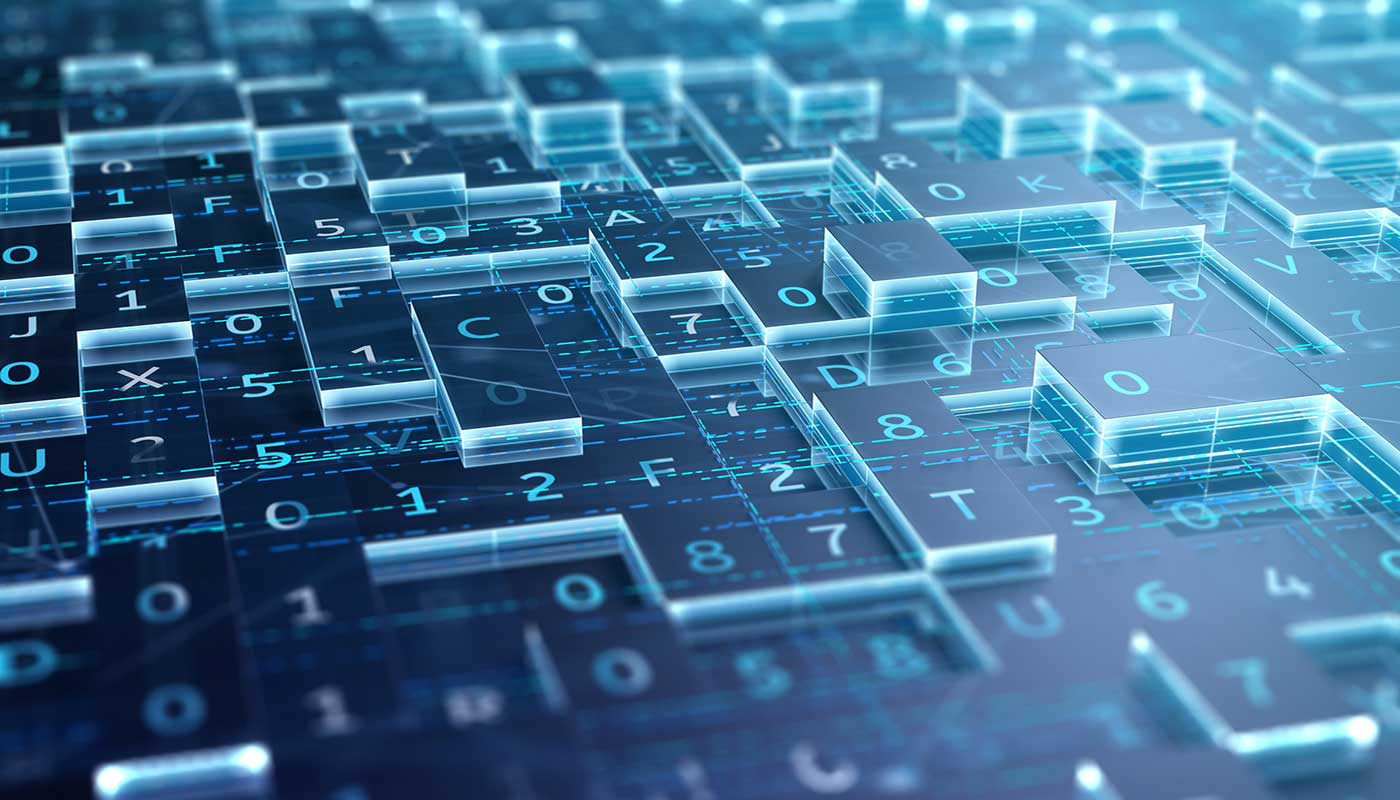
COVID-19 has had an unprecedented impact on the economy, society and financial institutions. While the long-term effects are uncertain, we know that the global pandemic will fundamentally transform how people interact with each other. And, technology will play a key role.
In finance, of course, technology has already had a transformative impact. Traditional banks now compete with digital banking products and services from technology giants (Apple, Google, Facebook and other so-called “BigTechs”) and financial technology (fintech) companies.
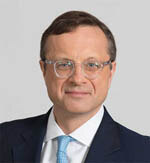
These firms offer broader and easier access to financial services (including credit) - but also, potentially, carry more risks. What's more, they are now using innovative, disruptive technologies like artificial intelligence (AI) for everything from credit underwriting to fraud detection and anti-money laundering.
The rapid evolution of tech in finance dates all the way back to the late 1990s, when many banks introduced 24/7 digital access that offered account management services. Around that time, moreover, digital platforms, such as LendingTree and Lending Club, emerged as financing intermediaries, and PayPal revolutionized the payment systems landscape.
PayPal, in fact, paved the way for other technologically-driven retail payment providers, such as Square, to compete directly with traditional banks. Specifically, Affirm pioneered point-of-sale (PoS) financing: installment loans offered to consumers during the checkout process at the PoS, delivered in the terminal itself.
Rapid Product Development: A First-Hand Example
The speed of technological innovation in financial services, outside of regulated institutions, has been greatly accelerated by the lack of rules that generally well-meaning regulators impose. In my experience, product design and development in an unregulated, non-bank environment takes a fraction of the time (and cost) compared with similar changes in a regulated bank environment.
