Although Interest Rate Risk in the Banking Book (IRRBB) is not a new topic, there are very few discussions and publications about the technical challenges that many banks still face.
Regulators have set clear expectations for the IRRBB technology stack. The corresponding regulations (e.g., BCBS 368 of 2016 and European Banking Authority 2018 guidelines) contain high-level principles for IT architectures and data management, which banks and their software providers must meet.
Any IRRBB solution needs to facilitate banks' interest rate risk measurement in terms of Economic Value of Equity (EVE) and Net Interest Income (NII). Whereas EVE quantifies a position's sensitivity to pre-defined interest rate shocks in terms of present value, NII focuses on the Earnings-at-Risk given certain interest rate risk shock scenarios. Due to various methodological degrees of freedom paired with data and performance-intensive calculations, a well-designed technological setup is pivotal.
This article elaborates on the necessity of establishing a flexible and consistent IRRBB architecture that excels through advanced performance and data management capabilities.
Flexibility of the Architecture
No two banks are alike. Since IRRBB touches upon the very core of the business, great flexibility is needed to cater for differences in contract specifications and business models. Even for small and medium-sized banks, projecting cash flows into the future under multiple scenarios can be time-consuming and require disk and memory space.
A well-designed and powerful IRRBB solution needs to reflect the bank's internal view on interest rate risk exposure while sticking to the regulatory (reporting) guidelines. Furthermore, it should help banks keep up with the fast-changing regulatory rulebook using a reasonable number of resources. Banks' system landscapes are constantly updated, and outdated technological infrastructure components are common. Hence, IRRBB vendor solutions typically need to support several client configurations, e.g., regarding SQL servers.
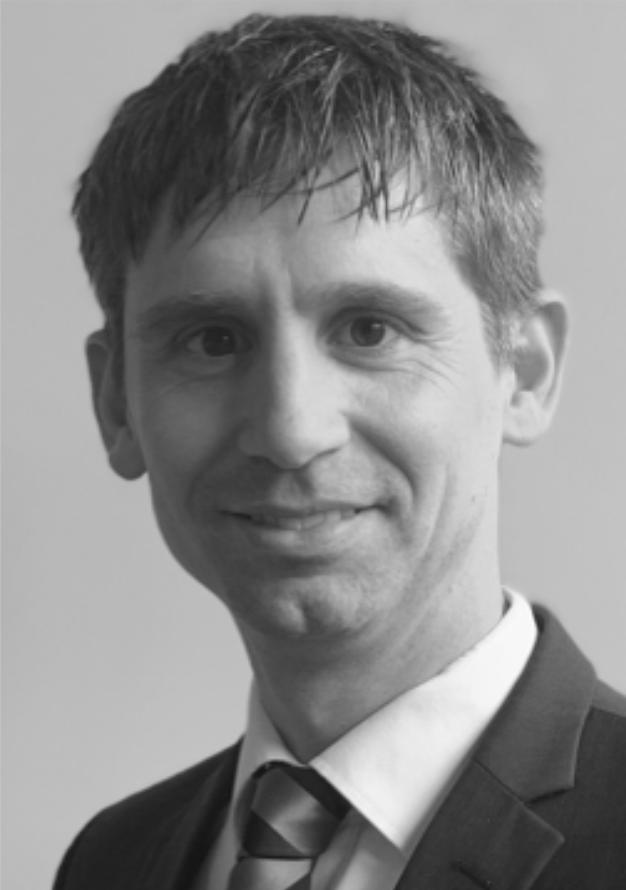
Functional requirements of several business departments (both front- and back-office units) must be met simultaneously. For example, IRRBB requires the adequate consideration of embedded behavioral optionalities (e.g., prepayment optionalities, drawing of credit commitments, computation of hypothesized withdrawals of non-maturity deposits). Furthermore, the models are dynamic over time and must be continuously updated and validated.
Hence, the vision of a loosely coupled enterprise environment to foster flexibility was regarded as the Holy Grail for some years. Service Oriented Architectures (SOA) were considered a solid technological answer in that respect, but the services could not be deployed fully autonomously.
Meanwhile the implementation of completely de-coupled units like microservices appears to be best for creating and maintaining a complex risk solution landscape supporting agility by automating testing and deployments (via so-called CI/CD pipelines). Changes to small containerized microservices can be made more quickly without putting the rest of the system at risk. In the IRRBB context, the rate shock scenario generation and the cash flow projections represent perfectly re-useable microservices. However, microservices architectures also inhibit challenges for orchestration, resiliency, communication and the organizational setup.
