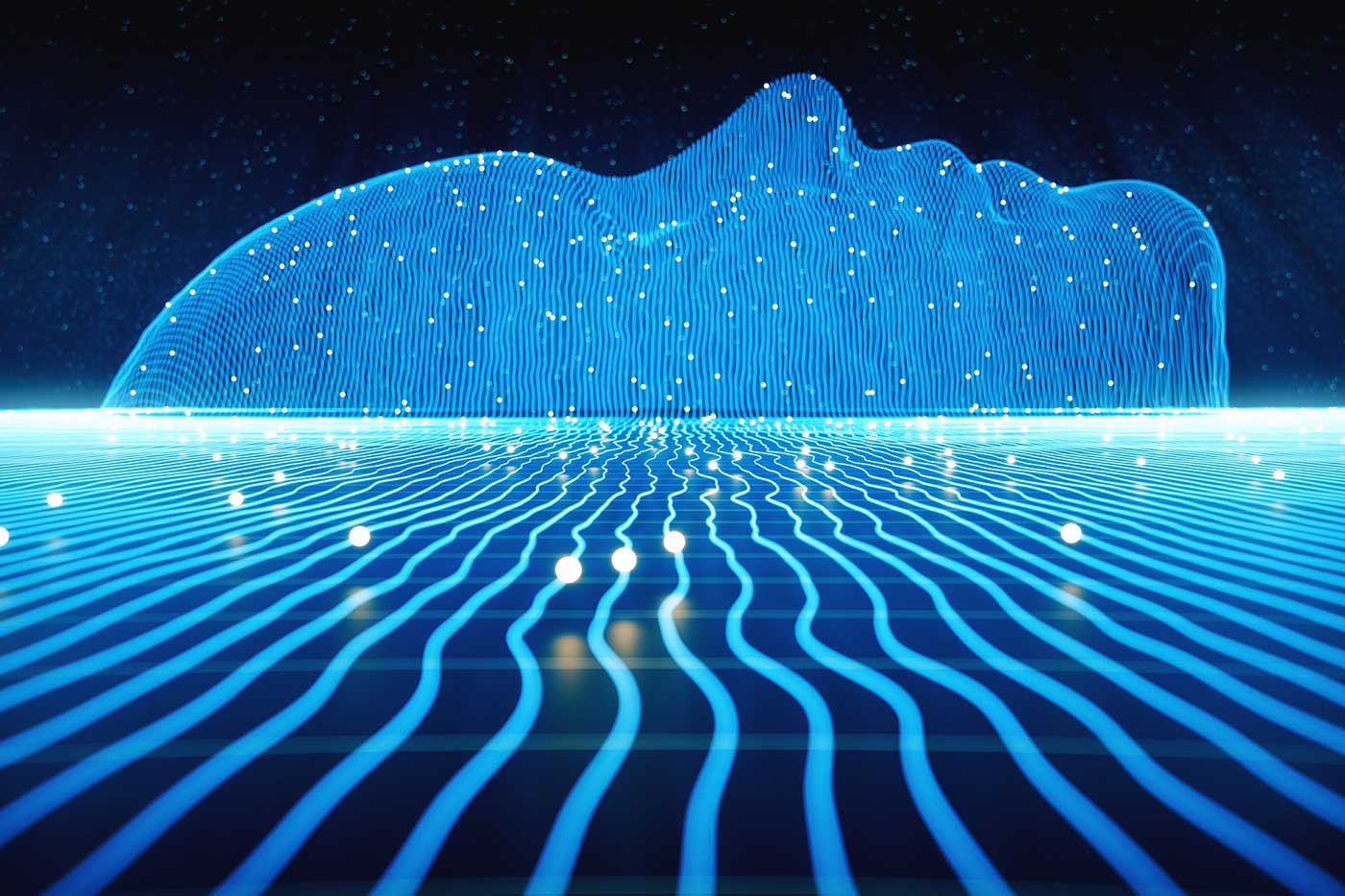
Artificial intelligence (AI) and machine learning (ML) continue to ascend in the financial services space, receiving kudos for their ability to mitigate money laundering, improve risk forecasting and revamp credit decisioning. But these disruptive technologies are not without their flaws, and there have been questions, in particular, about prejudices that may be built into their algorithms.
