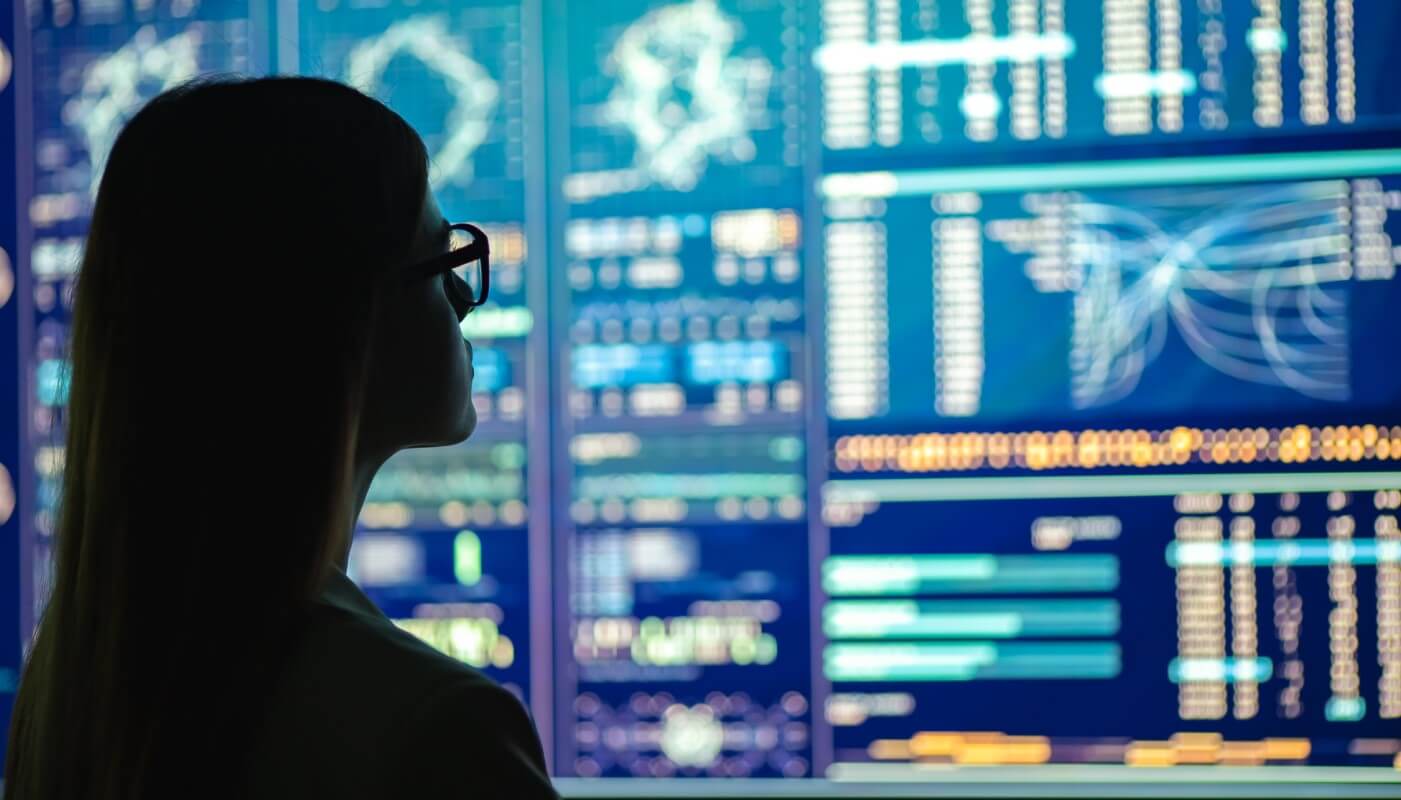
As the implementation deadline for the Current Expected Credit Loss (CECL) accounting standard nears, financial institutions are now feeling the compliance heat. However, calculating future losses is a multi-layer challenge convoluted by the heavy tails of credit loss distribution.
The underlying principles of CECL have the same objective as stress-testing exercises: to help institutions prepare for unexpected, adverse events. It changes the way the allowance for potential credit loss is calculated, and the key question is how to determine the right size of the allowance.
This is complicated by the fact that CECL does not mandate a one-size-fits-all approach. Indeed, the standard allows for a variety of implementation approaches, and it is critical to select one that not only meets the loss allowance requirement but also aligns with the size and complexity of your institution.
Currently, the standard approach is to select one baseline scenario and estimate losses for the life of all loans or loan segments. More sophisticated institutions might generate a few economic scenarios (baseline and one or two good and bad ones), estimate the probability of each scenario, calculate life-long expected losses on each, and combine these multiple outcomes by applying the same probability weights that were used for scenarios.
However, neither of these basic approaches produce the correct estimate of expected credit loss, and there are several reasons why.
