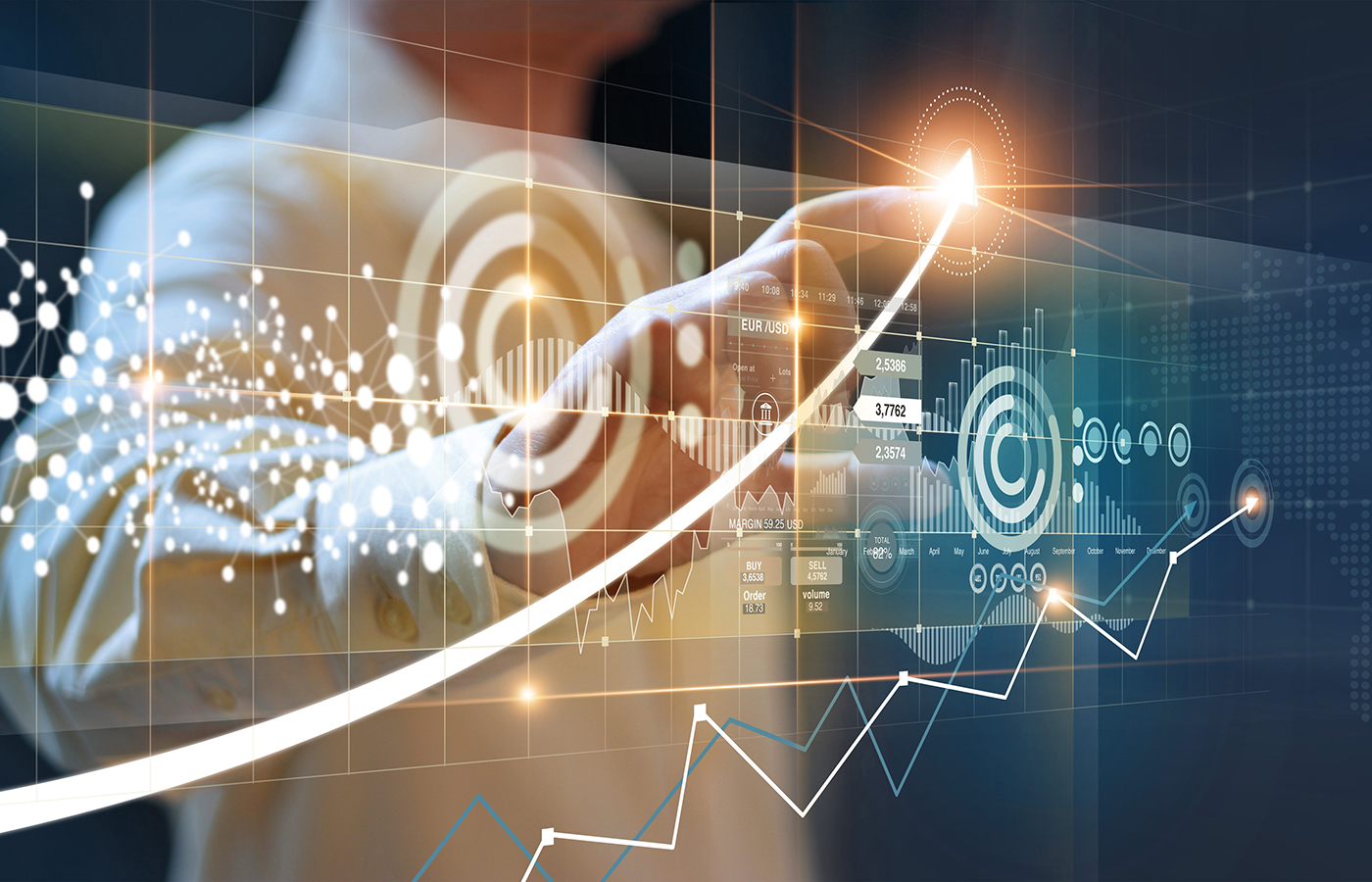
The problems with credit loss-projection methodologies that rely on historical data have been illuminated over the past three years. Regression-based credit risk models have, indeed, been failing, thanks largely to volatile market conditions, ranging from the COVID-19 pandemic to rising geopolitical risk to inflation and increasing interest rates,
Complicating matters further, at the outset of 2023, all major research publications are predicting tough economic times ahead. Risk professionals, of course, can’t wait until markets stabilize to accumulate enough new data for loss estimation. Instead, in a world where unpredictability is permanent, one must identify tail risks with realistic probability.
While economic forecasts range widely and change frequently, the common theme is that this year’s macro environment will face significant obstacles, with stagflation and a recession potentially on the horizon. When it comes to credit risk, such unprecedented uncertainty creates a major challenge when selecting scenarios for “reasonable and supportable” forecasts, as required by the CECL and IFRS 9 reporting standards for expected credit losses (ECL).
