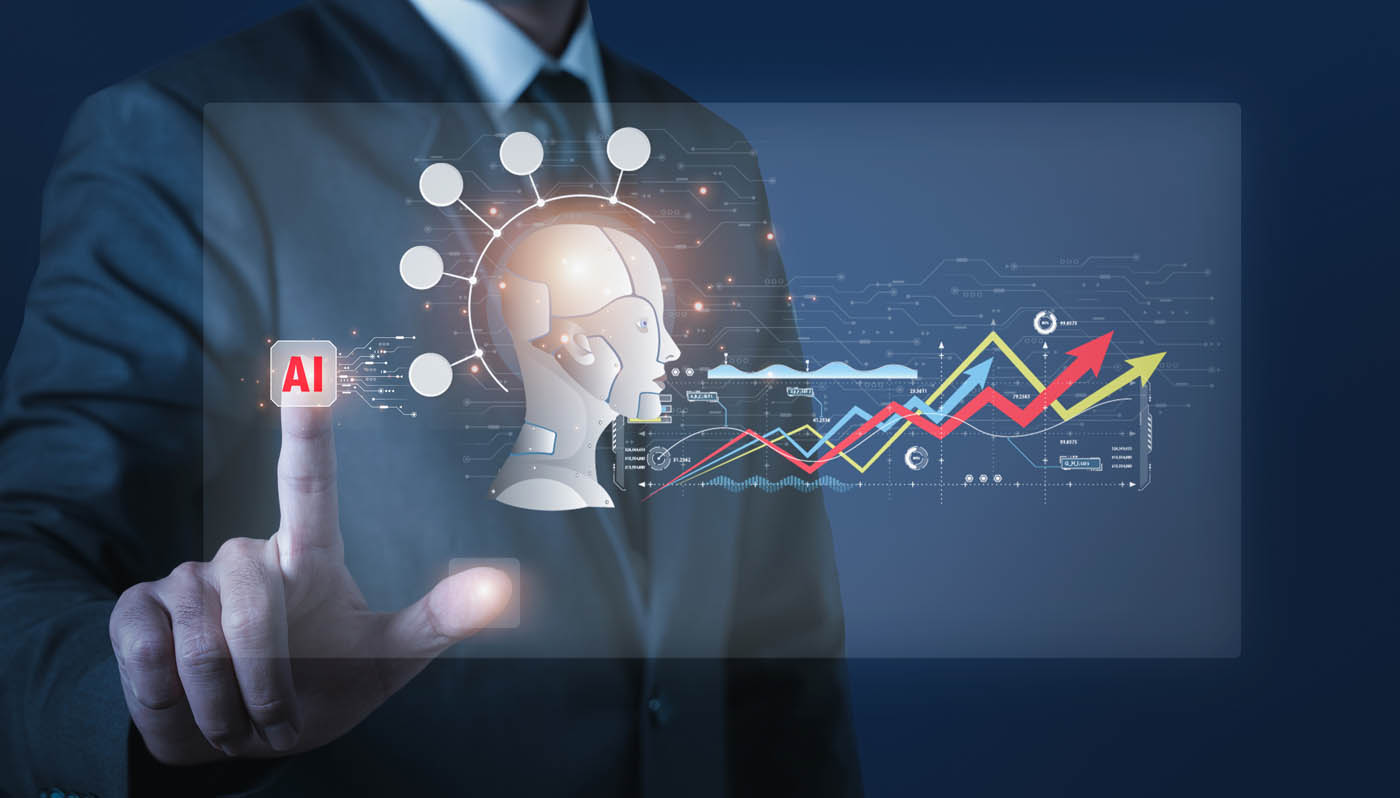
Artificial intelligence is seemingly ubiquitous in financial services today. Still, disruption and instability threats remain, and a new academic paper has raised another AI concern: reversibility.
Financial institutions are currently using this next-generation technology for everything from fraud detection, anti-money laundering and modeling to stock selection, data mining and quantitative risk analysis. But the paper by University of Chicago researchers Alex Kim, Maximilian Muhn and Valeri V. Nikolaev, Financial Statement Analysis with Large Language Models (LLMs), should give risk managers pause about the potential impact of ChatGPT and other LLMs.
Aaron Brown
The aspect that should make risk managers sit up and pay attention is that ChatGPT results are reversible. If ChatGPT likes a stock, it can not only tell you why but also offer guidance on what a business should do to improve its stock returns. This creates a bidirectional link – one that has the potential to improve both the economy and the financial system but also opens up the possibility of new types of disasters.
