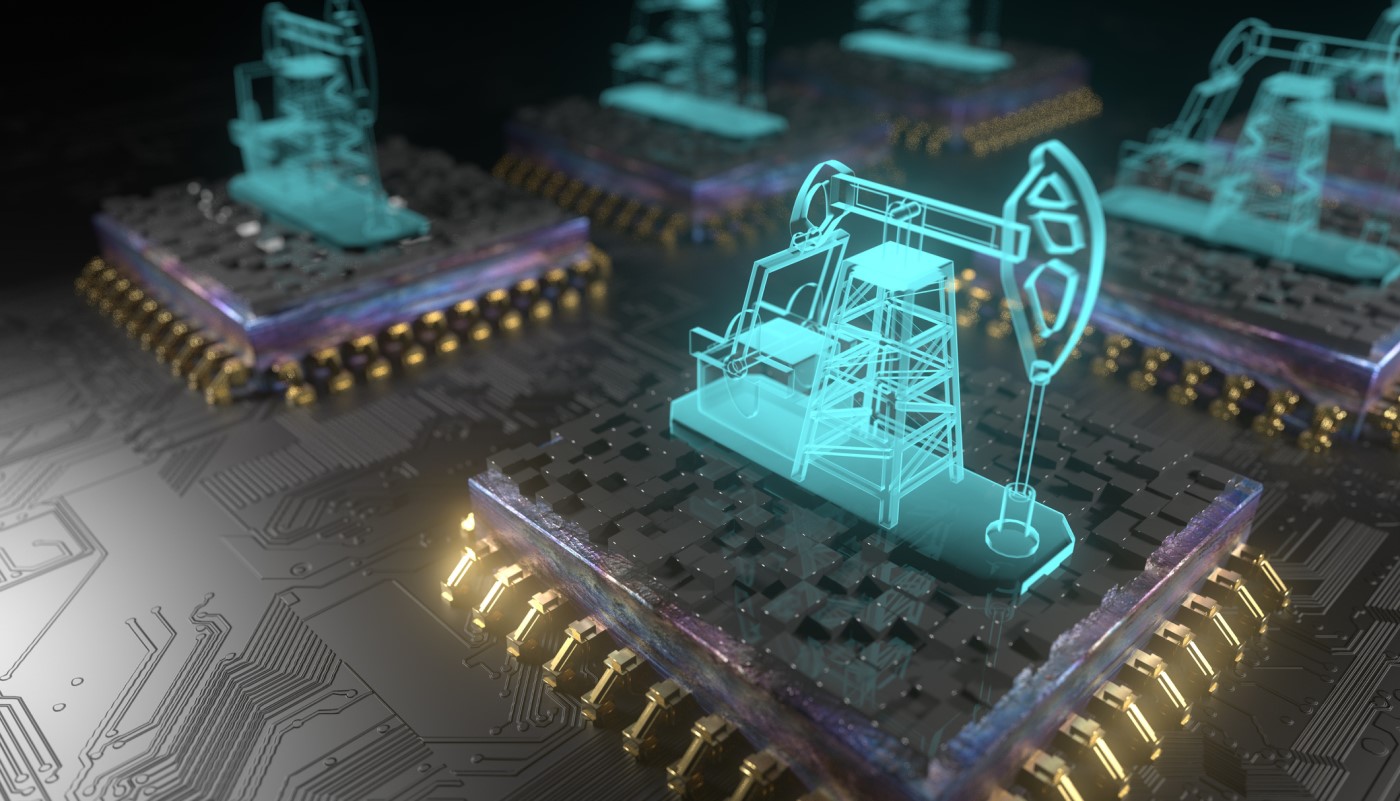
In a post-pandemic world where historical data has been so clearly found wanting, it should come as no surprise that synthetic data approaches are sprouting like mushrooms after a rainstorm. In the financial services industry, they are being used for risk management and stress testing, as well as for verifying the adequacy of hedges.
Like animal-friendly faux fur coats, synthetic data is manufactured - artificially created rather than resulting from actual events. It's a type of data augmentation that is helpful when the datasets available for training are small, or where their distribution properties have dramatically changed.
