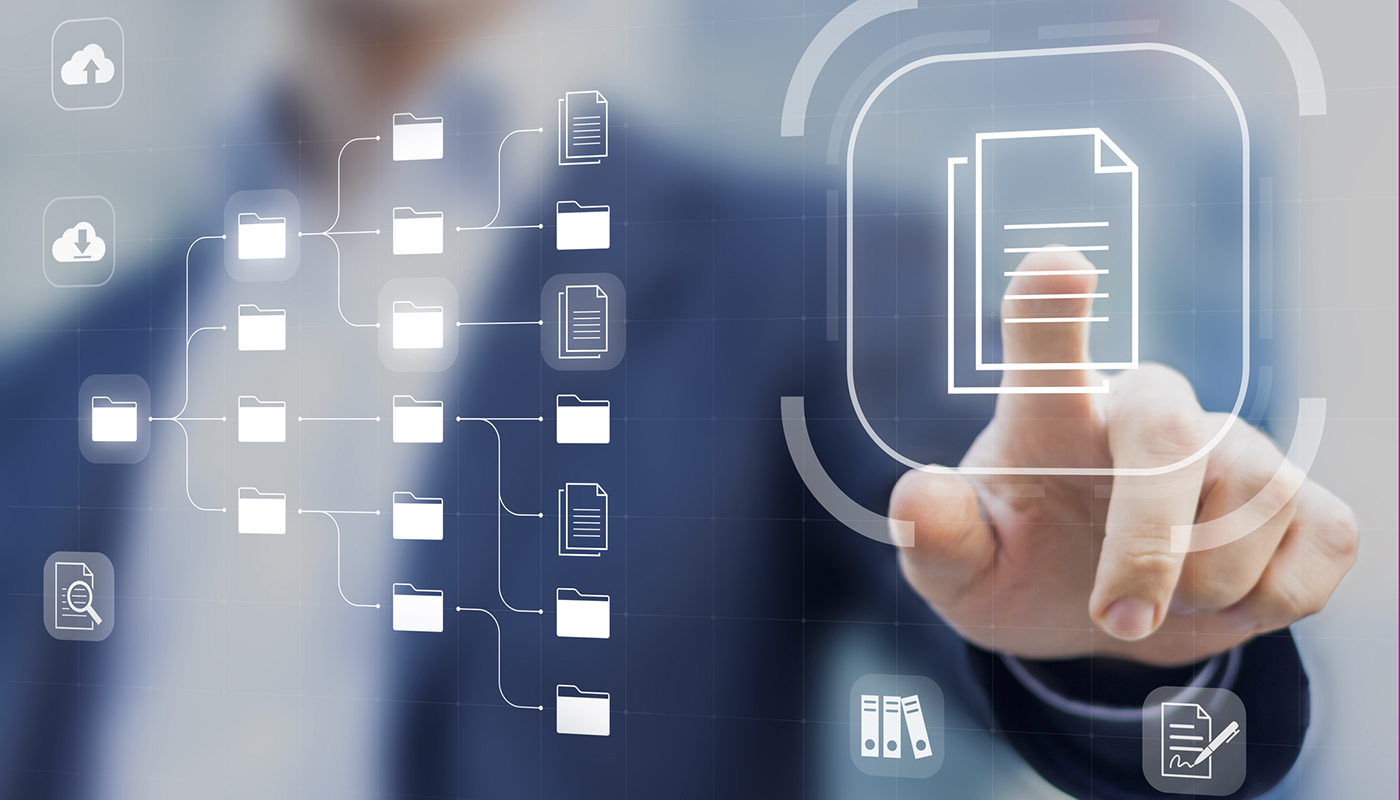
The digitalization of businesses has the potential to unlock new opportunities for increased profitability and reduced risk. However, to reap the rewards of this transition, firms need to take a top-down, big-picture approach that makes use of explainable machine learning (ML).
Alla Gil
Before delving further into the steps necessary to achieve digitalization, it’s helpful to clarify exactly what we’re discussing. In short, digitalization is the use of digitized information – in conjunction with new computerized technologies – to improve business processes, create new revenue streams and enhance productivity.
It's one of two components of the so-called digital transformation – the shift from brick-and-mortar banking to digital data, processes and systems that is sweeping through financial services. Digitization, or the conversion of data into a digital format, is the other.
So far, digitalization has been mainly implemented in consumer finance, compliance, anti-money laundering (AML), and cyber risk – as well as in finance and accounting workflow analyses. Eventually, it’s expected to be applied to broader risk management functions, like stress testing and asset-liability management (ALM).