To gain a better understanding of this dilemma, we need to go back to the 1960s, when quantitative researchers were first looking at sports betting and analytics. There were two main approaches.
One was purely statistical and mainly ignored the sport, instead concentrating on the betting market. It produced rules like “bet the underdog on the road,” or “bet the team with a losing record against the spread.” Judicious application of a set of rules like these — and a few that were a bit more complicated — produced consistent profits.
The other approach was to gather a lot of information about the actual sport and estimate outcome probabilities by calculation or — more often — simulation. This proved to have little value for profitable betting, and was largely ignored for decades.
However, slowly but surely, this “sabermetrics” or “Moneyball” approach seeped into how sports were actually played. Starting in the 1990s, and taking off in the 2010s, we saw, for example, hockey teams pulling goalies earlier, American football teams going for more fourth down and two-point conversions, and basketball teams shooting more threes. This proved quite helpful to coaches and managers – unlike the betting-driven statistical approach.
In finance, statistical approaches have dominated quantitative hedge fund investing. Certain guidelines have made investors a lot of money – e.g., buying small stocks and shorting big ones, buying stocks that have gone up recently and shorting those that have gone down, and buying stocks with lots of book value relative to market capitalization.
These “rules,” however, did not teach us anything about how to run a business. Moreover, the great quantitative hedge fund managers have not been distinguished by either their success in running non-financial businesses or by the advice they have given to corporate managers. Their investments have not yielded any change: the same anomalies they were chasing in the 1960s are around today.
On the other hand, ChatGPT, and modern AI in general, can link finance and real economic decisions bilaterally. If an LLM can tell and investor what to buy or short, it can also tell a CEO how to run the company to maximize shareholder wealth.
This might transform the economy and turbocharge economic growth. But we have little historical experience with these kinds of bidirectional links. Like a short-circuit in an electrical system or a feedback whine in an amplifier, it’s possible to imagine one of these links getting into a positive feedback loop.
AI vs. Human Analysts
Another interesting, but hardly surprising, finding from the paper is that LLMs are, overall, superior to equity analysts with respect to forecasting.
The authors fed ChatGPT five years of financial statements for a large universe of public companies from 1966 to 2018, giving only the standardized account names and numbers, with company name and year redacted. They asked ChatGPT to guess whether next year’s earnings would be higher or lower.
The initial results were unremarkable. ChatGPT did about the same or a little worse than human analysts. While the human analysts had far more information, we know human experts are bad at prediction. Moreover, guessing whether next year’s earnings will be higher or lower is not a primary, or even secondary, concern of equity analysts.
Next, the researchers told ChatGPT to use standard value investor methods to analyze companies’ financial statements. This caused accuracy to jump well past human analysts, to near the performance of the best statistical methods. This is also expected.
It’s been known for over half a century that if you ask experts what they do, and program a computer to duplicate it, you get better results than the experts deliver. For reasons of both ego and career, experts like to insist that their field is an art rather than a science, and that they add great value with their experience and intuition, which help them know when to accept the results of the simple rules and when to overrule them.
The truth, however, is that experts generally subtract value by ignoring the rules. Experts have knowledge, but generally about simple stuff that computers can apply more systematically.
We’ve also known for a similar amount of time that you don’t need five years of full accounting statements to beat human decision makers. The Altman-Z score from the 1960s, for instance, uses five simple ratios to predict defaults better than rating agencies.
Simple rules, like “buy stocks with high book-to-price ratios,” beat the market. So, there’s no big surprise to the authors’ finding that one could generate significant positive alpha by buying the 10% of stocks ChatGPT was most confident would post earnings increases and by shorting the 10% that ChatGPT was most confident would not.
Why AI? Tight Coupling Lessons from Past Disasters
AI is also now helping to reduce a risk that has previously fueled financial disasters: tight coupling.
Seventeen years ago, just before the 2007 liquidity crisis that would later metastasize into the 2008 global financial crisis (GFC), risk manager Rick Bookstaber published A Demon of Our Own Design, which explored the role of tight coupling in major financial disasters.
Tight coupling is a natural consequence of efforts to make systems efficient, but it can also make them more fragile. For example, prior to Henry Ford, automobiles and other complex machines were built by keeping the machine in one place and by having workers move from one to another, performing their assigned task on each. This was a loosely coupled system. If there were a problem with one car, the worker could spend more time on it, or skip it to go on to the next car and deal with the issue later.