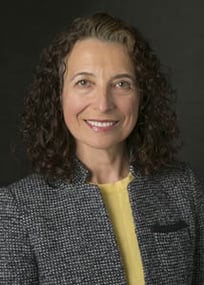
Modelers have always been restricted to just one history to test against. Synthetic data, in effect, provides modelers with thousands of histories to learn from.
Traditional stress testing with specific scenarios is one example (albeit limited) of this approach. When synthetic data is used as a supplement, one does not have to restrict oneself to just a few stress scenarios. Rather, it's possible to generate the full range of scenarios - not only for use in stress testing but also in planning or hedging.
There is, however, one problem: synthetic datasets generated by standard models only replicate the properties of historical data. So, a standard, synthetic-data-producing Monte Carlo simulation - with static covariances and deterministic drift - will not generate any unprecedented surprises.
Even though one can create an unlimited number of scenarios for testing using models supplemented by synthetic data, such models will not be able to capture “market caprice.” There is a good chance, therefore, that they will miss outliers.
Advice for Overcoming Historical Data Obstacles
Techniques for correcting this problem differ, depending on the intended use of the synthetic data generated. They include training-high frequency trading algorithms; marketing new products to consumers; and backtesting, forecasting, and hedging models for financial risks. So, let's discuss the techniques appropriate for risk management and capital allocation.
Two considerations are crucial: (1) most historical shocks are negative; and (2) it is necessary to learn separately from smooth and stressed historical periods, and then to combine the two. Let's now take a closer look at both of these issues.
Negative Historical Shocks
Markets are prone to sudden shocks that cause unexpected regime shifts - mostly with adverse results. This is supported by the fact that the average correlation between the S&P 500 and VIX indexes is close to negative 75%!
If markets were as likely to jump up as down, this correlation would be close to zero. Such strong negative correlation means downward movements are much more pronounced. Figure 1 (below) illustrates these facts.
Figure 1: Historical Levels and Returns of the S&P 500
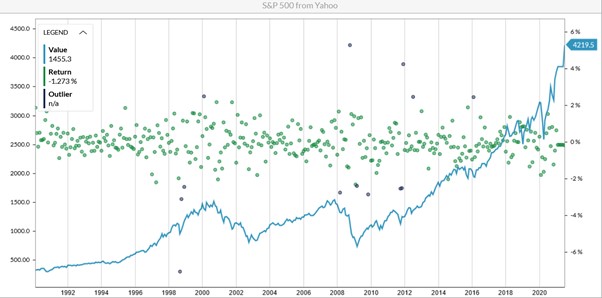
The blue line in Figure 1 shows the level of the S&P 500; the greed dots are returns, while the purple dots are outliers that happen with a small probability. As you can see in the chart, the outliers mainly coincide with negative returns. Moreover, the most abrupt movements have a downward direction, and - without strong government aid (as in 2020) - recovery from crises (e.g., after the dotcom crash of the early 2000s and financial crisis of 2008) is fairly slow for equity markets.
Learning from Both Smoothed and Stressed Historical Periods
It is wrong to consider that all data comes from the same types of distribution. In Figure 1, average returns over the entire period (1992 - 2020) are around 9.2%, with volatility of about 11.7%. But if one looks only at the periods without outliers, then expected returns would be 12.8% and volatility only 10.3%.
The outliers observed in this time period occurred during the Asian crisis of 1998, the tech bubble of the early 2000s, the financial crisis of 2008, and the Eurozone crisis of 2011.
Modelers can get a smoother picture of the S&P 500's dynamics by interpreting everything as a single distribution and by looking at the entire history - with stable markets and shocks intermixed. However, sampling from this type of distribution will not capture the potentially abrupt market changes that may be on their way - and this could give rise to flawed synthetic data.
The solution is to pull the data apart and sample separately from these two types of market movements, adding unprecedented shocks to historical ones. Subsequently, we can create multiple, forward-looking scenarios that will overcome the inadequacies of historical patterns and generate synthetic data appropriate for these rapidly changing market conditions.
Consider the following example involving one of the most unpredictable market variables: the price of Bitcoin (BTC). The chart of its historical values (see Figure 2) clearly demonstrates that prior to January 2020, Bitcoin data did not predict the growth that later ensued.
