. It centers on market risks in trading activities (including stressful situations), and it calculates both stressed VaR and expected shortfall (ES) – or expected tail risk exposure.
While the U.S. implementation of the FRTB has been postponed (the Federal Reserve is still finalizing the details of the regulation for U.S banks), its primary aim of enhanced stress testing of trading books is clear.
However, the FRTB does not address one key question in stress testing exercises: namely, which stress scenarios should be applied to different banks and their trading activities, and what correlations should be assigned between the risk drivers affecting trading positions? Under the FRTB’s standardized approach (SA), these parameters are usually prescribed, while the internal models approach (IMA) allows institutions to propose their own suggestions for stress correlations.
In either case, selection of these stress correlations must be specific to each firm’s business model, risk profile and exposure to different markets and asset classes. Only then regulators can overcome the “one size fits all" approach and adequately capture the specific vulnerabilities and risks of individual institutions and accurately assess their resilience.
How to Find Stress Correlations
Estimating all KPIs in all scenarios is a significant challenge, however – even for big banks. Moreover, it simply may not be feasible for small and medium-sized banks.
Consequently, there is a need for a new-and-improved process for selecting critical scenarios that are most relevant – not only for assessing banks’ capital and liquidity adequacy but also for incorporating stress correlations into trading book models.
The first step in this process involves grouping similar individual exposures into uniform segments, such as loans and deposits of various product types and market segments; trading strategies with similar behavior patterns; and asset segments of the investment portfolio.
The second step is to link these segments to the generated full-range scenarios. As even small banks may have dozens of such segments, manual derivation of equations to link them to the scenarios would take months and is not practical. Therefore, this linking process should be automated.
The third and final step consists of projecting all segments on the scenarios, calculating KPIs and analyzing their outcome distributions. Indeed, this approach allows banks to calculate the implied correlations for these specific outcomes from multiple scenarios.
It might also come in handy for regulators. In the case of FRTB, for example, regulators will be able to hand over (for SA) or verify (for IMA) stressed correlations across all banks, emphasizing their variety of trading strategies specific to their portfolios.
To achieve this, banks will need to calibrate the equations for each pure trading strategy (e.g., long-short equities, currencies, interest rates, volatility, and correlation) and then link each strategy. Behavioral and performance outcomes for all scenarios must also be projected.
Subsequently, to calculate the expected shortfall in the tail of many different trading strategies in various banks, one just needs to apply the respective weights of each strategy in each bank’s trading portfolio, which will ultimately yield the full distribution of such portfolio outcomes.
The stress correlations can be deduced from a range of scenarios (50-100), leading to tail risk for a specific portfolio. This process will enable regulators, rating agencies and investors to (1) have a comprehensive view of expected shortfall in banks’ trading books; (2) consistently compare banks positions; and (3) easily integrate the exposures to get a system-wide perspective.
Once a few most relevant reverse stress scenarios have been identified through this top-down approach, these scenarios can be plugged back into the detailed models to perform the full bottom-up risk assessment.
Parting Thoughts
Financial institutions have different business models, risk profiles and exposure to different markets and asset classes. As a result, the impact of a particular economic scenario can vary significantly among institutions.
A "one size fits all" approach does not adequately capture the specific vulnerabilities and risks of individual institutions. This could lead to inaccurate assessments of their resilience, misleading results and incorrect conclusions.
Despite this, many upcoming regulations are still scheduled to be implemented as silos, with the focus on specific risk exposures such as market, credit or liquidity risk.
Regulators should instead demand a customized approach to measuring the integrated risks we see across all banking activities. Banks’ board of directors, moreover, should push their institutions into adapting idiosyncratic reverse scenario analysis, driven by discovery of adverse KPI outcomes and hidden pockets of risk.
Alla Gil is co-founder and CEO of Straterix, which provides unique scenario tools for strategic planning and risk management. Prior to forming Straterix, Gil was the global head of Strategic Advisory at Goldman Sachs, Citigroup, and Nomura, where she advised financial institutions and corporations on stress testing, economic capital, ALM, long-term risk projections and optimal capital allocation.
Topics: Modeling