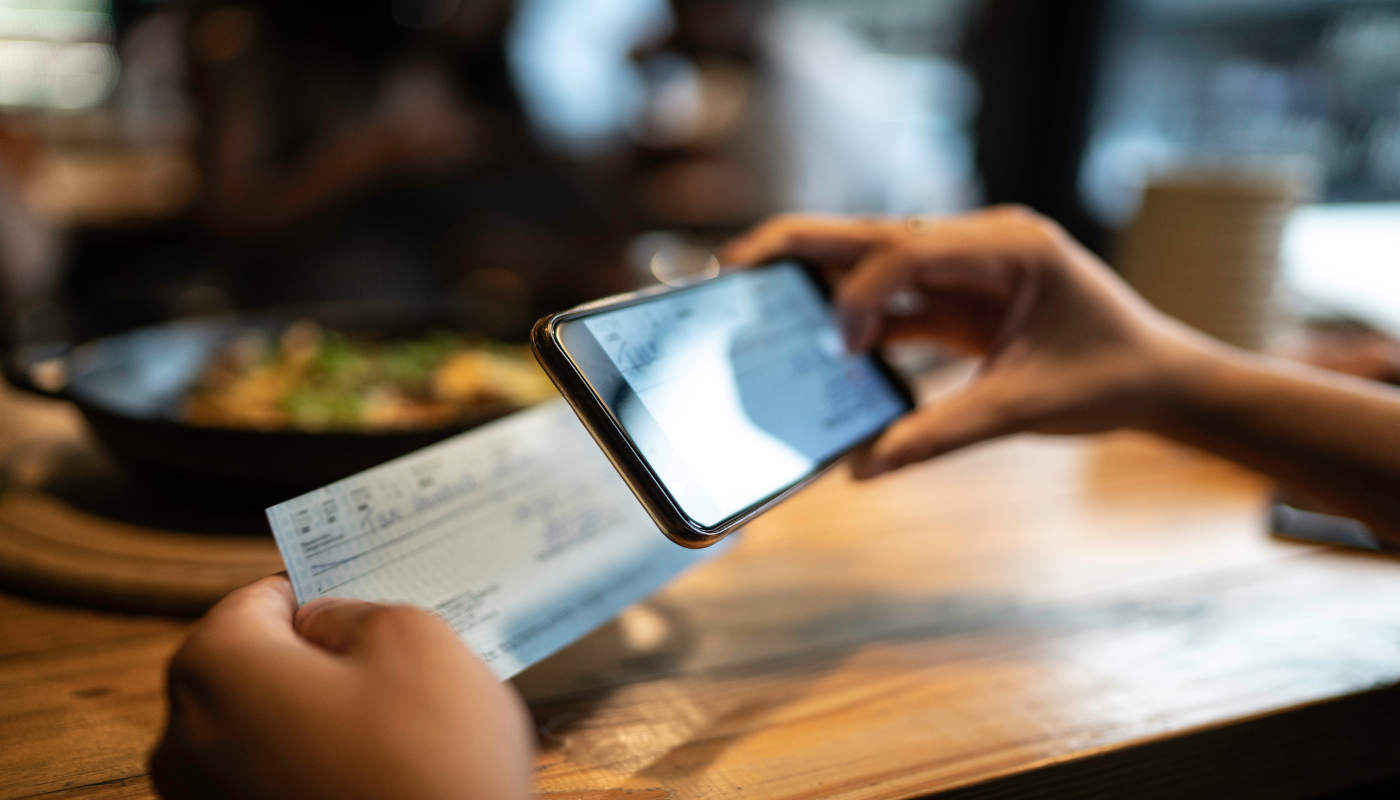
Earlier this year, in just a few weeks, COVID-19 forced greater adoption of innovative technologies and accelerated a worldwide digital transformation. In financial services, operating models and commercial strategies were disrupted. As banks, asset managers and other financial services companies (and their clients) adapted to a more online, mobile, remote world, they had to quickly reassess their strategies and recalibrate their growth opportunities.
Robotic process automation (RPA) is emerging as a key strategic component for banks in this rapid digital transformation. In fact, McKinsey estimates that automated, AI-driven technologies could potentially deliver up to $1 trillion of additional value annually in financial services worldwide.
According to the ECB, digital banks have a sizable cost advantage. Their cost-to-income ratio of 47% compares favorably to the traditional banks' 73%.
It is therefore easy to understand why automation only stands to increase in the future. However, the recalibration (e.g., of existing businesses and strategic dogma) that is now required is fraught with idiosyncrasies.
Reimagining Workflows and Confronting Model Risk
Intelligent automation and advanced analytics create vast efficiencies, as well as the opportunity to scale and optimize processes. But, as workflows become increasingly digitized, algorithmic and interdependent, risk managers need to understand how to manage a slew of complex and opaque risks.
In addition to identifying, managing, measuring and mitigating traditional business risks, they must comprehend how tech-driven operational risks interact with each other. Risk managers working in highly digitized firms will, moreover, need to have a keen understanding of model risk management to do their jobs.
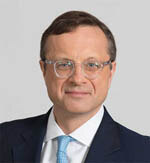
It is particularly important for firms that are in the midst of their first true digital transformation - with limited prior exposure to redesigning end-to-end digital workflows across the organization - to recognize the toll of complexity and the cost of managing the same. The financial benefits to digitization are impressive, but they come at a cost.
