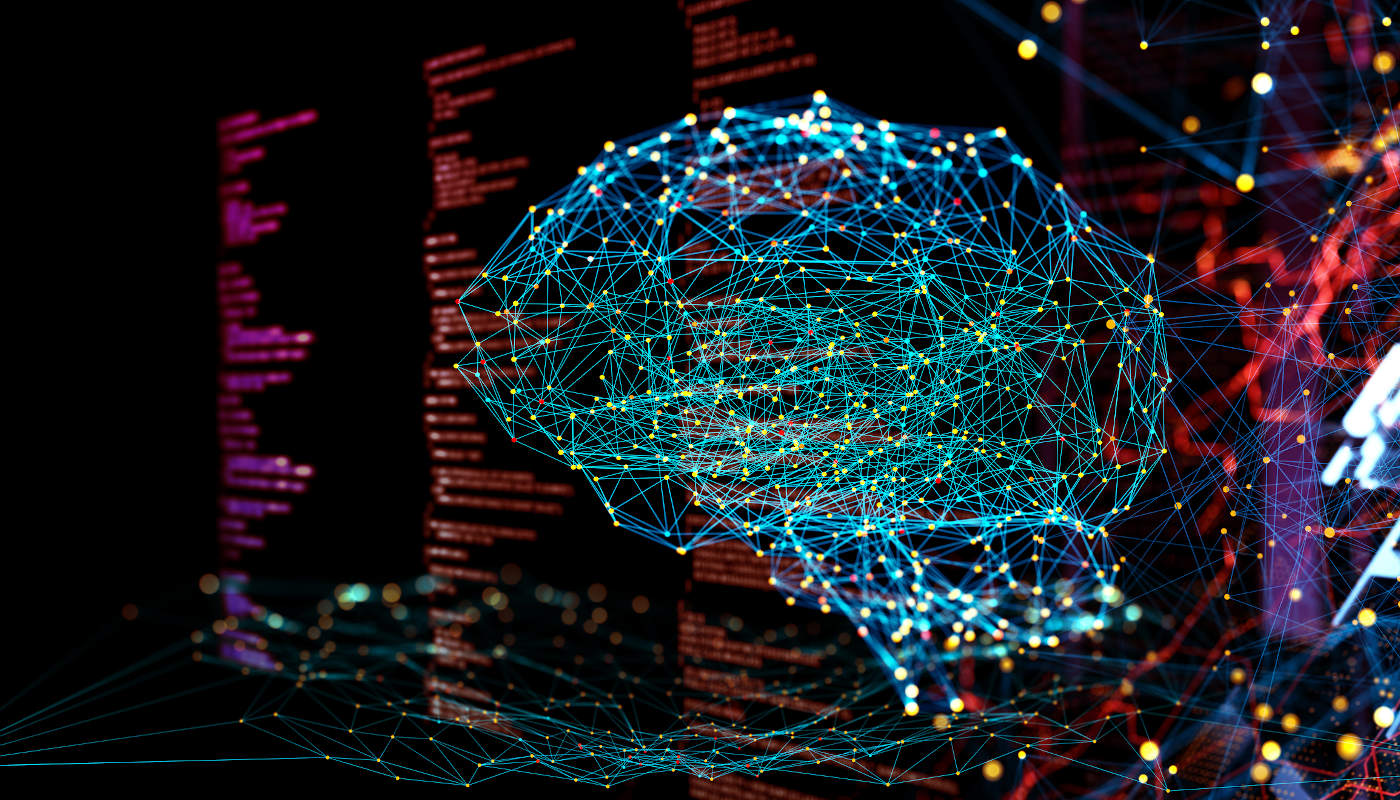
While artificial intelligence brings fundamental changes to quantitative and analytical modeling, risk managers are having to update and adjust their work processes to keep pace with the transformative nature of the technology and how it is being applied.
Jacob Kosoff, head of model risk management and validation at Regions Bank in Birmingham, Alabama, points out that traditionally, risk managers would make their assessments only after models were fully developed and ready for implementation. AI requires risk managers' involvement at multiple stages of model development.
“AI models,” Kosoff explains, “are more complex, require far more cross-collaboration, demand the participation of far more stakeholders, and involve the use of a lot more data and computing power, upwards of 1,000 to 10,000 variables in some instances.”
“Model risk management teams cannot be an afterthought or merely perform a checking role,” he continues. They “need to be effective advisers early on in the development stage. This way, everyone wins.”
Such realizations are underscored in a McKinsey & Co. article, Derisking AI by design: How to build risk management into AI development. They have influenced the design of machine-learning model management offerings from the likes of Algorithmia and Arthur AI, two start-ups in the monitoring category that were listed among research firm CB Insights' AI 100 for 2020.