Consistency of the Architecture
Stimulated by the BCBS 239 principles for effective risk data aggregation and reporting, published in 2013, consistency has become an essential feature of any bank's system architecture. Each data point requires a single point of truth. The IRRBB requirements further emphasize the need for holistic and redundancy-free architectures enabling consistent EVE and NII calculations.
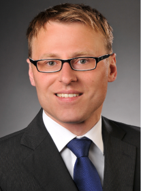
Additionally, the IRRBB processes must be aligned with other risk or capital management processes (e.g., capital planning, Value-at-Risk measurement, funds transfer pricing, liquidity risk). IRRBB spread risks, for example, must be incorporated in the Value-at-Risk calculation for market risk. The IRRBB modeling approach to non-maturity deposits must be considerate of liquidity risk models. Again, microservices and APIs (application programming interfaces) facilitate the need for holistic bank management in that regard.
The move towards an integrated platform that uses a de-coupled and containerized architecture allows banks and software providers to establish central teams for specialized analytical resources. In turn, these shared services across the organization function as a method factory servicing the business. Methods used in batch processes are called through the same API. As these shared services (e.g., for pre-payment calculations in IRRBB) are owned by central teams, business units have access to the most current version.
Performance and Data Management
The BCBS 239 principles also articulate clear expectations for consolidation, drill-down and forecasting capabilities. Further key functionalities required for scenario analysis and stress testing are timeliness, completeness, adaptability and accuracy. Hence, banks need to be able to increase the frequency at which IRRBB is monitored.
Traditionally, most institutions assess monthly data points - in part because of challenges in terms of calculation runtimes and data collection. Increasing the frequency and simultaneously covering multiple scenarios, a bank with, say, 1 million deals could easily end up processing more than 500 million IRRBB cashflows. Hence, banks must carefully consider performance implications.
The BCG Global Risk 2020 report mentions outdated IT architectures, insufficient data management and low performance as one of the main reasons for inadequate IRRBB management. Smart data management, and in-memory processing boosted by distributed processing or grid computing, provide ways to tackle these challenges. Intelligent data management, in combination with in-memory analytics, even allows for intra-day and real-time risk and scenario calculations, including the latest transaction updates or simulated scenario changes. New technology stacks such as SAP HANA or big data applications like Spark have introduced new possibilities for financial risk management including IRRBB.
Scalability and Cloud
Distributed computing allows for vertical (scale up) and horizontal (scale out) scalability. Financial institutions can add more power to their existing machines (up) or by adding new machines to the system. Here the need for another technology has arisen: Cloud computing and its auto-scaling capabilities become a more and more valid option for banks which historically maintained their IT using a multitude of internal system administrators. When banks start heavy simulations like IRRBB, cloud providers can provide more computing power on demand.
Data lineage is another important aspect of a state-of-the-art IRRBB solution. The solution must offer a drill-down of IRRBB result data down to the single transaction or even cash flow level. Risk managers can thereby better identify the drivers of EVE changes navigating through intermediate data splits such as “EVE by currency” or “NII per time slice.” For bank holdings that are forced to run complex consolidation steps in their reporting, this is at times a time-consuming task which lacks automation.
Summing up, technology is the great enabler. It has driven financial risk management in banks to a new level, also partially driven by increased regulatory demands. High-performance computations facilitate a comprehensive analysis of interest rate risks in terms of EVE and NII covering multiple time spans and scenarios, enabling better informed management decisions.
However, the heavy use of complex technology also bears risks. Little wonder that, among others, IT resiliency and continuity risk will play an increasingly important role for regulators and bank boards of directors alike.
Stefan Trummer (stefan.trummer@bearingpoint.com) is senior product manager for Reg and RiskTech software solutions at BearingPoint. He has more than 15 years of experience in regulatory compliance and risk management in various roles as consultant, subject matter expert and in software development.
Dr. Patrick Hauf (Patrick.Hauf@zhaw.ch) has been working for the Swiss entity of BearingPoint RegTech leading the IRRBB team in his role as product advisor. He recently transferred to ZHAW School of Management and Law, one of Switzerland's leading business schools, to bring in his experience in risk and asset management. He also teaches risk management at the University of Konstanz, Germany.