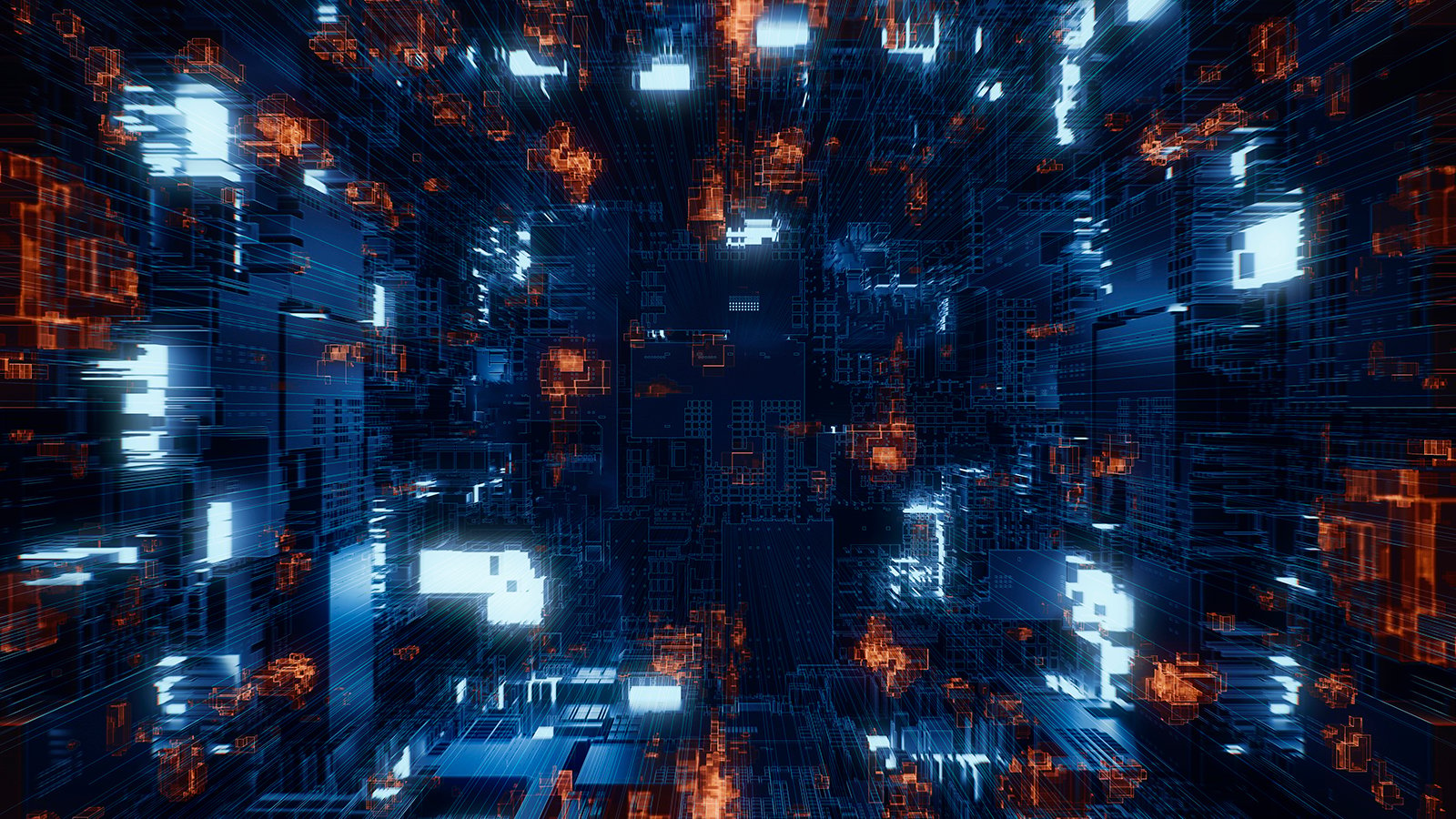
Generative AI has been a smash hit with consumers. Earlier this year, for example, OpenAI’s ChatGPT chatbot set a record by racking up 100 million users within two months of its launch. But does this disruptive technology offer any practical value to credit risk modelers?
