When banks manage risk, conservatism is a virtue. We, as citizens, want banks to hold slightly more capital than strictly necessary and to make, at the margin, more provisions for potential loan losses. Moreover, we want them to be generally cautious in their underwriting.
But what is the best way to arrive at these conservative calculations?
There are really only two choices. First, the senior managers at a financial institution could instruct their analysts to produce models that yield conservative forecasts. By “conservative forecast,” I mean one that deliberately overstates potential credit losses or that deliberately understates potential revenue. Alternatively, bank executives could seek a “balanced” or “accurate” prediction from their analysts and then use subjective management overlays to render the resulting numbers suitably conservative.
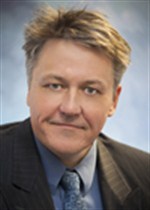
The second of these options should always be preferred.
The problem with asking an experienced statistician to produce a conservative forecast is that they will invariably succeed. In the context of probability-of-default modelling, for instance, you may have a particular number in mind that you want to hit - like 10% peak losses under stress. If there's a million possible models and a thousand that will pass validation, you can usually find ten that will get you pretty close to the target. At the end of the day, of course, you only need one.
This is not proper analysis. That said, it does take exceptional skill to be able to maintain the perceived validity of the process while, in fact, the numbers are all pre-determined. But make no mistake about it: If you set out to produce a 10% loss forecast and your model achieves that outcome, the estimate is not actually a statistical construct.
Finding the Right Model
Conversely, the analysts could just be asked to build their very best models.
For problems akin to baseline forecasting or credit scoring, this instruction is relatively easy to convey. In credit scoring, for instance, the task facing the modeler is to maximize the separation between good and bad accounts in terms of their calculated score - i.e., to maximize the KS statistic or something similar. In baseline forecasting, the task is to minimize out-of-sample forecast error.
It's easy to calculate statistics that allow these abilities to be measured, and thus to see which model has historically performed best.
In scenario analysis, however, you are estimating what will happen conditional on events unfolding in precisely the stated manner. Given that the scenario has never happened and will never happen, the model can never be truly invalidated. It thus becomes a partly subjective exercise to decide whether a particular model is doing a good job or not.
This subjectivity opens the door to conservative forecasting. Most modelers will compare their stressed projections to portfolio performance during the Great Recession and decide, on that basis, whether the model is fit for purpose.
We saw an interesting manifestation of this recently. A mortgage portfolio scenario was presented by us that showed future stressed losses running about 60% of the actual levels observed during the 2008/09 recession. We pointed out that the new scenario is arguably less severe than that event - with peak unemployment rising to 8% (instead of 10%) and house prices falling by substantially less. We also demonstrated that underwriting standards are now far stricter; borrowers today have more skin in the game and far higher average and minimum credit scores than their 2007 forebears.
Nonetheless, the model user was adamant that the final numbers be more severe than in the Great Recession - a conservative forecast if ever there was one.
In the context of, say, a stress test, use of such a forecast means that senior management will never get to see the best possible, unbiased view of their financial outlook. In the context of CECL and IFRS 9, meanwhile, it means that a purely arbitrary component will appear in the company's financial statements - seemingly produced by a statistical model, but which actually sprung to life in the mind of a manager.
If the bank in question chooses to capitalize on the basis of a repeat of the Great Recession, then so be it. As a citizen, I'd be satisfied by this outcome. However, the bank's managers and investors should know how much of the capital is indicated as necessary by a scientific investigation of the portfolio and how much is pure subjective cushion to cover things like model risk and other uncertainties that not are captured by the data. One would think that regulators would also benefit from banks producing analysis that is capable of drawing this distinction.
Parting Thoughts
Having worked with many institutions with a range of stripes and hues, I'd argue that conservative modeling is prevalent in the risk management industry. Given that the aim of regulators is to push banks in the direction of increased safety, it must be tempting for them to prefer models with an obvious conservative bent.
The only downside is that, in doing so, they effectively expunge the practice of statistical science from the risk management process. As a citizen-statistician, I want the best of both worlds: accurate, informative statistical models coupled with explicit, subjective and conservative management overlays.
Tony Hughes is a managing director of economic research and credit analytics at Moody's Analytics. His work over the past 15 years has spanned the world of financial risk modeling, from corporate and retail exposures to deposits and revenues. He has also engaged in forecasting of asset prices and general macroeconomic analysis. Please click here if you'd like to read other recent articles Tony has written as part of his “Modeling Risk” column.
Article Updated: April 8, 2024