Alla Gil
The traditional practice of relying on economic predictions and subjective management overlays to determine ECL can produce deceptive results – and has proved inadequate in turbulent times. Risk managers who want to more accurately forecast ECL amid volatile conditions should (1) estimate potential losses on a full range of future scenarios; (2) find the expectation of this distribution; and (3) discover scenarios leading to relevant outcomes.
The critical question here is how to estimate ECL using multiple scenarios.
To start, it helps to understand that there are three major components of credit risk: probability of default (PD), loss given default (LGD) and exposure-at-default (EAD). These components all have their own dynamics, and their correlations are dramatically different under normal versus stressed conditions.
There is strong empirical evidence, for example, that when PDs increase during an economic downturn, so do LGDs and EADs. To capture the dynamic behavior of these components, let’s now consider how they can be linked to the macroeconomic and market variables of different scenarios.
Projecting Probability of Default
PDs are mainly impacted by the credit rating of loan obligor or bond issuer. It is therefore important to include potential downgrades in scenarios.
A historical rating transition matrix is a good start for a PD estimate. But we know that downgrades issued by rating agencies usually lag behind actual deterioration in a company’s credit quality. The same holds true for banks’ internal loan ratings and for ratings of private companies. (For publicly-traded companies, typically, a downgrade is only issued after a traded credit spread increases.)
Historical rating transition matrices should be adjusted based on the current macro and market variables for each scenario. If a scenario experiences a macroeconomic, geopolitical or industry-specific shock, a jump in credit spreads might lead to an accelerated rating transition.
Scenarios that imply increased downgrade probabilities can serve as the early warnings for upcoming changes in PDs. When multiple obligors get downgraded because of a systemic shock, the correlations between their defaults and downgrades go up, impacting the overall portfolio.
Projecting PDs on the scenarios therefore requires two steps.
First, the stable market dynamics should be modeled using the historical average transition matrix, which reflects idiosyncratic changes in the credit quality of obligors.
Second, if a particular scenario experiences macroeconomic, geopolitical and/or industry-specific shocks at a given time point, the credit spreads must be adjusted according to their behavioral patterns.
Depending on the shock event, industry sector and rating, these shocks can generate massive additional downgrades or push credit spreads closer to a default boundary.
LGD and EAD Guidance
Modeling LGDs consistently with the scenarios should reflect three major drivers of LGDs: the seniority of the obligor, collateral quality, and the values of the assets.
LGDs reflect the market value of assets and other collateral in the recovery. When a company defaults, its debtholders are supposed to take control. However, in practice, seniority defines the portion of a firm’s assets to which creditors might be entitled in the event of a default. I
In a stressful environment, the recovery after default can be substantially lower than originally assumed, and LGD can therefore be substantially higher. For example, even if inflation shock (combined with economic stagnation or a looming recession) pushes up the value of the asset, dried up liquidity and lack of demand could mean no buyer would pay the elevated price.
Generated scenarios should include all variables that drive the value of recovery in the event of a default. Consequently, for scenarios with stressful shocks (that have already resulted in increased downgrades and defaults), LGDs would go up as the recovery projections go down.
Initial EADs, meanwhile, can be measured as the current funded level of the loans. As scenarios progress, the funded levels of revolving loans and credit lines should be adjusted based on observed behavior.
Obligors may behave differently based on the type of loans they take. A loan is more likely to be prepaid, for example, in favorable market conditions – i.e., when an obligor has the opportunity to get a new lower-interest loan. On the other hand, under adverse conditions when the credit quality deteriorates (either as part of the overall economic situation or for an industry-specific reason), obligors will usually take a portion of their unfunded commitments.
In the event of a default, an obligor will try to draw on as much of unfunded commitments as allowed by loans’ covenants. Therefore, in stressed scenarios, EAD increase together with PD and LGD.
Through the use of full-range scenario analysis, risk managers can project credit exposures the same way they play out in real life. This approach incorporates the impact of shocks on credit spreads, as well as equity, commodity, real estate and other market variables. Moreover, it allows for dynamic modeling PDs, LGDs and EADs, and shows their capital and liquidity implications – even for unprecedented scenarios.
Parting Thoughts
To estimate potential credit losses with realistic probabilities, and to analyze extreme events consistently with the rest of the distribution, full-range scenario analysis is needed.
In a dynamic market environment, realized future trends could be far away from the projected ones. Since tail outcomes impact a portfolio’s expected values, it is important to measure the entire distribution of credit portfolio exposures,
The looming recession might provide us with the new data to estimate ever-changing behavioral patterns. But we can’t let the future surprise us, and we can’t wait for an economic collapse to happen to gather new data.
Models driven by full-range scenario analysis not only learn from the past but also empower risk managers to be better prepared for future unprecedented scenarios.
Alla Gil is co-founder and CEO of Straterix, which provides unique scenario tools for strategic planning and risk management. Prior to forming Straterix, Gil was the global head of Strategic Advisory at Goldman Sachs, Citigroup, and Nomura, where she advised financial institutions and corporations on stress testing, economic capital, ALM, long-term risk projections and optimal capital allocation.
Topics: Modeling