According to Thomas Wallace, a McKinsey/Risk Dynamics partner in London and co-author of the Derisking paper, risk managers must ensure that a basic framework for AI risk management is in place from the start. They must ask fundamental questions about whether a model addresses the right business problem, employs appropriate or relevant data, can be sufficiently scaled up, and whether or not its purpose and construct are compliant.
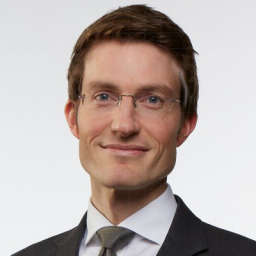
“In this new world, risk managers and analytics teams need to work much more in parallel and take joint responsibility when building AI models,” Wallace says.
Understandings and Tools
The McKinsey experts prescribe: a general understanding of analytics techniques as well as AI and machine learning risks, what can go wrong and how; awareness of best practices in testing for bias, fairness and model stability; an understanding of how data selection can decrease or exacerbate risks; and understanding the various roles of analytics team members in order to engage with these professionals in a knowledgeable way.
“Understanding how analytics teams work is critical,” says Wallace, and in turn, risk managers can adjust to a more agile work style and participate more closely at all stages of development.
“Risk managers are used to a model development program that can take six, 12 or 18 months, and they don't do much until the end of the development process,” says Wallace. But with AI, he explains, models are being developed much faster, and because of their complexity, risk management must be “baked” into the process.
In other words, tools to assist in model interpretability, bias detection, and performance monitoring are built into the technology and continually applied. Similarly, standards testing and controls should be embedded into various stages of a model's lifecycle.
Thus, Wallace says, risk oversight is called for at the ideation stage, or when the business use case and its regulatory and reputational context are being assessed; during the data sourcing period to help define what data sets are off-limits and what bias tests are required; and during model development so that the transparency and interpretability of the model is appropriate for the given use case.
“Good Monitoring”
A critical goal is “good monitoring of these AI models when they are being used, so you know what's going on,” Wallace says. “You can't let them into the wild and check on them a year later, as their performance can drift over time and they can do things you really don't want.”
The McKinsey report identifies workflow technology platforms as required tools that can facilitate the collaboration and cooperation among risk managers and analytics teams in such areas as documentation standards, recordkeeping, testing and model explainability.
Diego Oppenheimer, CEO and co-founder of machine-learning technology company Algorithmia, says a key benefit of such software is its ability to accelerate processes and group collaboration while avoiding the costs of a built-from-scratch platform.
“We saw at the beginning of the pandemic that all the data going into these AI models was wonky,” Oppenheimer says. Historical data that models had trained on turned out to be no longer relevant. Speedy adjustments were necessary - made possible by systems such as Algorithmia's. They integrate into the established processes of an organization, but also provide the right amount of document standardization, observability. and collaboration so that all members of a model risk management team have total visibility - what Oppenheimer calls “the who, how, where, when and with what data.”
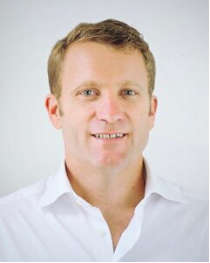
“Centralization of the model-building process is the avenue to achieve speed,” says Oppenheimer, and in his opinion, speed is critical to a data-driven organization that aims to successfully manage AI risk.
Data and Explainability
Arthur AI's real-time monitoring platform assists with model explainability - how it arrives at a particular decision - and any unintentional or emergent bias in models, says CEO Adam Wenchel. The system uses AI to identify data patterns, data drift, and any anomalies or bad decision-making that risk managers need to respond to in ensuring the reliability of AI models.
“We automate the many quarterly reports that risk managers might normally produce for very high value models,” Wenchel says. “A report that, in the past, took a risk manager several days to produce, is now an automated process,” thus freeing up risk managers to spend more time on how models are impacting customers or whether the needs of regulators are being met.
Wenchel adds that, increasingly, potential bias risk in AI models is detected by collecting demographic or subgroup data, or to have a third-party firm such as Arthur AI do so and measure different outcomes for different groups. This is in contrast to the assumption that without collecting such data, a model is less likely to discriminate. “Regulators are just starting to make the transition to this way of thinking,” Wenchel says.
Other providers of AI-powered model development and monitoring tools in CB Insights' AI 100 included Fiddler, Snyk, Darwin.ai, DataRobot and H20.ai.
Aside from new tools, Kosoff at Regions Bank says that risks can be dramatically reduced when there is broad AI training, so that everyone interacting with or making use of artificial intelligence - not just the model risk management team - is aware of its strengths and weaknesses and knows when to question its validity.
“When COVID hit, spending styles changed dramatically, and there were far more remote purchases,” says Kosoff, and what was once an indicator of credit card fraud - a spike in card-not-present purchases - became the norm.
“The fraud models suddenly lost their predictive power,” the banker says, “and when that happens, we want our fraud investigators - as well as others - to have the training and confidence to be on top of this.”
Katherine Heires is a freelance business journalist and founder of MediaKat llc.