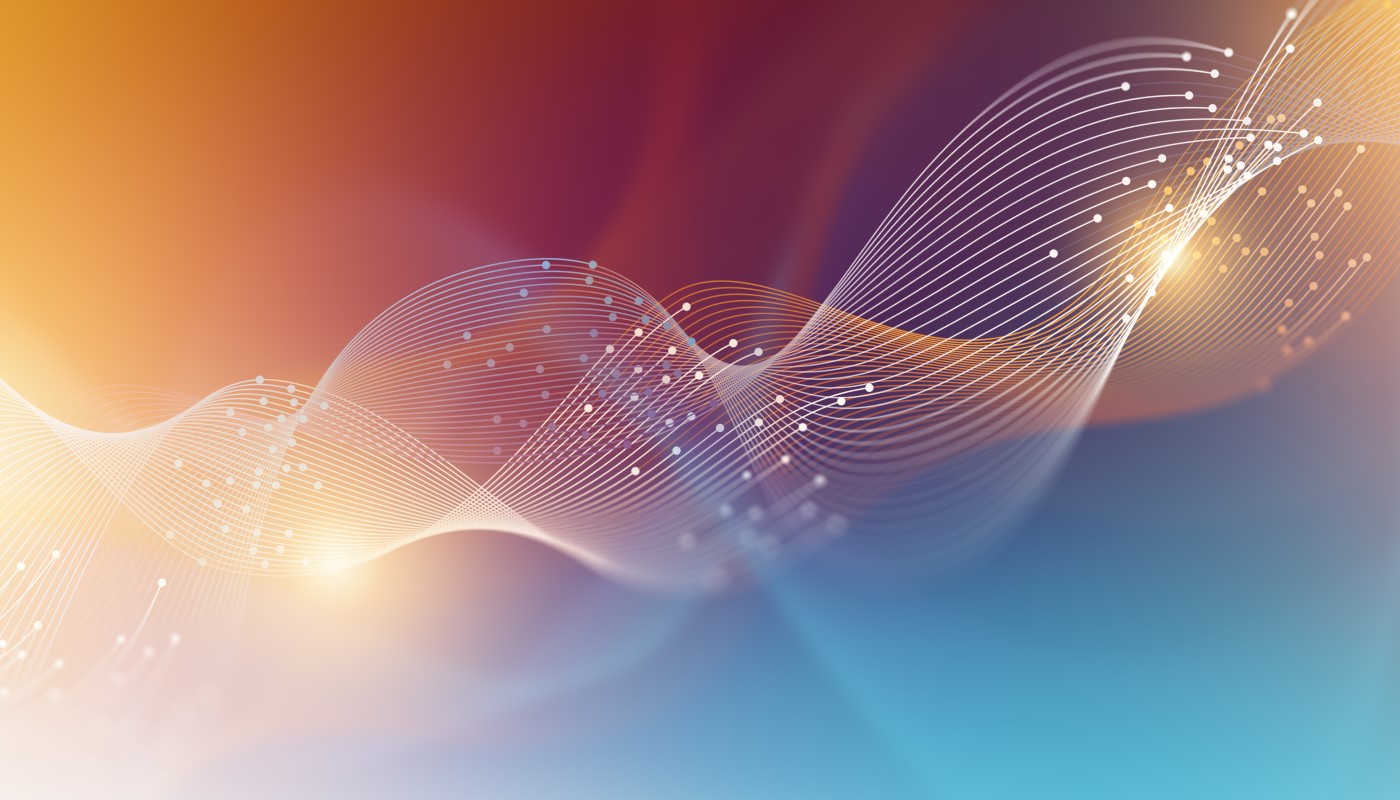
Data has come to be known as “the new oil,” and as with motor oil, data comes in synthetic forms. Longtime risk manager Aaron Brown recalls pioneering work in the 1980s and '90s, “except we didn't call it synthetic data back then,” he says. It was test data for scenario analysis or stress testing and was produced with human oversight, based on statistical parameters.
Today, juiced up with artificial intelligence and other high-tech tools, synthetic data has new buzz, brings new benefits, and risk management is again on the frontier.
“Now we have machine learning people coming to risk managers and saying, 'We have these shiny and new, synthetic data generation tools - would you like to test them out?'” says Brown, formerly of AQR Capital Management, currently teaching financial mathematics at New York University.
Brown sees “fruitful” new iterations of synthetic data that will result in more systematized practices, augmenting and perhaps ultimately replacing human risk management functions. A onetime GARP Risk Manager of the Year, he muses, “I don't know if there will be a Risk Manager of the Year in a decade. Instead, there may be an algorithm of the year.”