Current Uses, and Optimism for the Future
Digitalization is already helping financial institutions combat fraud and cyber risk. To detect credit card fraud or a potential cyberattack, an ML algorithm must be programmed not only to identify the IP address of each login but also to search for anomalies – like an out-of-pattern transaction type or a transaction that originated in an unusual geography.
Digitalization can also be used to identify market manipulation by traders, as has recently been alleged in the case against SMBC Nikko. Indeed, banks have already digitized (at least) daily data on trading positions’ sensitivity to market moves. This information combines trading strategy, risk limits and behavioral patterns of a specific trading desk.
Using this data, ML-based digitalization can establish a link between market variables and trading positions, flagging out-of-pattern trades. Just like a credit card transaction may be declined if it’s out of pattern, a questionable trade may be proactively checked for appropriateness.
Similar methodologies can be used for stress testing. For example, to project potential trading results on future market scenarios, banks can use the same ML-driven models that link trading positions to market variables. Since it can also project banks’ lending patterns in a multitude of future scenarios, this approach can address the calls for the new stress tests that incorporate banks’ own behavior.
Advancing Scenario Analysis
The transition toward digitalization also applies to scenario analysis.
Though scenario planning has been a leading tool in strategic foresight, constructing scenarios using traditional methods is challenging when a situation lacks analogies from the past.
Today, for example, we’re witnessing a combination of impactful events – including the pandemic, supply-chain disruptions, inflation, and the Russia/Ukraine war – that have never previously occurred simultaneously.
Moreover, ambiguity about government response to future crises has complicated matters further because banks continue to face uncertainty about how a new round of shocks could impact their strategies. But these challenge can be efficiently addressed through comprehensive, ML-driven scenario analysis.
To better understand full-range scenario analysis, we can compare it with different approaches in education.
Math and science, for example, teach rules and laws of nature and formulas that connect various variables. History and literature teach about various actual and fictional (but easily imaginable) scenarios, as well as how to adapt to them. Lastly, science fiction outlines scenarios that never happened before but are possible, and for which we need to be ready.
When some of these scenarios come true, we are not caught off guard.
A Step-by-Step Process
The full digitalization process consists of three steps: first, readying your formulaic dependencies – e.g., calculation of NII, RWA, ECL, LCR/NSFR and capital ratios from underlying scenario variables; second, preparing for scenarios you have seen before (whether good or stressful); and third, learning how to deal with dependencies, shocks, and consequences you have never seen before.
Most firms, and especially CCAR banks, have already taken the first two steps. To implement the third step, banks need to do the following:
1. Link funded loan levels and unfunded commitments – as well as deposits and all PPNR segments – to macroeconomic and market variables using explainable ML techniques like regression with regularization. (To avoid the biases of modelers’ experience, it’s important to omit a-priori hypotheses.) Through this approach, a firm will be able to see if and how geopolitical shocks and inflation impact its credit risk and deposit run-offs; how unemployment and various productivity indices impact PDs (via potential mass downgrades or prepayments) and LGDs (through adjustment of collateral values); and which factors affect the behavioral patterns for EADs.
2. Generate the full range of scenarios (including historical and potential external shocks), and incorporate their knock-on effect on all variables. This automatically generates potential dramatic changes in long-established historical correlations between asset classes, similar to what we are observing today.
3. Apply dependency equations developed in step 1 to thousands of scenarios generated in step 2. This will simultaneously yield the outcomes for ALM (scenario-consistent levels of loans and deposits and respective interest rates), credit losses (scenario-adjusted transition probabilities, default and prepayment rates, and collateral values), and capital ratios (scenario-specific RWAs and other balance sheet items).
Banks that complete all three steps of this digitalization process will be able to pre-emptively assess impact of various scenarios; proactively develop response mechanism in the form of lending decisions, loan and deposit pricing, and liquidity buffers; and prepare adequately for the long-term consequences of potential chaos and disruptions, regardless of whether government will come to the rescue or not.
Parting Thoughts
Banks’ digital transformation must go way beyond big data processing. While digitization is well under control, the second step – the digitalization of risk management – is the way to develop robust strategies that can pre-emptively adjust to even unprecedented futures.
ML-powered digitalization will enable financial institutions to build an integrated, enterprise-wide platform, through which they will not only be able to monitor risk continuously and receive early warnings indicators but also improve resilience. Moreover, it will help them navigate uncertainty with a high level of confidence.
Alla Gil is co-founder and CEO of Straterix, which provides unique scenario tools for strategic planning and risk management. Prior to forming Straterix, Gil was the global head of Strategic Advisory at Goldman Sachs, Citigroup, and Nomura, where she advised financial institutions and corporations on stress testing, economic capital, ALM, long-term risk projections and optimal capital allocation.
Article Updated: May 20, 2024