ChatGPT is the most visible application that belongs to the broader domain of “Large Language Models.” It is an example application of generative AI: artificial intelligence that is used to generate new output based on brief user instructions called “prompts” – a process also known as prompt engineering. You can submit questions to ChatGPT on almost every topic and it will deliver answers in a format of your liking.
Earlier this year, I attended a credit risk conference that featured a ChatGPT-generated speech from the moderator. The utterly convincing speech highlighted some recent credit risk trends.
To find out more about how much ChatGPT understands about credit risk, I engaged in a session with the chatbot and asked it how generative AI could improve credit risk modeling. ChatGPT identified a total of 18(!) areas in which generative AI could prove useful to modelers. These can be condensed to the following four key benefits:
- Enhanced PD, LGD and EAD modeling, through models that are better calibrated.
- Superior stress testing, via the generation of wide-ranging, adverse scenarios.
- Improved model validation (with the help of synthetic data), reduced modeling bias and better explainability.
- More balanced datasets (better default/non-default proportions) and improved data quality and outlier detection.
Coding Enhancements: A Game Changer?
In the short run, the one area in which generative AI has the potential to fundamentally change the way credit risk modelers work is in the coding of PD, LGD and EAD models.
One can, for example, ask ChatGPT for approaches to known modeling dilemmas – e.g., how to convert a through-the-cycle (TTC) PD to a point-in-time (PIT) PD. When I asked this question during my session with ChatGPT, it did not spontaneously recommend the Vasicek formula; after this was suggested, however, it confirmed the validity of the idea and provided the specification of the Vasicek formula.
Moreover, when asked to write the related code (converting TTC PD to PIT PD, via the Vasicek formula) in the R programming language, ChatGPT spit out the following snippet:
Figure 1: Code Snippet for Vasicek Formula Generated by ChatGPT
ChatGPT expressed the required formula in a function, which is a good idea. It also added a lot of comments, improving the understandability of the code. What’s more, it explained the application of the function, with an example.
Marco Folpmers
The coding enhancements ChatGPT deliver could be a game-changer. Indeed, going forward, credit risk model developers may very well use two screens: one with their standard coding environment, the other with ChatGPT (or similar) support.
Potentially, generative AI could have a larger impact on credit risk modeling than even the surge in machine-learning (ML) models.
Caveat: The Hallucinations Dilemma
For all its potential benefits, ChatGPT is far from perfect. The system gets derailed from time to time, producing nonsense that is embedded within otherwise sensible paragraphs. This phenomenon is called “hallucinating,” and is dangerous because user expertise is needed to separate the wheat from the chaff.
The conditions under which this problem occurs remain a bit of a mystery, partly because generative AI is still in its infancy. However, it seems like hallucinations are most likely to happen if you feed ChatGPT wrong information, refer to events after the cut-off date for its knowledge repositories (September 2021, as of this writing), or use deliberately unclear prompts.
It’s also likely that if you ask ChatGPT follow-up questions that require deductive reasoning, you’ll run into a hallucination sooner than when you ask queries that require encyclopedic knowledge and look-up functions.
As I found out when grilling the system about a linguistics matter, it can produce false answers with amazing self-confidence. On the positive side, though, when confronted with such errors, ChatGPT apologizes and concedes its mistakes.
Clearly, ChatGPT does not pass the Turing test, but it wasn’t meant to do that. It is a human-computer interface that leverages data in innovative ways, generating, along the way, new and creative solutions to difficult problems.
Parting Thoughts
Credit risk modelers would be well advised to examine carefully the pros and cons of generative AI systems like ChatGPT.
The strongest feature of this disruptive technology is its versatility. One minute, it can answer general questions on methodology, and in the next it can provide guidance for concrete approaches – including specific formulas and step-by-step implementation instructions to credit risk modeling challenges. Moreover, it can do all of this in one’s preferred programming language.
Generative AI is certainly not flawless. It can be duped if you use unclear prompts or feed the system wrong information. However, it also provides strong benefits to credit risk modelers, including coding enhancements that improve PD models.
Consequently, I expect generative AI systems like ChatGPT to be quickly and eagerly adopted in the FRM community.
Dr. Marco Folpmers (FRM) is a partner for Financial Risk Management at Deloitte the Netherlands.
Article Updated: March 26, 2024
Topics: Modeling