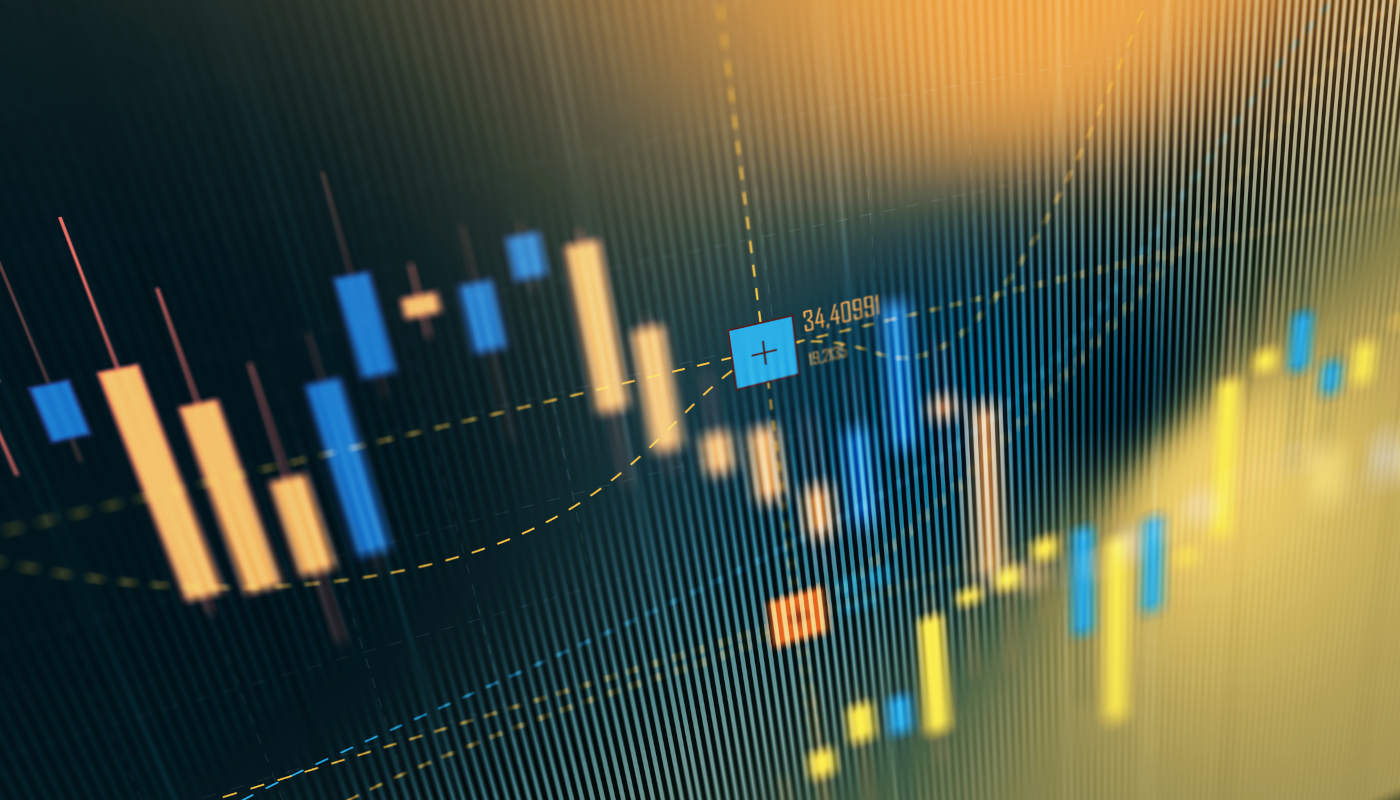
To improve alpha, quantamental portfolio managers combine fundamental analysis with various quantitative approaches. By identifying patterns and statistical relationships across fundamental and quantitative factors, advanced computational techniques drive quantamental strategies. These strategies pose a series of risk management challenges.
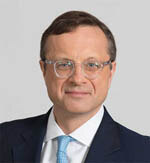
The fundamental approach to portfolio analysis focuses on a limited number of stocks, typically within a sector or some common fundamental characteristics - such as ROA, free cash flow generation or earnings yield. The quantitative approach analyzes vast amounts of financial and non-financial data. It uses various combinations of quantitative factors - such as momentum (runs in prices), liquidity (bid-ask spreads), volatility (range of price changes), sentiment (social and news media) and economic-driven data (including alternative data) - to identify patterns and trends.
How does this unique type of portfolio management work from a risk/return perspective, and what types of obstacles does it present? Let's take a deeper look.
The Dual-Factor Vector Problem
Since the quantamental portfolio selection process combines input from these fundamental and quantitative approaches, the portfolio return to is determined by algorithmically-weighted exposures to two different types of factors: (1) a vector that is based on fundamental information and can be directly observed, priced and hedged; and (2) a vector that is driven by quantamental information that is hard-to-price and hedge, and may be derived using sophisticated algorithms. These dual-factor vectors determine the outcome.