Traditional synthetic data - also referred to as fake, simulated or dummy data - was often used in place of the risk of employing data that contains customer or transactional data and other critical information. However, such data could be reverse engineered, and thus did not fully protect consumer privacy.
Proponents of the new synthetic data say that when properly generated, the updated approach offers more precise modeling, cannot be reverse engineered, protects privacy and assures other regulatory compliance. It also addresses the need to feed AI-powered models large quantities of relevant data to ensure that they are trained adequately and work correctly.
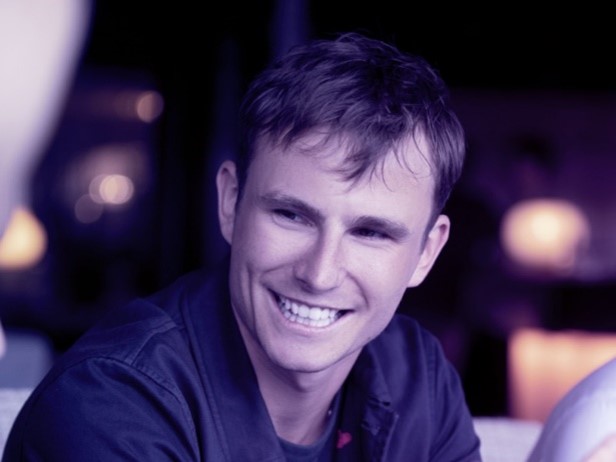
“So much of today's data infrastructure is based on using raw data, which represents a great deal of risk in finance,” explains Harry Keen, co-founder and CEO of synthetic data generation company Hazy in London. “With widespread adoption of [the new] synthetic data, we will see a significant drop in earth- shattering headlines about data leaks and thousands of data records being stolen or lost. That sort of story won't exist anymore.”
Open-Source and Safe
The new synthetic data is generated by machine learning algorithms, some of them open source and readily available. They replicate the often complex attributes of raw or real-world customer and transactional data, but without the personally identifiable information (PII) that reflects back to the original source and that could be highly problematic if falling into the wrong hands.
Hazy is one of a host of firms touting synthetic data generation for the financial industry. Others include Facteus in Portland, Oregon; Mostly AI in Vienna; Simudyne and Synthesized, both based in London; and Statice in Berlin. Truata, a Dublin, Ireland synthetic data software start-up is backed by IBM and Mastercard.
Last year, open-source data generation tools called Synthetic Data Vault came out of the MIT Laboratory for Information and Decision Systems.
Networks That Learn
Artificial intelligence is the game changer. Data strategy consultant Stefan Jansen, founder and lead data scientist of Applied AI and author of Machine Learning for Algorithmic Trading, says a data generation process known as generative adversarial network (GAN) takes the place of humans in creating guidance or parameters for producing synthetic data. It relies on deep neural networks and learns by trial and error “to capture a much more complex reality” that precisely mimics real-world behaviors, consumer banking habits or fraudulent activity.
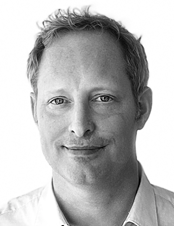
“It's much better if the computer learns from the data and makes probabilistic judgments based on it,” Jansen says, though he adds, “I am coming to this from the point of view of machine learning science rather than that of a risk manager.”
Particularly attractive to the financial industry is the fact that such data can be produced with smaller staffs, at lower cost, and in larger quantities than with older technology or manual methods. Rich, diverse and clean datasets are key to training and validating sophisticated models.
AI modeling success depends on “both high quality and high quantities of data, but, with all the privacy regulations in place today and competitive concerns about sharing transactional data, it is very hard to achieve that,” says Steve Shaw, senior vice president of marketing at Facteus. “With synthetic data generation, you can create a large quantity of data that is statistically relevant and yet safe to use.”
Speedy Coding
According to Petter Kolm, clinical professor and director of NYU's Mathematics in Finance program, “Today, the appropriate software libraries and tools are so easy to access, a student with the right Python coding background can be up and running with a machine learning model and synthetic dataset in a few days.”
Aaron Brown says, “Instead of dozens of PhDs working on a model, with today's machine learning capabilities, maybe all you need are three or four good programmers and a risk manager's knowledge.”
Jansen points to two types of machine learning. One, convolutional neural network, excels at producing synthetic data focused on imaging and image-focused models. Applications include medical diagnosis, autonomous vehicles and disaster planning.
The second type, recurrent neural network, is tailored to produce data that has a sequential structure, as in time series common in finance. Standard Chartered, for one, has been experimenting with a recurrent neural network technique known as restricted Boltzmann machine (RBM) to create synthetic datasets based on private client data, previously inaccessible for model development.
In an April 2020 Risk.net interview, Alexei Kondratyev, managing director and head of the bank's data analytics group, said it started to look at GANs to do probability distributions in a non-parametric way, and “we were somewhat surprised it hadn't been done before.”
