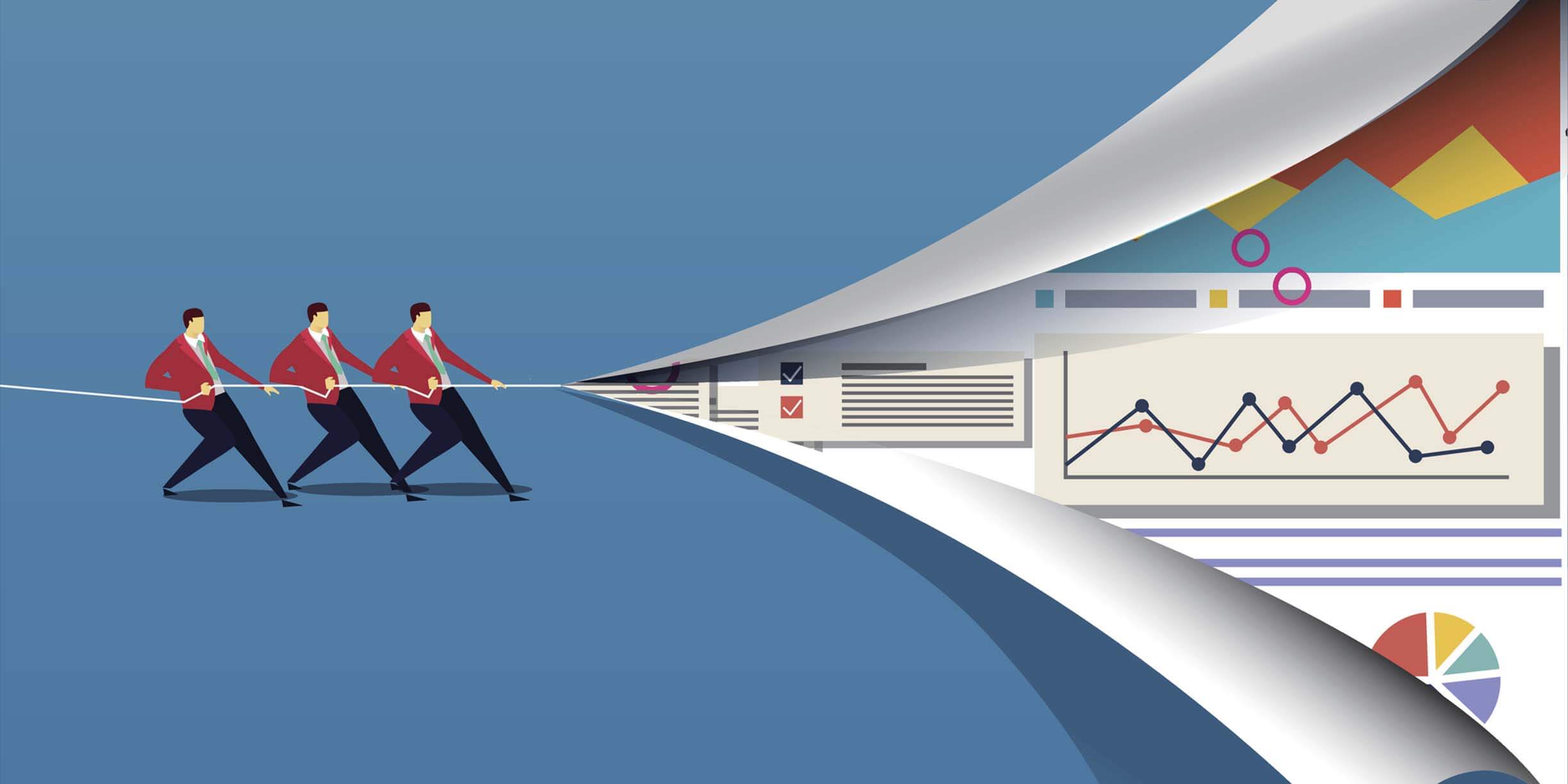
Amid the pandemic, traditional data has failed to provide the timely insights required for drastically-changing behavioral patterns. That's one of the reasons why alternative data has seen substantial growth over the past 10 years and is now all the rage.
But what is alternative data, where does it come from and what specific benefits does it provide? What's the role of technology in the alternative data phenomenon, and what steps can a firm take to maximize its return-on-investment (ROI) from this unconventional information?
Unlike traditional data sources, like financial statements, economic databases, and SEC filings, alternative data can come from almost anywhere - including sources like social media, satellite imagery, news sites, web traffic, and other non-traditional sources.
The main users of alternative data are asset managers and hedge funds, who are always on a lookout for new ways to improve alpha in their performance. There is also a growing number of other users, such as companies seeking real-time data to track their customers' needs.
The result has been an explosion in alternative data services and providers. Indeed, the volume of alternative-data sales has grown to $1.72 billion, with a forecasted compound annual growth rate of more than 50% over the next seven years.
Maximizing ROI, via Selectivity and Technology
Today, there are many different alternative data sources and providers - but it's become increasingly difficult to identify which of them are actually useful. To underline the point, middlemen have sprouted up, for the sole purpose of checking data quality and scoring data providers based on their usefulness.
Most alternative data generated by individuals or sensors tend to be unstructured and difficult to process. Specialized firms can clean and organize the data to make it easier to use. But that's not enough: you also need a matchmaking software that tells you whether the sometimes-expensive alternative data is worth your time and money.
