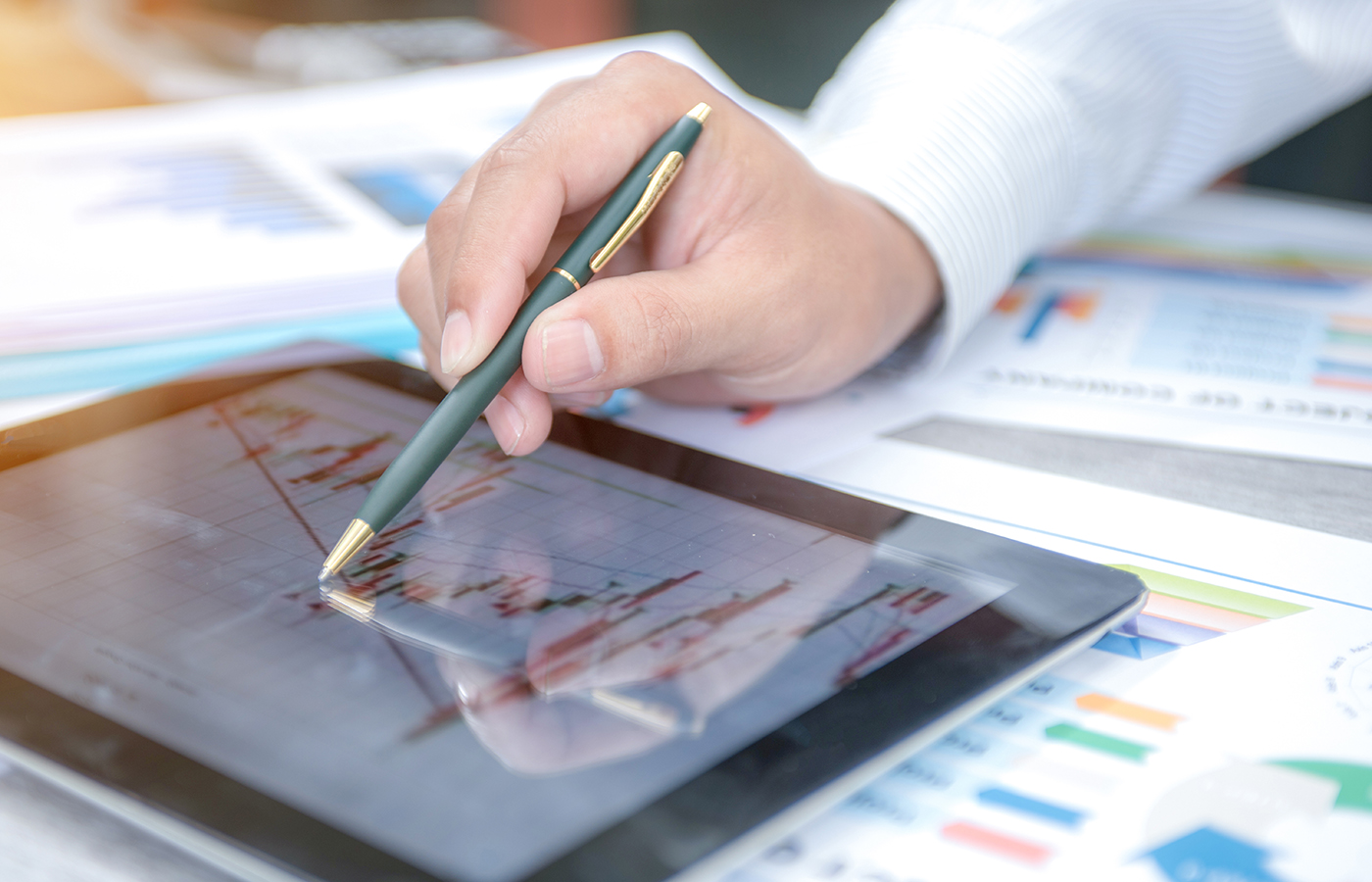
Contending with an unprecedented combination of shocks, financial risk modelers have lately had to muddle through some very tough times. Indeed, when you are relied upon to forecast the financial future, volatility and uncertainty – fueled by everything from a global pandemic to Russia’s invasion of Ukraine to high inflation and rising interest rates – doesn’t exactly help your cause.
