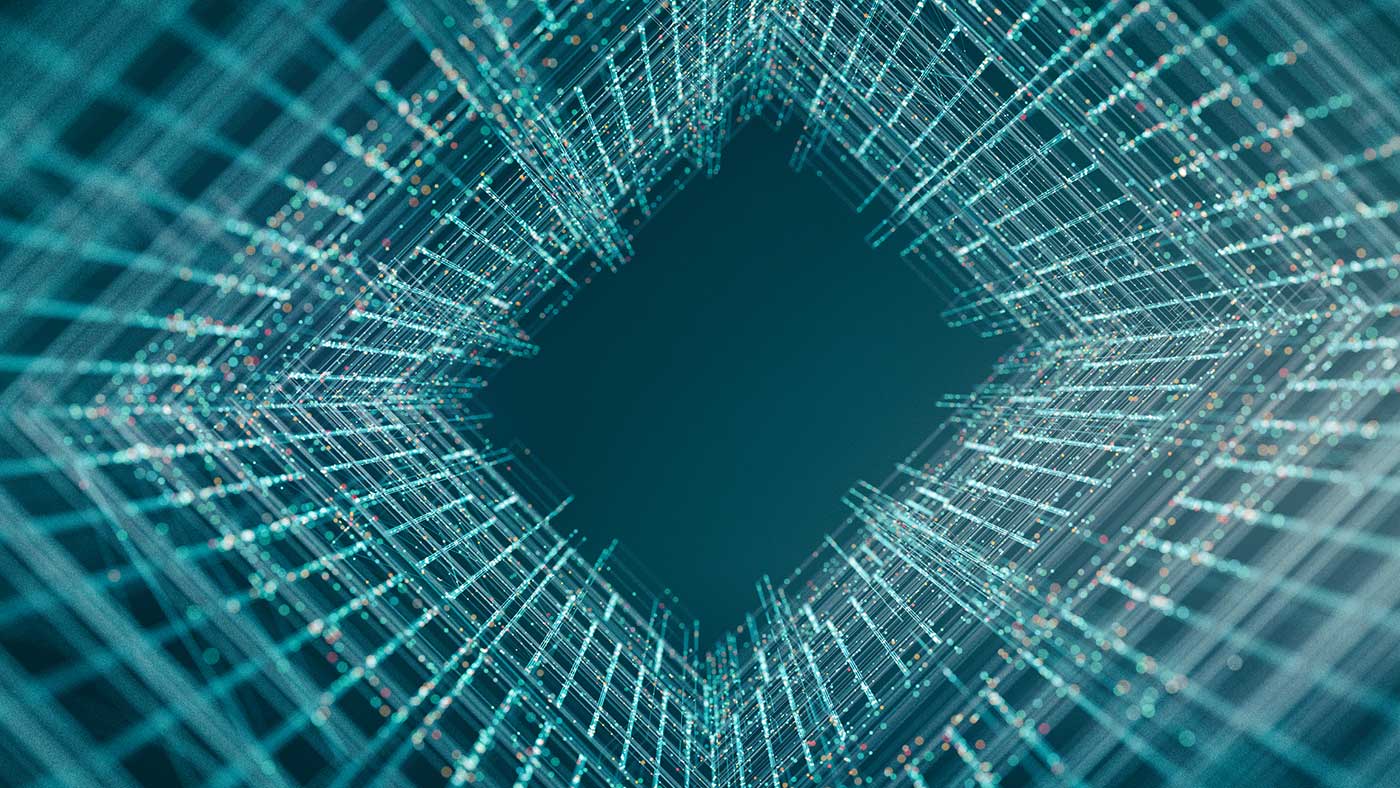
Many organizations now have a chief data officer or “data czar(ina).” Organizations increasingly want to be data driven, and change company culture to value data in the same way they would, say, strategy or stock price.
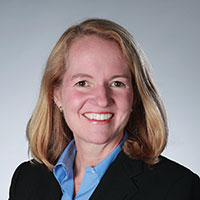
What's more, even if your organization does not have an enterprise data architecture, risk management depends critically on the relevance and integrity of its data, and the data governance that creates clear roles and responsibilities for data ownership.
Previously, we covered the business architecture of IRM, or the risk processes that support a bottoms-up risk management process to engage business owners in risk identification, control assessment and action planning. Now, let's suppose your company has put these bottoms-up risk assessment processes in place, and would like to develop a central data architecture. Simultaneously, let's also assume your organization values data integrity, and would like to avoid spreadsheets and a proliferation of user-designed tools.