Early Stages
Such tools in the financial sector are in the experimental stage, Jansen says, and there is more to learn about deep-learning model performance. But it is known that quants at large hedge funds and banks as well as fintech firms are actively testing.
“The basic idea of having deep learning techniques applied to the generation of synthetic data is barely five years old,” Jansen says. “It was applied to time series data only three years ago in the medical field, and only two years ago in finance.” Results are promising, but 100% efficacy is not guaranteed.
According to Luther Klein, managing director in Accenture's Finance and Risk group, there are multiple reasons for risk managers to get up to speed with synthetic data. It can safeguard data privacy in model building or data sharing; allows for more robust testing for gender, racial or other protected classes in models; and can facilitate the rapid buildout of stress-testing scenarios and of challenge frameworks or models.
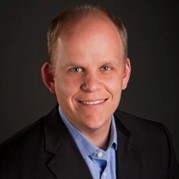
Klein sees risk managers playing a critical role: “We will need risk managers to run and manage the complexity of these new models, using their skills in data analytics to understand the risks that are identified and then to integrate their findings into business decisions, qualitative measures, and the risk profile of the company.”
Klein says synthetic data can advance model performance in fraud, anti-money laundering and Know Your Customer; cybersecurity and data monitoring; and in testing for bias. “Over the next two to three years we will see further innovation and utilization of synthetic data in all these areas,” he says.
Overcoming Limitations
Alexandra Ebert, chief trust officer at Mostly AI, says the most common applications to date are in fraud prevention: “In most fraud datasets, you only have a tiny percentage of cases to work with, but with the use of synthetic data, you can boost the signal strength of your data, and this allows your algorithms to better predict outcomes.”
Ebert anticipates growth in the area of open banking, which may involve sharing of data with third parties, and sees banks testing more outlier or edge cases in their models and in new technology platforms they may have acquired.
“Rather than conducting tests with the average performance data you have, synthetic data can include edge cases that allow you to figure out if your new systems or models are performing as thoroughly as you require,” she says.
Michael Dowling, professor of finance at the University of Dublin and co-author of the paper AI and Machine Learning for Risk Management, notes that historical market data is a limited dataset on which to base risk projections. The result can be overfitting, or a situation where modeling errors occur. “Synthetic data can provide financial risk managers with new time series data to double check their market risk models,” he says.
He says synthetic data will boost the quantity of data available for operational risk models, credit card fraud models and “any low-frequency event that can be catastrophic.”
Account for Outliers
Sebastian Weyer, co-founder and managing director of Statice, advises risk managers to keep in mind that “synthetic data can be used for any type of business analysis problem,” and particularly when training data-hungry, machine learning models.
Yet, Accenture's Klein points out, because synthetic data can only mimic real world data, it may not cover all outliers, and that “could be exposing your organization to risk beyond what is acceptable. So it is important to understand the quality of your data source and to question if the quality is highly correlated or not.”
Dowling at the University of Dublin says users should question whether their synthetic data is retaining biases that may exist in the natural data. “Is it allowing discrimination based on gender or ethnicity?,” he asks, noting that the Apple credit card that was introduced with Goldman Sachs in 2019 encountered gender bias complaints. Users of synthetic data, Dowling says, need to be aware of such hazards and adjust models accordingly.
Kolm at NYU warns that with the greater availability of data, financial and market prediction models, in particular, can become more sophisticated and complex, and thus more difficult and costly to employ.
“Such models are extremely data hungry,” he says. “They require far more data to be trained on, and far more computer power,” and so it is possible that with broader use of the new synthetic data, large financial firms that have access to extensive or supercomputing power may gain significant advantages over other market players.
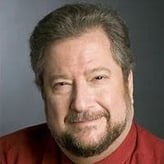
Risk expert Brown sees both positives and negatives: “It will improve the quality and precision of models,” he says, though getting better predictions is half the battle. The rest involves risk managers persuading others to employ the new tools, derived from “a black box built by a long-haired guy,” along with a recognition that no matter how great machine learning is, there will always be unknowns stemming from tail events.
“Big data and machine learning might have gotten us down from 99.01% unknowns to 99% unknowns, Brown says. “While that does make a difference at the margins, it does not eliminate crises or make them fun. It's like getting five minutes more advance warning of a tornado, which helps some people get out of harm's way, but it doesn't stop the tornado from doing a lot of damage.”
The bottom line in Brown's thinking: “We need a new generation of risk managers who understand how to use synthetic data and machine learning models, but who also have the credibility to change human behavior” and help people put the models' findings to constructive use.
Katherine Heires is a freelance business journalist and founder of MediaKat llc.