Risk models, after all, primarily rely on historical data to make predictions, and now that information is colliding with data on current events that have little or no precedent. Complicating matters further for modelers are government-support programs, which were initially intended to mitigate default risk but may soon sunset as a result of tightening post-pandemic monetary policies.
The end result of these circumstances has been a failure of models, highlighted by credit risk methodologies that proved largely inaccurate in the early days of the COVID-19 crisis. However, for risk modelers, the unprecedented shocks have not only presented huge challenges but also demonstrated the value of modeling expertise – and that could be good news for aspiring and early-career FRMs who have an interest in modeling.
Evan Sekeris, Head of Non-Financial Risk, Americas, MUFG
Evan Sekeris, the Head of Non-Financial Risk, Americas, at MUFG, Japan’s largest bank, knows all about the current obstacles that models must overcome and the value that qualified, forward-thinking modelers bring to financial institutions. Currently, he is responsible for the risk management frameworks of MUFG’s various non-financial risks — including data, operational, model, third-party and business continuity. Throughout his career, moreover, he has worked with a multitude of models across institutions with varying goals.
Sekeris began his career in academia as a professor of economics at The Claremont Colleges before moving to the Federal Reserve Bank of Boston as a financial economist. Subsequently, he transitioned to the Federal Reserve Bank of Richmond, where he led the quantitative risk unit in bank supervision.
Recently, Sekeris spoke with Risk Intelligence (RI) about the challenge of building and evaluating financial models for rare and extreme tail-risk events; the skills that are now in demand for early-career risk practitioners and FRM candidates seeking jobs in risk modeling; and the reasons why model validation is a good place to start for aspirational risk managers.
RI: How do you anticipate some of the global shocks we’ve faced — including the pandemic, Russia's invasion of Ukraine, and elevated inflation — will impact the career path of beginning and future risk managers?
Evan Sekeris (ES): The lesson is that risk at financial institutions has gone from being all about market and credit risk to being increasingly focused on non-financial risks. These operational risks used to be an afterthought. Then the Basel Accords came along and now operational risk is a well-established discipline.
It's not just that the risk has been formalized, but also that the environment in which we operate has changed. Operational risks have become more prominent and, in some instances, become dominant risks. There are geopolitical risks and others — like inflation and the pandemic — that are big threats.
From a career perspective, I think future risk managers will have to be much better rounded than their predecessors. Credit risk and market risk are here to stay, but an understanding of operational risk is critical for anyone who aspires to a career in financial risk management.
RI: Since models are based on history, what does the unprecedented nature of some recent shocks say about the best way to oversee models?
ES: It’s true that models used in the financial industry are predominantly based on historical data. But they can be much broader. Think about climate risk, where there is no data because it hasn’t happened. We must forecast in the future.
Given our empirically-driven view, tail events and other shocks are difficult for models to handle. Under such circumstances, your data suddenly doesn’t behave as expected and your model’s forecast will likely be poor.
For example, when COVID-19 lockdowns hit, there was a spike in unemployment, which led models to anticipate soaring credit defaults. But government-support programs created a different outcome.
High unemployment coexisted with the lowest default rates we’ve ever had. The models didn't perform, but that doesn’t mean you throw them out. We still build models based on historical data, because it's very informative. Then we incorporate forward-looking behavioral assumptions.
However, if you introduce too many assumptions, some may view it as a deviation from the historical record and not supportable by data. But that's the whole point: if you anticipate the future won’t look exactly like the past, you must introduce assumptions that acknowledge there will be a deviation from the historical record.
We used to stay as close to the data as we could. That's a mindset change that's happening in the industry, but it's a painful transition, because how do you validate assumptions without data? You rely on people's beliefs about what the future holds or what future correlations will look like.