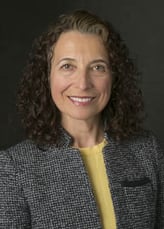
Most of this matchmaking is performed through natural-language-processing (NLP) techniques, matching the needs expressed in a drop-down (or multiple-choice) menu selection with the description of the data.
If you are a hedge fund that simply wants an alpha boost for a particular strategy (like predicting M&As by following corporate jet flight destinations and frequency), this approach is probably sufficient. However, if you are a corporate CFO, a bank CRO, or the CIO of a pension fund, you need more than that. You need to make sure that the proposed data is not just related to your business, in general, but also correlated with your very specific key performance indicators (KPIs).
Alternative data can be compared to food supplements: while it may not be your main source of nutrition, it can be very beneficial, if selected wisely and with the help of an expert. Modern machine-learning techniques can help organizations identify what type of alternative data can be useful - beyond just matching the need and source categories through NLP.
Given enough history, companies can directly connect their revenue and expense segments to mature data sets. For example, as investors have started judging companies for sustainability, everyone has become very focused on environmental, social and governance (ESG) data. Climate data, moreover, is one of the key alternative data types that must be used for estimating one's ESG exposure.
There are no ESG reporting standards yet, so it's important to develop a robust methodology, supported by data evidence that can be adapted to meet multiple requirements. Even if an organization has not yet been significantly impacted by climate change, the effects of climate and ESG risks on KPIs should be communicated to investors and reported to regulators. So, it's important to have a methodology in place.
Connecting with KPIs
A good initial step is to select alternative data sources that can potentially be correlated with your revenues, expenses and other KPIs of interest. Subsequently, you can use regression with regularization to identify which of those KPIS have stable correlations with certain alternative data sources.
These correlations do not necessarily mean causation. But certain drivers of KPIs might have underlying hidden dependencies that are causing correlations between them to increase dramatically - particularly in the tails.
The key to connecting an organization's KPIs to potential alternative data indicators is to identify not just stable historical correlations but also the hidden ones. These hidden correlations cause extreme tail risks that are often called “uncertainty” or “unknown unknowns.”
While these are typically considered impossible to quantify, one can use the full range of scenario analysis to prepare for such outcomes. But this is only feasible if you are estimating their ranges and probabilities, rather than trying (unnecessarily) to be precise in projecting the future values of these outcomes.
Clustering methods connecting KPIs with alternative data can help identify early warning signals and dynamic tail correlations, adding to firms' ability to prepare in advance for adverse outcomes
Impact on Risk-Aware, Long-Term Planning
Alternative data could be used to understand the potential long-term impacts of selected strategies on KPI outcomes.
One cannot, however, foresee the future. So, instead of focusing on the precision of the forecast, strategic application of alternative data should prioritize getting the accurate range of all plausible outcomes. While it is common to claim that uncertainty cannot be quantified, this opinion arises from incomplete generation of distribution outcomes.
Most such distributions are constructed with the standard Monte Carlo simulation with static covariances, throwing in a few stress scenarios to arrive at more dramatic tail results. This doesn't represent the full picture because, in real life, the tail might “wag the dog” - i.e., the tail outcomes that are generated consistently with the rest of the distribution also affect the expected outcomes.
Consequently, the key is to generate scenarios with an augmented Monte Carlo that incorporates shocks, their impacts and further snowball effects - thus simultaneously building realistic tails with consistent probabilities. Considering that traditional data (e.g., SEC filings) is quite “small” with low frequency (monthly or even quarterly), you don't need a lot of history or an abundance of high-frequency alternative data to generate the Monte-Carlo-augmented scenarios with realistic tails.
Asset managers and hedge funds are concerned that their competitors use the same sources of alternative data. In contrast, when strategic decision-makers at these firms use the same sources, they shouldn't be worried.
Indeed, when many firms in the same industry use the same sources for alternative data, it actually creates benchmarks that make it easier for investors and regulators to compare companies on a consistent basis. Moreover, since each company links alternative data to their specific KPIs, the same-source issue doesn't jeopardize a firm's competitive edge.
So, at least in some respects, alternative data requirements for corporate decision-making might be relaxed in comparison to hedge funds' needs for high-frequency trading. Dissimilar to a high-frequency hedge fund trading strategy (which relies on data that must be analyzed instantaneously), one should not be concerned if alternative data processing takes minutes (or even hours) on a corporate level - because the entire strategic planning process takes weeks.
Parting Thoughts
Alternative data is now so mainstream that there are meetups dedicated to debating whether alternative data is still “alternative.”
We often hear that data is the new oil. However, without a proper way to analyze it, we can't use it - i.e., we can't “dig the treasure” out of the ground. If you don't have the tools to analyze the data and derive insights from it, having all of the data in the world is not helpful.
Every firm's goal should be to “turn data into information, and information into insight,” former Hewlett-Packard chief executive officer Carly Fiorina once said. The insight necessary for strategic decision making is very different from the insight needed for the next-minute trade. Alternative and traditional data sources must both be analyzed with this difference in mind.
Alla Gil is co-founder and CEO of Straterix, which provides unique scenario tools for strategic planning and risk management. Prior to forming Straterix, Gil was the global head of Strategic Advisory at Goldman Sachs, Citigroup, and Nomura, where she advised financial institutions and corporations on stress testing, economic capital, ALM, long-term risk projections and optimal capital allocation.
Article Updated: May 20, 2024