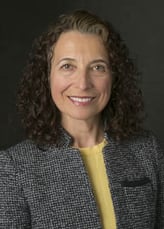
First, guessing one “right” scenario upfront is almost impossible. A small number of scenarios, including compliance-driven scenarios and scenarios based on fundamental theory, are likely to miss the most important and relevant risks for the bank. To see the full picture, one needs to generate thousands of scenarios with multiple combinations of risk factors and their knock-off effects.
Second, credit loss is a non-linear function of the underlying scenario. Just because a scenario is expected, doesn't mean the same holds true for the respective credit loss. Credit loss distribution has heavy tails, especially on a portfolio level, driven by increasing correlations between the downgrades and defaults in adverse scenarios. These tails impact the expected value of credit loss distribution. Therefore, unless the entire distribution of outcomes is obtained, your ECL calculation can be deceptive.
Expected loss forecasts should be calculated exactly the way they sound - as expectations of future credit losses. This means, first, a firm must generate the full distribution of potential credit losses (including unprecedented outcomes) on multiple scenarios, and then average them. The probabilities of the outcomes are to be deduced from bank-specific distributions of credit losses - and not from generic distribution of economic forecasts.
Getting expected credit loss right is important for banks of any size and complexity, and even smaller institutions can achieve this objective using a full distribution, multi-scenario approach.
The Buffer Dilemma
Estimating expected credit losses correctly is a necessary, but not sufficient, condition for determining the size of the buffer against future adversity. This involves a tradeoff between the size of the buffer today and the future volatility.
On the one hand, you can try to keep your ECL at its current level and risk the future volatility of your provisions. On the other hand, you can try to absorb this volatility at the inception of your ECL forecasts and take advantage of the initial smoothing relief, but risk an adverse reaction from investors and equity analysts. (“Why are you keeping such a large buffer?” “Are you hiding a potential time bomb in your balance sheet?”)
The optimal buffer is one that serves three functions.
First, it should absorb some of the future allowance volatility, without representing a real burden to a bank.
Second, the scenarios that were used to arrive at the full ECL distribution should provide early warning signals, highlighting when the buffer should be reduced or increased ahead of big changes in market trends.
Last but not least, a bank should be able to easily and transparently explain (to its relevant constituents) why it made its choice.
The goal of provisions should not be the precise calculation of the potential future needs, but, rather, finding an optimal buffer that will not be an unnecessary burden today and will mitigate the volatility of earnings going forward, through sensitivity and scenario analysis.
Benefits of the Multi-Scenario Approach
It is only after the completion of all these steps - generating thousands of scenarios, finding the proper expectation of credit loss that incorporates stress events, and identifying the optimal trade-off between buffer and volatility - that the baseline scenario can be selected. This multi-scenario approach makes it easier to answer regulators' questions as to why a specific scenario has been chosen, rather than a different one.
Since this strategy produces the probabilities of all other forecasts specific to the bank, one can now easily answer the auditors' questions about expected losses (e.g., “why do you expect 2% loss, and not 3.5%?”) and credit loss distributions. Answers can be supported with the underlying scenarios, reducing the subjectivity associated with qualitative adjustments.
This will also address a potential risk of procyclicality (i.e., a scenario in which economic conditions deteriorate, CECL provisions go up and a vicious cycle begins) - exactly what proper implementation of CECL should prevent.
Yet another advantage of the multi-scenario approach is that it mitigates the thorny problem of data plaguing other methods. In the wake of the past 10 years of stable markets, using historical data to set reserves would now likely produce unrealistically low levels of capital.
Moreover, many banks don't even have sufficient history: either they started collecting the data after the crisis, or the nature of their business and their customers (as well as the market environment) changed so much as to make the long-term history irrelevant. The famous maxim that “history is not a good predictor of the future” is very relevant today. Indeed, it's not only true for investment performance but also for your PDs, LGDs, EADs and the expected life of loans.
Parting Thoughts
The new standard truly represents a blessing and a curse. It's a blessing because its longer-term horizon presents an opportunity for a holistic approach that connects growth plans and risk appetite with lending policies and stress testing. It's a curse because it requires a fair amount of additional work on data and models.
The bottom line is that CECL requires you to forecast, quantify and justify. You can do all of this more efficiently using a holistic approach that doesn't try simply to guess a few scenarios (base, good and bad), but instead analyzes a multitude of forecasts through automated reports. As an additional benefit, this type of approach would also yield early warning signals, ensuring that loss allowances can be adjusted before they become extremely expensive.
Alla Gil is co-founder and CEO of Straterix, which provides unique scenario tools for strategic planning and risk management. Prior to forming Straterix, Gil was the Global Head of Strategic Advisory at Goldman Sachs, Citigroup and Nomura, where she advised financial institutions and corporations on stress testing, economic capital, ALM, long-term risk projections and optimal capital allocation.