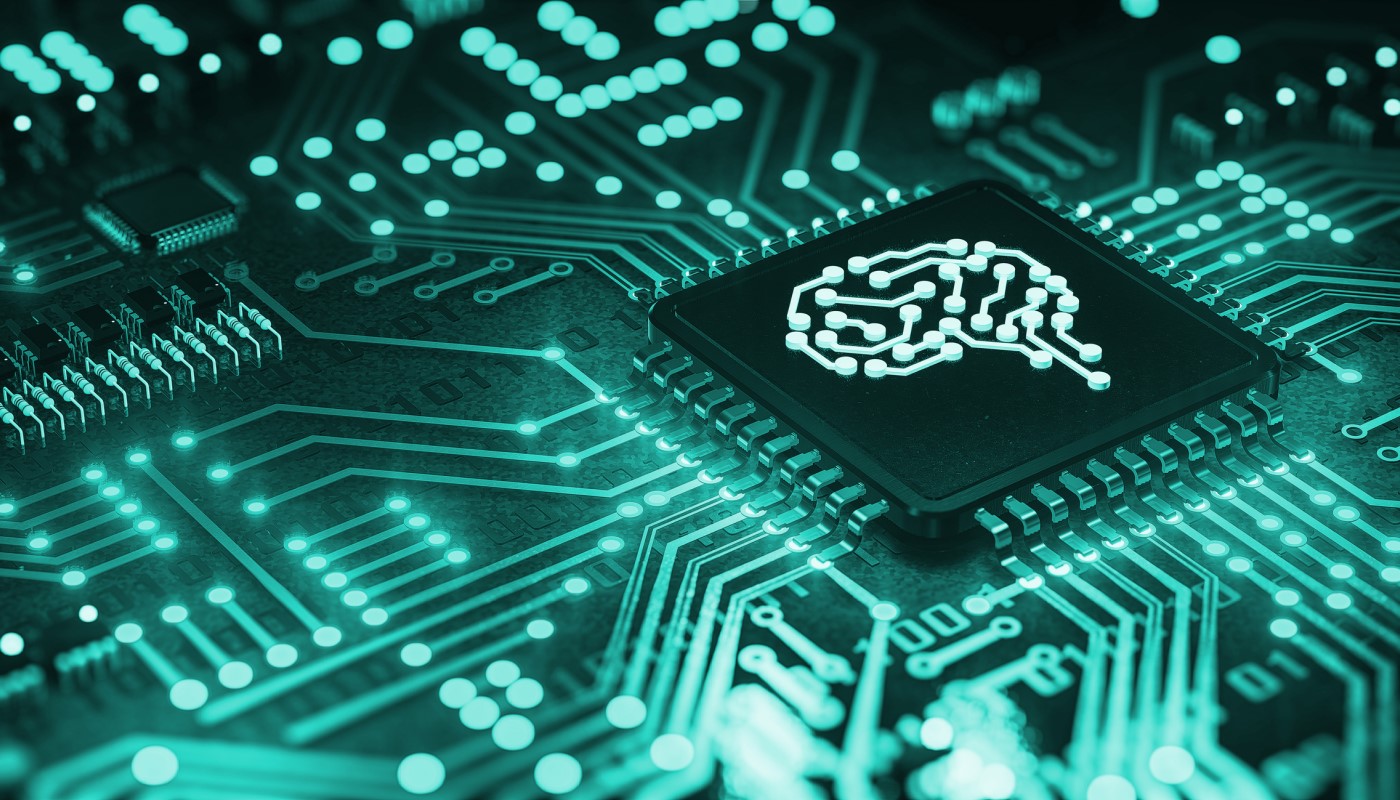
In the financial services community, surprises aren't to everyone's taste. Sometimes, like the pandemic and the lockdowns it caused, they're nasty. Sometimes, like the rapid market recovery prompted by unprecedented government stimulus, they're nice (unless you were short the market).
Looking ahead, we may be in for a third, rather unpleasant, surprise: a surge in defaults, which might finally arrive once the stimulus spigots are closed.