Poor model risk management and model governance accounted for an uncomfortable number of unexpected outcomes at a large bank with which I worked earlier. While I cannot name this bank, I can tell you that it has an impressive commercial lending portfolio.
For its small- and-medium-sized enterprise (SME) customers, it envisioned a close to fully-digitized operation. To advance this digital vision of its business, the bank launched a large-scale RPA project that was intended, in part, to streamline its customer relationship management processes.
The bank approached the RPA project methodically, automating the processes for a group of potentially low-risk customers, before scaling up to include increasingly complex and more risky customers. In scaling up processes, business complexity increased, and a notable number of processes encountered unexpected variations. In part, these unexpected results (effectively processing defects) were a reflection of increased technical, algorithmic and infrastructure complexity - some of which could have been prevented.
Root-cause analysis clarified that some of the bank's key RPA models were documented poorly, hastily and unclearly, yet passed the reviews of an overworked and understaffed model risk assessment team.
Recognizing the iterative nature of RPA programs, the bank revised some of its other operational processes, before further automating some other key compliance and risk management business processes. It also added some additional key analytics to streamline customer onboarding and validation, which ultimately led to an increased standardization of model documentation and model risk across the organization.
Financial services firms with increased digitization will experience upticks in model failures: both in the sheer number of model risk problems and the complexity of these failures. And with models touching on all parts of the business, including those that were not sufficiently digitized and are not expected to become digital yet, risk managers (particularly model risk managers) will need to develop a deeper understanding of business processes and the limitations of complex, sophisticated algorithms. In short, risk managers will have to leave their comfort zone.
Most importantly, when developing new digitized businesses, model risk managers and the C-suite will need to learn how to balance complexity with execution.
PPP and Risk Management: A Cautionary Tale
During the pandemic, we've seen a strong rise in banks' adoption of third-party lending platforms. In the U.S., this increase is generally attributed to the Small Business Administration's Paycheck Protection Program (PPP) - a pandemic response with partially forgivable loans aimed at the neediest businesses (mostly SMEs). As the number of SMEs applying for these loans snowballed, banks had to turn to external vendors to manage this process.
Even before the pandemic, banks were increasingly relying on third-party services to alleviate technology bottlenecks and increase the efficiency of internal systems and processes. (One bank in Singapore reported that its number of new digital accounts increased 2.4 times in Q1 2020, compared to the same period in 2019.) But the scale of the PPP was unprecedented.
To address the demand for PPP funds, many banks chose to rely on third- party loan management solutions, adding integration risk to their risk management considerations. Financial technology (fintech) companies offered easy access, rapid loan processing, and integrated funding, benefitting both the banks and the borrowers.
Moreover, due to the rush to put PPP money into the borrowers' bank accounts quickly, the SBA allowed banks to rely on borrowers' own certifications to determine their eligibility for loans. (We all know how well 'liar loans' worked out in 2007.)
To monitor some of the more obvious concerns of third-party risk effectively, a bank's risk management function must combine well-integrated processes with good understanding of the limitations of each of the vendors and processes involved in the complex daisy chain of managing digitized loan applications, account openings and credit decisions.
Of course, outsourcing operational processes to trusted third-parties is hardly a foolproof approach. For example, when processing the avalanche of new loans and onboarding new customers that the PPP precipitated, banks could not always rely on their own KYC systems, diligence checks and onboarding processes. Indeed, integration between systems did not always work as intended.
For instance, anti-fraud analytics were not always scaled up to manage successfully the large PPP demand. Aggregating and independently verifying information provided by the borrowers - such as eligibility information, payroll data and operational history - effectively slowed down automated underwriting and funding process, eating into margins and earnings.
It should come as no surprise, then, that PPP fraud is likely to be massive. To take advantage of the flexible, grant nature of PPP loans, some borrowers created fake businesses, while others received funds using stolen identities.
What's more, JPMorgan Chase - which has distributed in excess of $29 billion of PPP loans this year - recently acknowledged that some of its employees and customers acted in bad faith.
The extent of fraud has likely been mitigated by financial institutions with well-functioning KYC programs and stringent credit underwriting criteria. However, a quickly increasing reliance on third parties (like fintechs) has complicated the process. For many banks, the rapid development of PPP lending put increased pressure on their innovative - but novel - digitalized solutions.
While failure of key models was grudgingly expected, banks will have to recalibrate their approach to address inefficiencies in model governance, model risk and similar risks in an increasingly digital future. In our digital future, model risk management will be a strategic risk.
Parting Thoughts
The COVID-19 driven digitization of banking business models has opened up novel model risk management concerns. Banks should expect more supervisory scrutiny and more stringent regulation of their automated models, particularly key models with strategic importance.
But the core concern remains the complex and uneven trajectory of any post-COVID economic recovery. As credit losses are expected to accelerate, capital will become expensive, putting significant performance pressure on management.
How the C-suite will manage digital transformation to achieve sustainable growth - via investments in new technologies, cost reductions and enhanced workforce productivity - undoubtedly depends on balancing conflicting demands. As long as there is both political and financial capital to support broad transformation initiatives, these concerns will be addressed. When such capital evaporates, risk managers may become exposed.
Peter Went (PhD, CFA) lectures at Columbia University on disruptive technologies, such as artificial intelligence and machine learning and their impact on financial services and financial risk management. He also advises privately owned and publicly-traded companies in the U.S., and elsewhere, and is a senior advisor to a major opportunistic credit fund.
Article Updated: November 5, 2021