Ford introduced the moving assembly line, where workers stayed in one place and cars moved. This was more tightly coupled. Workers could no longer spend more than the allotted time on one car; if one worker skipped his step on a car, moreover, all downstream workers had to be notified to skip it as well. While the moving assembly line was much more efficient, even small problems could bring the entire line to an expensive halt — or could result in production of many defective cars.
Bookstaber noted that something similar had been going on in finance and correctly predicted that it would be a key factor in a systemic crisis. Problems could spread more rapidly than people were prepared to deal with them, into areas of finance far removed from the initial shock.
There were many responses to the 2008 GFC designed to insulate key parts from tight coupling – via segregating risky activities from essential infrastructure and adding capital to absorb shocks – without shutting down the financial assembly line. Regulations were introduced to stop institutions from mindlessly passing on defective items, which allowed downstream institutions to add layers until they blew up for a remote downstream user.
To technophiles like me, AI seemed a better solution than more capital and more rules. Instead of a dumb system that processed paper independent of economic reality, an AI algorithm could have made sure the loan was in proper shape for the next step (at each stage in the process), diverting problem loans to systems or people equipped to deal with them.
While AI could have helped with the tight coupling problem, there is also an argument that dumb coupling, rather than tight coupling, was at the root of the GFC. From this perspective, the issue with assembly lines was not that they linked steps together, but that they operated at a fixed speed, regardless of the actual work progress on each car.
A smart assembly line — which required technology a century more advanced than what was available to Henry Ford — could route each car to the appropriate worker only when it was ready and could divert problem cars to workers trained to handle them. This could be both more efficient and less fragile than a fixed-speed assembly line.
In financial terms, by 2007, some of the large mortgage originators were turning over their balance sheets every 36 hours. In other words, their salespeople were closing loans constantly, funding them with bridge financing from Wall Street, pooling them, securitizing the pools, breaking up the cash flows into collateralized obligations and selling them to end investors in a day-and-a-half.
Even a short interruption of this process caused their warehouse credit lines to balloon and hit ceilings. This made their commitments to finance mortgages, often made months earlier, impossible to meet. It also meant if there were problems (say, bad appraisals or missing documentation), loans were often too far downstream to pull out of securities and fix by the time the issues were discovered.
Generally speaking, the post-GFC reforms have not worked. Perhaps more precisely, they have not been trusted to work. In every financial crisis since 2008, regulators have not waited to see if the new regulations and capital requirements would prevent collapse, but have instead rushed in with the bailouts everyone always swears will never be giving again.
Indeed, over the past 15 years, massive central bank support has been thrown behind every major financial disaster or threat – including the rolling Euro crisis from 2010 to 2014, the corporate-bond market issues from 2016 to 2019, C0VID problems from 2020 to 2022, and Silicon Valley Bank and Credit Suisse in 2023. What’s more, there seems little reason to expect anything different in the future – at least until developed country sovereign debt exceeds the level at which governments and central banks have enough credit to backstop markets.
While AI is currently being used to mitigate many of the issues of tight coupling in finance, it has also introduced new concerns, as we’ve discussed.
Parting Thoughts
One of the big worries in modern finance is contagion — a problem that entails one link cascading into other links, and eventually making it back to the original location to begin a new process of amplified risk, leading to a blow up. But with the type of bidirectional links now produced by generative AI tools, like ChatGPT, you can get essentially the same problem in a single link.
The hope is that AI will make smart links that know how to invoke circuit breakers or other tools to prevent this. But rapid deployment of AI in finance is outstripping practical testing of risk management tools. Risk managers are not likely to change this pattern, but we can keep an eye on things, develop stress scenarios around them and prepare to survive a new type of disaster.
Aaron Brown worked on Wall Street since the early 1980s as a trader, portfolio manager, head of mortgage securities and risk manager for several global financial institutions. Most recently he served for 10 years as chief risk officer of the large hedge fund AQR Capital Management. He was named the 2011 GARP Risk Manager of the Year. His books on risk management include The Poker Face of Wall Street, Red-Blooded Risk, Financial Risk Management for Dummies and A World of Chance (with Reuven and Gabriel Brenner). He currently teaches finance and mathematics as an adjunct and writes columns for Bloomberg.
Topics: Tools & Techniques, Risks & Risk Factors, Responsible & Ethical