The goal should be to efficiently compile and aggregate this data collected across the organization (see Figure 1), as a precursor to a project to automate the risk management process, workflows and reporting.
Figure 1: Implementing Integrated ERM
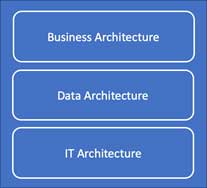
Integrated risk management (IRM) is akin to customer relationship management (CRM) in the 1980s. Before systems and processes were introduced to manage client information and relationships, data were scattered in silos, resulting in inconsistent and redundant information with no aggregate view of the client and its importance to the business. CRM systems entered the picture to create a single view of customer information and interactions across business processes and roles.
IRM systems and processes aim to achieve the same thing - to provide an integrated picture of risk information and processes across the business and support units. To accomplish IRM requires the establishment of a business process and risk data architecture.
Performance objectives, risks, controls, events, obligations, physical and information assets, third parties, policies and objectives are all related. Organizations should be clear about how business performance objectives relate to risk appetite; how risks relate to controls; what events happened that exceeded risk appetite thresholds or violated specific policies or a regulatory requirement; what controls are established in specific policies; which third-parties support assets critical to a business process; and whether tested controls are within acceptable tolerance levels of residual risk.
Benefits of an Integrated Approach
An IRM data architecture integrates three layers of information, from sources both within and external to the organization.
Figure 2: Three-Layer IRM Data Architecture
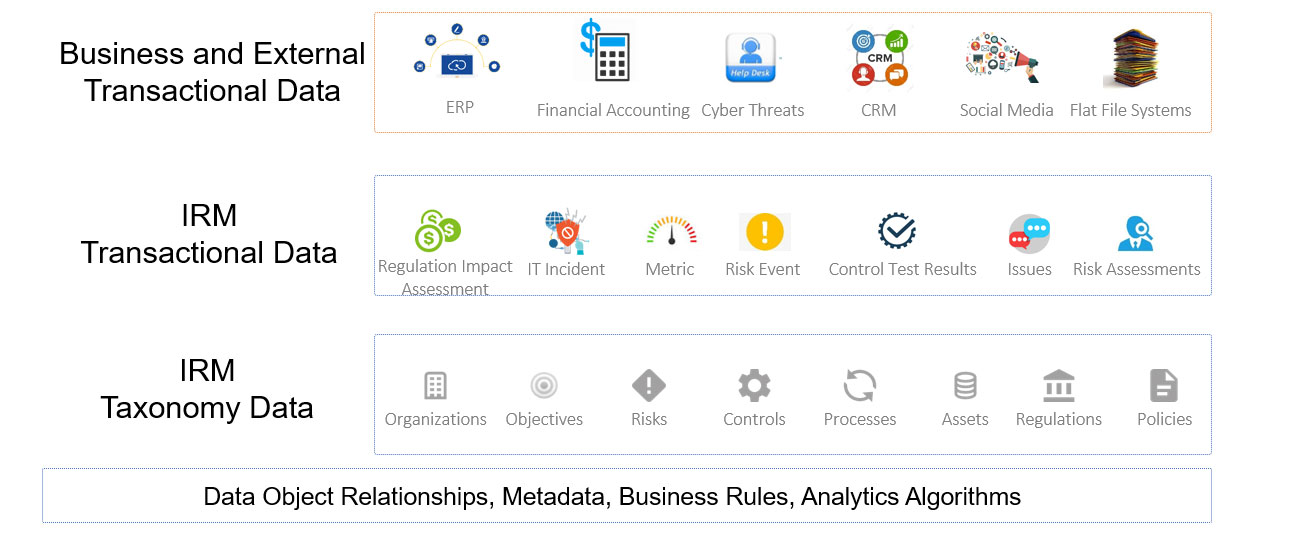
Business and external transactional data are the external data feeds that will be fed into your risk systems to help automate some decision rules. For example, business transaction data can include the vendor spend and organizational structure data that may come from an organization's financial accounting system. External feeds could also contain cyber threat intelligence, vendor credit ratings and negative sentiment comments detected from social media sources.