A few months ago, I worked with a PoS network provider with a dominating presence in an emerging market, facing the strategic opportunity to extend credit through its well-established network. (Confidentiality prohibits me from providing specific details, including the company's name and headquarters.)
In this PoS' home country, almost everyone uses bank accounts to pay utility bills, including cell phone bills. Banks, moreover, extend credit chiefly to government employees and employees of large corporations, thereby effectively excluding access to credit to small and midsize corporations and their employees.
To meet the demands of a large, underserved market, the PoS network provider was in the position to offer credit - yet it needed to understand the market, its behavior and the right approaches to evaluate credit, absent credit scores.
We approached the problem by developing machine-learning models to identify segments where transactions exhibited lower fraud risk and less frequent non-payment.
What's more, to improve credit evaluation, traditional credit assessment (including credit scores, which do not exist in the POS' home country) were eschewed in favor of another set of machine-learning models. These models combed through large swaths of unstructured information, bank account transaction information and other digitized data (such as cell phone usage) to assess individual capacity and willingness to pay.
To extract meaningful signals, the models were trained on high quality, clean and well-engineered e-commerce, web interaction and social network data - among other alternative data sources.
Transparency and model explainability issues were addressed through the adaptive usage of alternative methodologies. The model validation process was designed to be dynamic and continuous, with new transaction data continuously feeding into the system - improving overall learning and refining performance.
Sensitivity to novel information, particularly the identification of outliers that can potentially manifest themselves as (for example) fraudulent activity, was integrated into the decision making.
Our models were not designed to be autonomous decision makers. Rather, model outputs should offer indicators to help humans in making better decisions. It is human-centered artificial intelligence (AI) that supports the credit decisions, as loans are extended to customers in an otherwise under-served market.
BigTech Financial Disruption
If a small, well-funded, tech-savvy fintech in an emerging market can use AI to develop a new business model quickly, then it's clear that technology's true potential to disrupt finance is tremendous. The only strategic barriers are established business practices and complex regulatory approval processes.
However, BigTechs like Google, Facebook and Apple have recently muscled their way into financial services, and can overcome these technological obstacles with relative ease and agility. These firms have large-scale networks and huge user bases. Indeed, with their captive audiences, BigTechs can design business models at a lower cost, leveraging the data generated by their user interactions.
While banks offer low-cost digital banking access 24/7, their online subsidiaries typically cannibalize revenue. Goldman Sachs' Marcus - a predominantly a retail banking franchise - is one notable exception to this rule.
In contrast, if BigTechs could obtain banking charters in the U.S., they would not eat away their own businesses. Rather, with a lower cost base and more nimble product development strategies, they would be able to compete with traditional banks.
While no digital bank has received a charter to operate in the U.S. (Varo has passed some, but not all, regulatory hurdles), upstart digital banks in Asia have emerged, backed by large technology or telecommunications players - without the support of banks.
For instance, in China, TenCent's WeChat offers payments and microcredits through its subsidiary, WeBank. (These services are integrated into its online platform.) Moreover, Kakao, a South Korean mobile messaging service, operates a digital bank.
Asian BigTechs integrate financial services into their platforms across their extensive ecosystems: through their own services and, indirectly, through third-party, rebranded services. TenCent, for example, relies on network effects, large-scale product development and capacity for rapid deployment, as well as the pragmatic use of AI.
In the U.S., meanwhile, both Apple Pay and Apple Card are successes, but they are not pure fintech solutions. Apple, without a banking charter of its own, sources banks (including Goldman Sachs) to provide services under its own brand.
Risks of Digital Banks
Many digital banks (both digital franchises and branches) seek to meet aggressive balance sheet growth targets, which they achieve through the combination of high-cost retail funding and generally lax credit underwriting. The tech culture expects and emphasizes (rapid) top-line growth, rather than quality growth.
To compete with established brick-and-mortar banks, upstart digital banks must absorb significant client-acquisition costs. This can have a negative impact on risk-adjusted return across the credit cycle.
Digital, tech-driven banks are potentially less resilient and more exposed to secular changes in the credit environment - a concern that has already piqued the interest of regulators. Moreover, having a digital-only presence presents unique technological risks. (Model risk and technology risk eventually morph into strategic risks.)
In addition to demands for better data protection and greater cybersecurity resilience, these banks also need to address concerns on the credibility of the business model itself: any susceptibility to hacking could critically destabilize the entire digital banking industry. In fact, the trustworthiness of all global payment systems was called into question during the SWIFT hacking scandal in 2016.
On top of reputational, business and strategic risk, digital banks that outsource services to smaller (independent) vendors also face considerable third-party risk.
Glimpsing the Future
The transformative power of technology in finance is likely to accelerate, further altering the banking landscape. BigTechs and fintechs are using innovative, disruptive technologies, like AI and machine learning, for everything from credit underwriting to fraud.
Ultimately, digital banks will compete with traditional banks by offering, for example, broader, easier and sometimes more equitable access to banking services. While they also carry unique risks that make their future somewhat unclear (particularly in the U.S.), it is certain that the regulatory playbook will have to adapt to a digital future.
Peter Went is a lecturer at Columbia University, where he teaches students about disruptive technologies like artificial intelligence and machine learning, and their impact on risk management.