Figure 2: Historical Levels and Returns of Bitcoin Prices
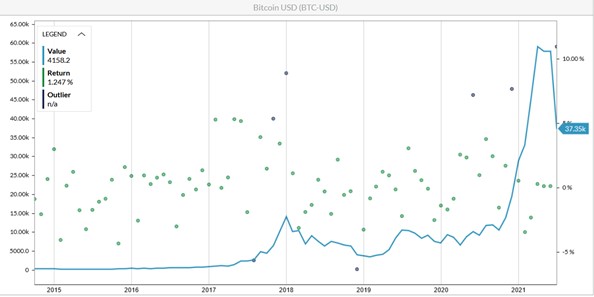
If we were to train our generators on the data prior to January 2020, and learn from a single set of historical outcomes, the statistical characteristics of the data would be a 59.7% average annual return and 73.8% volatility.
Synthetic data generated with these characteristics would be unlikely to cause the huge uptick in the price of BTC we observed later in 2020. But if the outliers were removed, the stable market growth rate would be 69.3%, with volatility of 70.9%. By overlaying previously-removed outliers and adding potential unprecedented shocks, one can generate synthetic data that includes future outcomes - such as Bitcoin's growth from $9,350 to above $63,000.
Moreover, if the separation of stable markets and shocks is applied, the forward-looking distribution based on synthetic data will include realized outcomes and more (see Figure 3) - even when synthetic data is trained on the period that did not have a huge growth spurt.
Figure 3: Histograms of Historical Values and Synthetic Data
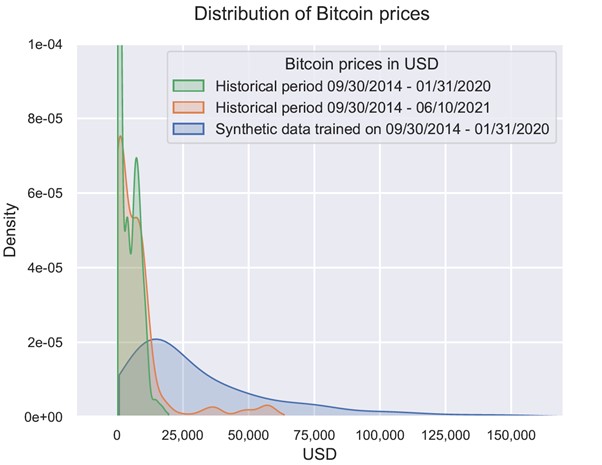
These principles are reflected in the generative models that follow the signature of the path, revealing various behavior patterns and generating more efficient synthetic data. Indeed, these types of models allows us to derive useful information even from small sets of features, and have their own way of separating smooth movements in available history from abrupt jumps.
Parting Thoughts
Everyone knows that having all the data in the world today will not help to predict the future. The idea behind generating synthetic data is not to predict the future, but, rather, to be able to analyze all potential outcomes with their probabilities.
This allows risk managers to ensure that scenarios for planning and stress testing are selected while considering the full range of outcomes; that business strategies are selected while anticipating all customer behavior patterns, even in unprecedented environments; and that hedging strategies - which must be dramatically different, in the tails and from the perspective of markets as usual - adequately meet all challenges.
Using synthetic data improves risk governance, as risks can be identified ahead of time and addressed through contingency planning. What's more, embedding forward-looking scenario simulation and what-if analysis in (almost) real time - and having risk and strategy considerations closely aligned - are vital to being ready for the unknown.
When used properly, synthetic data enables risk managers to build thousands of future lives that reflect the historical patterns of the past, while still including unprecedented outliers. This makes it possible to test risk models, financial plans and hedging strategies in a wide variety of future environments.
To overcome the inefficiencies of historical data, modelers just need to interpret data patterns with the additional step of separating different regimes.
Alla Gil is co-founder and CEO of Straterix, which provides unique scenario tools for strategic planning and risk management. Prior to forming Straterix, Gil was the global head of Strategic Advisory at Goldman Sachs, Citigroup and Nomura, where she advised financial institutions and corporations on stress testing, economic capital, ALM, long-term risk projections and optimal capital allocation.
Article Updated: May 20, 2024