IRM transactional data is the output of your risk management processes, including (1) business-impact assessments, risk events, regulation or legislation-impact assessments; (2) control test results and risk assessments of business process, vendors and compliance obligations; and (3) IT and information assets.
It will also include metrics from the external data feeds that will trigger risk activities. For example, a large change in a financial account level may “trigger” a SOX financial control assessment. Or a large number of employees that fail to meet internal training requirements may trigger a risk assessment.
The IRM taxonomy is the standard language of our enterprise risk management processes. It allows an organization to capture and contextualize the risks.
To understand where a risk is happening, let's call it a “risk node” and tag it to organization, product and process. This node may be where you wish to perform a risk assessment or where a third-party vendor impacts your organization's ability to deliver. It can also be a point-of-control failure.
The node contextualizes the risk information that will be created. Take a look, for instance, at the following example from the energy industry:
Figure 3: Risk Data Contextualization
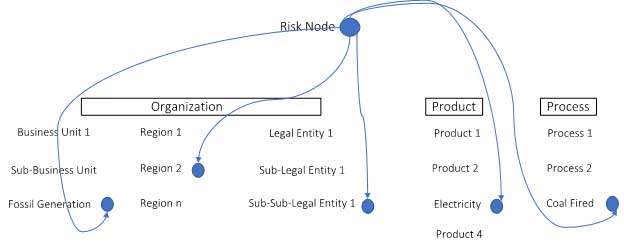
There is, actually, more to this taxonomy. Across the areas of IRM - including risk management, compliance, third-party management, internal audit, business continuity management and IT/Cyber - a common language should be used to help report and analyze the data.
Figure 4: Common Taxonomy of IRM
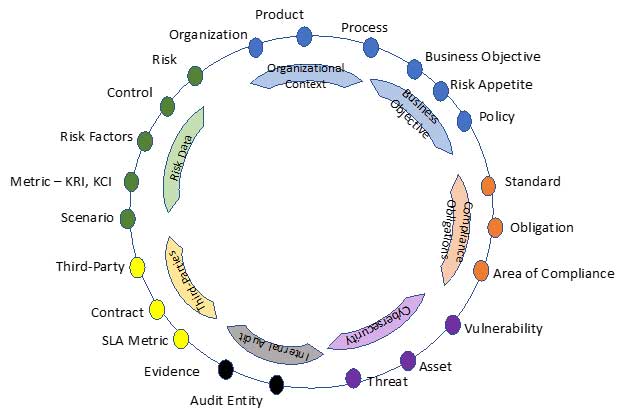
Data-Object Relationships
Each risk can be linked to multiple other data objects. For any data object, the relationships may be one-to-one, one-to-many, many-to-one or many-to-many.
Figure 5: IRM Risk-Data-Object Relationships
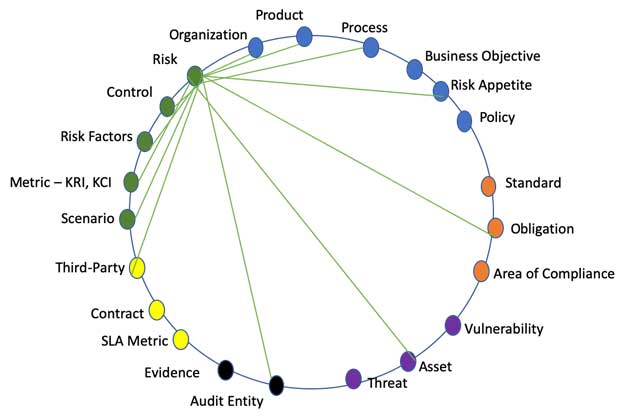
The illustration above is simplistic, as each library object can be connected to multiple “levels.” For example, a level 2 risk (see Figure 6) could be linked to a level 3 control.
Figure 6: Illustrative, Multi-Level Risk and Control Library Object Approach
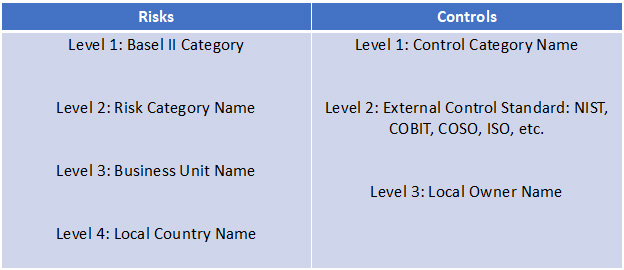
The board may understand the level 2 risk names and level 1 control names, while the business may have its reports created using level 4 risk names and level 3 control names.