This makes for something that's less black and white when you validate. But it can’t be willy-nilly. You must incorporate expert judgment into your model in a formal and coherent way, and validate it without using a historical record. I think that's going to be the biggest challenge in model risk.
Think like a modeler. Don’t become a technician who builds models without considering the context of your work.
Some modelers don’t fully understand the assumptions their models are built on. That is a key differentiator between a competent modeler and a great one who has a promising future in a changing environment.
If you understand why the model is built the way it is, how the assumptions play into the model, and you’re not looking at it from a purely technical standpoint, then when events like the ones we've been seeing occur, you’ll be able to adjust the model to incorporate new facts.
RI: How can someone who wants to work with models, either building or validating, acquire the skills they'll need to do the job?
ES: Good quantitative skills are necessary. To be a modeler or a validator at an institution which has advanced teams of this type, a master's degree in a quantitative field seems to be where the line is drawn. Many people have PhDs.
After that, it becomes a matter of gaining experience on the job. There aren’t enough academic institutions that prepare people for the more intuitive side.
Find your first job at a financial institution and use that job to understand how modeling works there. It's important to leverage the first few years to build up your skills and knowledge. How do you bring in that intuition, that practical element? School is for the technical aspects, and the rest you learn on the job.
My first job in the industry was at the Fed. I came from a purely academic background, and the first year I basically spent learning how modeling works in practice. I had a very theoretical understanding. That first year was extremely formative, but you need that willingness to take a different view of modeling and you need to use your time on the job to change your perspective about how it works.
RI: How do you convince a hiring manager — whether it’s via skills, experience or attitude — that you're prepared to build or monitor models in this era of crisis and uncertainty?
ES: The most important thing a modeler needs to demonstrate to a bank is the ability to not always be overly theoretical and to accept that econometrics and modeling applied to the financial world is not as clean as it is in an academic setting. In academia you may focus on very specific assumptions and ensure near-perfection when you publish your work. But that's not possible at a financial institution, where you're trying to build models that are useful and practical.
This is the hardest thing for those of us who come from an academic background. Most quants have that academic mindset before moving to financial services.
At first, you might see your bank’s work as sloppy, and, from an academic standpoint, it might be. But it's practical. Try to demonstrate that you can be practical. This doesn't mean that you abandon the rigor of modeling, but that you’re flexible enough to optimally marry that rigor with the realities of a business environment. I think that’s the key.
Candidates absolutely need the core quant skills to make it to the final rounds of interviews. The differentiating factor is good intuition and practicality, which is the ability to take your quant skills and apply them in the real-world. If you have the desire to understand the business and the environment in which the institution operates, you can translate that into useful modeling.
RI: You mentioned in a recent GARP webcast that it's difficult to hire qualified people to work as model validators. Might this be a good niche for early career or aspiring risk managers? And if so, how would they prepare themselves for this role?
ES: I think it's a great entry point, because it gives you the ability to see a wide range of models. Developing a model is a full-time endeavor. You’ll be assigned to one model and that's all you're going to do for a year or two. But validation of a model is much quicker.
As a validator, you’ll have the opportunity to see a broad swath of models. It's a great learning experience, as you go through these different models to see what criteria are applied from a validation standpoint. It helps you understand which elements of a model you don’t have to emphasize as much and which parts are material.
Model validation is especially useful if you don't know the financial industry well. You’ll see so many models, which can also help you figure out what type of modeling you like. Are you more into, for example, market risk or credit risk or marketing models?
I do think that if you start as a validator, you should make it a point early on to move to the modeling side and build models. Being on the receiving end of the validation is very important for understanding the challenges modelers face when they interact with validation. You can always move back to validation later if you prefer it.
Tod Ginnis is a content specialist at GARP. He is the author of a GARP blog that is aimed at early-career risk managers and professionals aspiring to earn their Financial Risk Manager (FRM) certification.
Topics: Model Risk