Certainly, some quantamental factors (such as volatility) can be priced, traded and hedged, but not all. Moreover, some widely-used quantamental factors, like sentiment data, are algorithmically derived. The dual-factor vector problem is essentially the qualitative differences between the aforementioned quantamental and fundamental factors.
Strategy development and asset-selection models are needed to validate strategies and build rigorous statistical models that better identify and manage sources of risk. Dual-factor vectors drive different types of quantamental approaches, including:
-
Factor investing, which is based on the characteristic factors of an asset (returns, volatility, profitability, size, market capitalization) - i.e., these factors' sensitivity (often called smart beta) to some specific index.
-
Risk parity, which is driven by differing sensitivities of financial assets to risk factors. Portfolio holdings are adjusted to reflect changes in risk volatility measures, where the relationship between volatility and portfolio allocations are determined inversely.
-
Statistical arbitrage, which exploits the short-term or long-term relationships between various factors.
All of these approaches require access to significant computing power and high-quality structured data.
The workflow does not significantly differ from the standard quantitative portfolio management, and includes (1) a sequential approach of data gathering, cleaning, structuring (labeling, mapping and creating the dual-factor vectors) and deployment; (2) screening for liquidity and tradability, size and execution factors; (3) cross-sectional alpha discovery and aggregation (e.g., statistical analysis and portfolio selection, weighing the relevant and identifiable factors from the dual vectors); and (4) execution.
However, the risk management process is complicated by modeling and computational complexities.
Managing the Risk of Quantamental Strategies
The steps in the traditional investment risk management process - establishing risk tolerance; identifying, measuring and monitoring risk exposures; and making adjustments, as needed - can be generally applied to quantamental strategies. But the dual-factor vector problem complicates that process.
Hedging is integral to any risk management toolbox. While hedging exposures to factors that can be priced is relatively easy using cash markets and derivatives, hedging exposures to the hard-to-price factors can be quite difficult. This limits the effectiveness of traditional hedging as a risk mitigation tool for quantamental managers, and is at the core of the dual-factor vector problem.
The black-box nature of the quantamental approaches also raises concerns about their algorithmic transparency and strategic explainability. Transparency is key, because algorithms identify patterns and capture the inherent nature of financial data.
To manage risks of a quantamental portfolio effectively, risk managers must perform a thorough model validation - and must understand the model's underpinning theory, performance and related inputs and assumptions.
Any misinterpretations about the underlying rationale of these models increases the burden on model risk management. Consequently, governance roles and oversight responsibilities are paramount.
Model validation, moreover, is critical, because it not only helps with model specification, development and deployment but also increases understanding the conceptual building blocks of the model.
While many machine-learning approaches work to extract patterns from digitalized pictures, weather phenomena and other information, financial prices and data typically exhibit serial correlation. It is important to heed Marcos Lopez de Prado's warning: outcomes of machine-learning models, including quantamental approaches, are frequently driven by statistical characteristics of return and price distributions, rather than by meaningful and objectively verifiable relationships between factors that generate real alpha.
Modeling Challenges
For quantamental models that rely on various data sources that have been enhanced algorithmically, there is a model-on-model problem. Model validation not only needs to assess each model, separately, but must also understand the interdependence between the models, their specifications, behaviors, and results - aka the model-on-model problem.
A good example is the use of sentiment data extracted from news, reports, online posts and other digital media. That data is collected through direct data feeds, web scraping and other collection tools, before it is aggregated and processed. Using various natural language processing algorithms, the emotional loading of words, expressions and business jargon is extracted, and this sentiment data is then used as a leading indicator (or quantitative factor) of price movements for stocks.
However, data quality problems can diminish the predictive capability of the sentiment indicators: how data is collected - and when it is collected, cleaned, aggregated and analyzed - all have an impact on the quantitative sentiment indicators. Often, it is the timing and the frequency of the data collection that has the most influence on the sentiment data - and not the data itself. Many low-quality vendors of sentiment data gloss over this important detail, particularly when they are offering machine-translated versions of sentiment data.
A New Paradigm
In the future, quantamental strategies will continue to combine immense computational power with sophisticated algorithmic pipelines. But the dual-factor vector problem also drives non-trivial modeling, pricing and governance issues.
Systematically constructed dual-factor vector strategies combine the idea of “thinking fast and slow.” However, the risk management aspects of the quantamental approach need to be properly understood and integrated better before portfolio managers can rightly herald their quantamental skills.
Peter Went is a lecturer at Columbia University, where he teaches about disruptive technologies like artificial intelligence and machine learning, and their impact on risk management.