Metadata
Metadata is a set of data that describes and gives information about other data. It's easiest to show this with some illustrative examples. The rule for metadata collection is collect only what you may need for reporting and analytics.
Figure 7: A Metadata Example
Data Model Attribute |
Metadata (illustrative) |
---|---|
Risk (level 4) |
Risk Owner, Risk Description, Comments, Internal/External |
Control (level 3) |
Control Owner, Control Description, Type, Embedded (Y/N), SOX (Y/N), Primary/Secondary, Automated/Manual, Preventative/Detective, External Third-Party Reliance (Y/N). If so, which Third Party (pick Third-Party library object), Supporting Documentation attachment, Training (Y/N) |
Process |
Process Owner, Process Description |
Let's consider an example involving controls to understand the importance of metadata. Identifying controls across the business, IT and SOX is tedious and painful work, often taking years. The question is, why bother? Well, after the data is compiled, interesting analysis can be performed to determine whether orphan controls are related to a firm's risk, compliance obligations or policies.
The metadata, moreover, can be leveraged to answer the following important questions: Can these orphan controls be stopped? Why are multiple groups testing a control that is related to a “low-rated” risk? If we have a primary and secondary control linked to a risk or compliance obligation, can we discontinue other secondary controls? If most controls are manual, is there a business case for automating the controls? And if many controls are detective, should we redesign the control to be preventative?
Based on my experience, there are very large opportunities to optimize an organization's control environment, typically leading to very large cost savings.
But please do not think this is simple. Metadata management can be tricky and political. For example, when evaluating the data, one must consider whether the risk owner in the first or second line of defense. Roles and responsibilities for risk data management will evolve in every organization, but great clarity will be driven by the need to establish a risk data architecture.
Business Rules
Business rules help make your IRM approach smarter and more risk based. They are the logic behind how data will be consistently used. For example, rather than schedule risk assessments every six months, why not trigger the risk assessment of a business process based on a series of control test failures, a certain percentage of employees failing to complete training, a change in vendor, a cyber risk event or the introduction of a new product?
Another example of a business rule is to automate the calculation of a risk based on the relationship between the risk and metrics thresholds. If negative news media and negative social media sentiment is rising, should the risk level be increased, and perhaps trigger an action plan to mitigate, accept or transfer the risk?
Artificial Intelligence, and Other Analytics Algorithms
Artificial intelligence (AI) has the potential to improve data management significantly. AI could be used for such IRM use cases as mapping risk assessments and risk events to appropriate vendors, assets or processes; automating data-object relationship mapping; deriving business rules to trigger risk assessments and control testing; and performing continuous control monitoring. (These and other IRM AI use cases could be the topic of another article.)
Parting Thoughts: Readiness for Workflow and Automation
The perception of an organization's integrated ERM program and people depends largely on it's the effectiveness of its risk data architecture.
Poor data quality can, of course, undermine a risk management program. Three approaches are often used to ensure data quality. First, each type of risk data must have an owner, and when the owner completes his or her work, the data should be reviewed and approved. Second, text input - and, more specifically, relying on drop-down menus for user selection of approved responses - should be minimized. Third, data problems can be flagged using post-data input, automated checks and business rules.
Conceivably, all of the layers in a risk data architecture could be managed manually, but this information becomes hazardous and inefficient in a two-dimensional spreadsheet. Therefore, in a future article, we'll be addressing the development of an IRM IT platform architecture -knowledge that could prove quite useful for those looking to bring efficiency to risk management processes, data management, reporting and analytics.
Before pushing forward with automation, though, each firm must first gain agreement on the best approaches for managing risk processes and risk data.
Brenda Boultwood is a Risk Advisory Partner at Deloitte. She is the former senior vice president and chief risk officer at Constellation Energy, and has served as a board member at both the Committee of Chief Risk Officers (CCRO) and GARP. Prior to joining Deloitte, she was a senior vice president of industry solutions at MetricStream, where she was responsible for a portfolio of key industry verticals, including energy and utilities, federal agencies, strategic banking and financial services. Before that, she worked as the global head of strategy, Alternative Investment Services, at J.P. Morgan Chase, where she developed the strategy for the company's hedge fund services, private equity fund services, leveraged loan services and global derivative services.