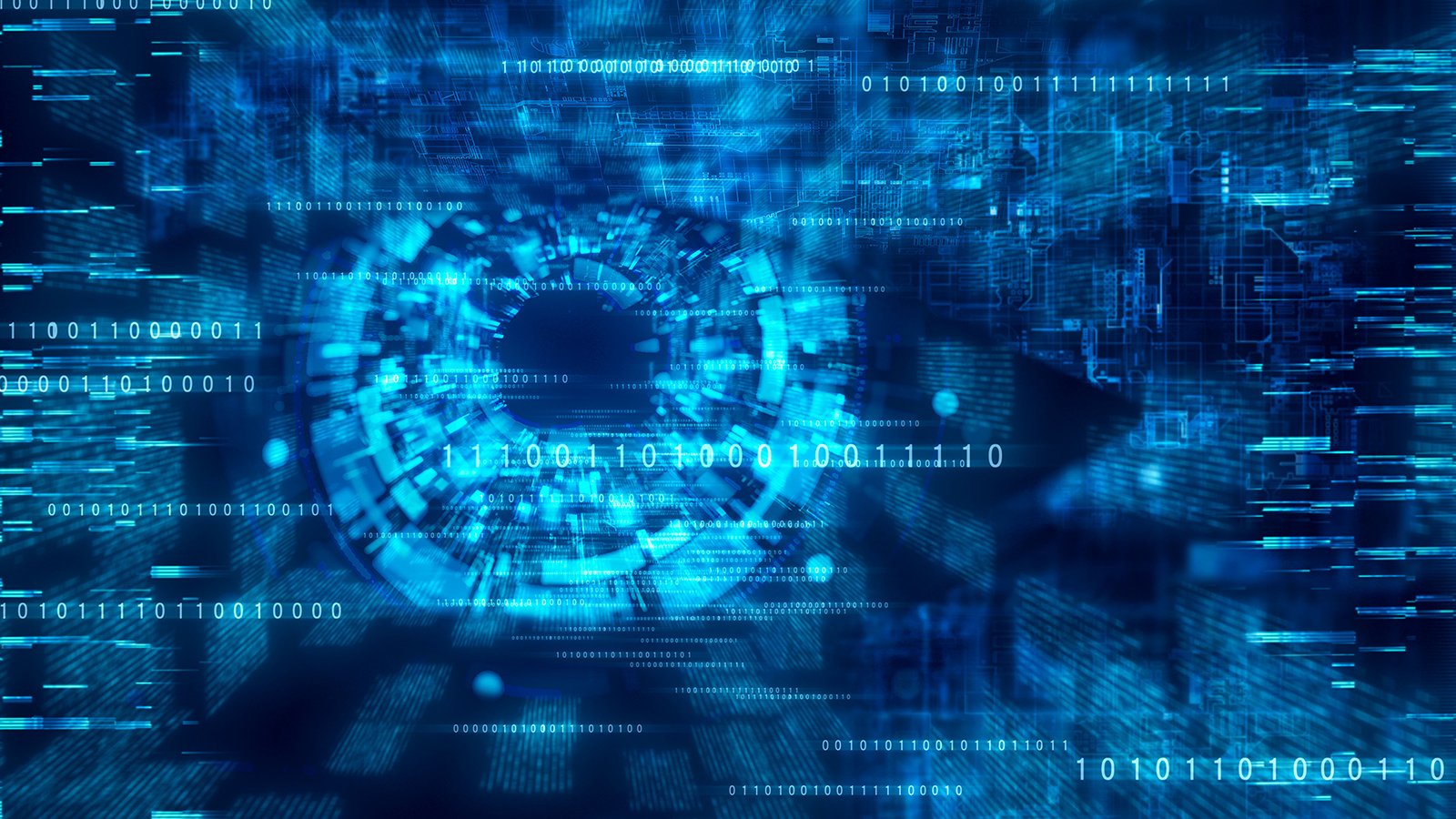
The rise of artificial intelligence tools over the past few years has improved modeling performance and has yielded risk management benefits across areas like fraud protection, anti-money laundering and credit underwriting. But it has also created concern about the dangers that they could pose to the financial system, society and even to the existence of humanity itself.