Unfortunately, it's only when AI and ML go awry that the public seems to notice them the most. Recently, for example, a new credit card offered by Apple (and financed by Goldman Sachs) made waves in the media for all of the wrong reasons.
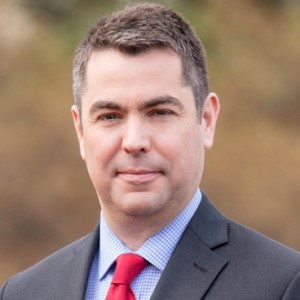
The credit lines offered by this card quickly came under scrutiny for gender bias. The card gave women, in some instances, smaller credit allowances than men. As a result, the AI algorithm it employed was immediately called into question.
Apple and Goldman Sachs attempted to squash any AI concerns about the card by emphasizing that gender was not used as an input variable in the algorithm that enabled the credit lines. The gender bias was instead attributed to “other variables” that were considered, but the damage was already done.
What was lacking, it seems, was proper testing.
During my time working in the mortgage finance space, I was involved in “Fair Lending” testing: Each time a new credit model was being considered for approval, rigorous testing was required to ensure that the new model was no more biased toward certain protected classes (race, gender, age, etc.) than the prior model. Subsequently, to ensure that the results of the initial tests were accurate and could be replicated continuously, a separate, independent body would conduct the same tests.
Indeed, a premium was placed on identifying the potential risks associated with bias and mitigating the potential legal and reputational damage such prejudicial credit decisions could trigger.
If Apple and Goldman Sachs had placed a similar emphasis on testing and re-testing, it's highly likely they would have avoided their credit card incident. In this specific case, the biases that the companies needed to protect against were not detected, partly because they were not included as input variables.
While we don't know the level of resources Apple and Goldman Sachs applied to the credit card's testing, we do know that the effort put forth was subpar - or at least not strategic. Embedding AI into any process requires adopting the right strategy up front, building the proper controls, integrating transparency, and further developing assurances for the process and/or operating model.
Another problem with the Apple/Goldman Sachs case is that we don't know how the code powering the card's algorithm was developed.
For example, was the team that developed the code comprised exclusively of males who weren't able to account for potential bias that wasn't immediately obvious? If so, that's a major issue that should have been addressed at the onset of the card's development. (Indeed, it should have been caught in the testing phase and corrected immediately.) If not, what other detective controls should have been put in place?
Regulation and Transparency
The Equal Credit Opportunity Act (ECOA) has been in place for roughly 36 years, and is intended, in part, to protect against discrimination during credit transactions. In the future, regulatory oversight of disruptive technologies should grow in importance, scope and frequency, particularly on the heels of high-profile, biased credit-underwriting events such as the example above.
Right now, AI and ML algorithms are often described as opaque. To change this perception and improve transparency, three things are needed:
- Clear Proof. First and foremost, firms will need to document and prove that their technology is non-discriminatory.
- Subsequent Verification. Internal audit and compliance divisions will need to staff up to ensure that they have the requisite skill set to probe and ask the right questions during oversight activities.
- External Regulation. Supervisors will need to beef up their abilities to assess compliance, as it relates to bias, adequately.
Undoubtedly, AI and ML are only going to become more prevalent. Simply put, the potential benefits of these disruptive technologies - e.g., better risk forecasting, more effective AML and improved credit underwriting - are too great to ignore.
However, to ensure they do more good than harm requires better testing, enhanced transparency and increased training in AI/ML-based technologies. What's more, to guard against discrimination in credit underwriting and other financial services offerings, internal and external supervisors must step up their game.
Peter Bannister is the SVP of GRC at MetricStream. Prior to joining MetricStream last year, he led the GRC program at Fannie Mae.
Article Updated: November 5, 2021