Many safeguards have been proposed to limit the potential negative consequences of AI, but as this disruptive technology becomes more advanced, its risks are also growing in complexity.
Jesús M. Gonzalez
Think, for example, about the susceptibility of generative AI tools to so-called hallucinations – errors that occur when you use unclear prompts or feed the system wrong information or ask it follow-up questions that require deductive reasoning. But one can also argue that its potential benefits right now outweigh its risks, as generative AI can, for example, potentially improve firms' ability to identify and rank threats and to communicate risks.
The same reasoning can be applied to AI as a whole, so it’s safe to say the technology is not going away any time soon. There is, however, one approach that can be applied to reduce its risk: harm reduction.
Learning From the Public Health Sector
Harm reduction is a public health approach and social philosophy that aims to reduce the negative consequences or harms associated with various risky activities or behaviors (e.g., substance abuse, sexual practices, smoking, gambling). Its core tenet is to prioritize the well-being and safety of individuals, even if they are engaged in behaviors that could be risky.
Laura M. Gonzalez
Through its evidence-based approach, harm reduction has shown to be effective in reducing consequences of health-related decisions. Although it sometimes incorporates legislation, harm reduction is different from a regulatory or legalistic approach in that it originates from a grassroots community-member perspective.
Harm reduction acknowledges that complete abstinence from certain risky behaviors might not be achievable or realistic for everyone. Instead of focusing solely on stopping these behaviors, harm reduction seeks to minimize the costs that can arise from them. It seeks to better understand the motivations of individuals to engage in potentially harmful behavior, educating and channeling them toward less harmful choices in their day-to-day lives, away from the regulatory eye.
Applying Harm Reduction Strategies to AI
The polar extremes of shutting AI down completely or letting AI grow unchecked are not realistic. But a harm reduction approach would at least minimize risks – to both the financial services community and to society.
Instead of eliminating the harms that AI might cause, this approach would focus on reducing negative consequences associated with AI technologies. The goal would be to ensure that AI is as safe, secure, transparent, ethical and responsible as possible.
A harm reduction approach would encourage AI developers to design and build systems with a focus on preventing unintended harm, such as bias, discrimination or unfair outcomes. It would also emphasize the development of safeguards that mitigate the risk of systems being hacked or misused.
Protecting the privacy and security of individuals’ data would be yet another item on the harm reduction checklist for AI. The idea would be to give individuals the ability to choose how their data is used by AI systems. On the financial services side, this would call for firms to adopt strong data protection practices and to adhere to relevant regulations.
There are three additional harm reduction pillars that can be applied to AI:
Educate and Inform
Provide accurate information to users, developers, policymakers and the general public about AI’s capabilities, limitations, risks and potential harms, and encourage them to use it in a safe and responsible way. This will empower all parties involved to make informed decisions about their actions related to AI use.
Community Engagement
Invite a diverse range of stakeholders, including ethicists, researchers, policy makers and affected communities, to develop and implement AI harm reduction programs in the financial sector. Multiple perspectives will help mitigate risk by framing solutions in broader terms (not just financial gain or loss) and by identifying novel cross-sector solutions.
Regulation and Policy Advocacy
Rather than taking punitive measures, such as criminalization, regulators should advocate for AI rules and policy changes that prioritize safety, fairness, accountability and well-being for the public and individuals. Newly developed regulations should balance the benefits of innovation with the potential harms associated with AI technologies, seeking solutions that are responsive to many stakeholders.
Parting Thoughts
This article provides a starting point for considering how harm reduction strategies could help risk managers find a middle ground in the AI governance debate. Harm reduction strategies are most robust when they are multi-pronged and framed in a way that is “local” to a particular issue.
If you have a leadership role in how AI might be implemented at your firm, consider different ways that you could change the mindsets of AI stakeholders, including developers and individual users. Give them information they could use to protect both their firms and the public.
Jesús M. Gonzalez (FRM) is a senior quantitative risk professional with over 20 years of experience in large North American and global financial institutions. He specializes in the building and validation of market risk quantitative processes, model risk management and governance of artificial intelligence and machine-learning technologies.
Laura M. Gonzalez (PhD) is an educational consultant, researcher, and author. She worked in higher education for over 20 years, and has published two books, 15 book chapters, and more than 60 scholarly journal articles.
Topics: Responsible & Ethical