As we seek answers about how to stress test properly for unexpected events, one might ask whether it would have been possible to prepare for these specific scenarios. Considering that the probability of a single scenario in the continuum of all future scenarios is a big, fat zero, the short answer - of course - is no.
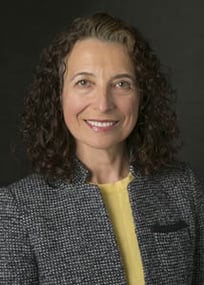
Part of the problem is that history is a poor guide when preparing for unprecedented events, even when scenarios appear similar.
For example, if you wanted to stress test for a tech bubble scenario, the dotcom bust at the turn of the millennium would not make a good match. Similarly, you cannot use the 2008 global financial crisis (GFC) as a scenario for stressing a new financial calamity, because the market environment, investor reaction, regulatory actions and government intervention will all likely be very different during the next crisis.
Indeed, whatever happened in the past will never occur in the exact same fashion again. The tech sector, for instance, is very different now (Facebook is no Pets.com). Banks today, moreover, have much stricter lending limits and hold capital through the cycle.
While the pandemic taught us a lot about lockdowns, any new wave will most likely be quite different. So, preparing for a specific, future scenario would appear to be futile.
But that doesn't mean you shouldn't try. It is, after all, still possible to be better positioned for unprecedented events, as long as you don't insist on a limited number of stress scenarios unfolding in a predetermined way.
Lessons Learned
What, exactly, have previous crises taught us? The first lesson is that instead of starting with a narrative, we should look at the combination of shocks - with their probabilities and severities, and their likely consequences. Subsequently, we should select severe-yet-plausible outcomes, and then build a narrative around that.
Second, what we must learn from the past is the dynamic of relationships: e.g., how different variables change their behavior from stable to stressful market conditions; the range in magnitude of their reaction to shocks; and how variables' correlations change under various shocks, with large probability/small severity, small probability/large severity, etc.
Although no one prior to March 2020 would ever assume a shocking four-fold increase in the unemployment rate (as happened in the U.S.), there were historical precedents of a 2.5x increase over a fairly short period of time.
If we had factored available data into our pre-pandemic scenarios, this would probably have impacted projected credit losses - if they were modeled solely as a function of unemployment. But that would have been the wrong model in the first place, because it would have relied on historical correlations. Moreover, even if the unemployment spike had been underestimated under such a model, more dynamic models would have provided reasonable coverage for actual and potential (but realistic) credit losses.
One positive to take from the pandemic is that banks were undoubtedly much better positioned to weather the stress of COVID-19 than they were prior to the GFC, because they had been performing regular stress testing unrelated to the pandemic for years.
Consequently, while a reasonable pre-pandemic stress scenario would have underestimated the unemployment shock a bit, it would have provided strong coverage of credit and equity shocks. There is also a reasonable probability that the quick market recovery we experienced would have been predicted, because we had at our disposal historical precedents of government intervention.
That said, credit losses could have turned out much worse if governments around the world hadn't intervened so quickly and with such largesse, so risk managers should have been ready for an even worse outcome than the actual one.
Prep Work: The More Scenarios, The Better
Getting ready for a specific doomsday scenario (e.g., a meteorite hitting Earth), and thinking that this covers you for everything else, is the type of approach you need to avoid when stress testing the unprecedented. When you think about it, it's the equivalent of protecting yourself from being struck by lightning and believing this would cover you for a possible stroke or any other potentially deadly situation that requires different mitigation strategies.
The point is, stress testing for the unprecedented requires the full distribution of outcomes. We still can't possibly pinpoint the precise impacts of such events, but we can estimate a ballpark range.
For example, building reasonable models for the subprime mortgage crisis was possible, even though the GFC was unprecedented. Indeed, contrary to popular opinion, the GFC wasn't a black swan, in the sense that it was possible to prepare for it.
At the time, it was clear to most people that there was a housing bubble, and that it could burst at any time. Even if the prices were forecast to fall just 10%, the average homeowner with a 95% loan-to-value ratio would suffer substantial negative equity. Such a hypothetical scenario would have yielded an increased number of defaults, followed by foreclosures, followed by a further drop in house prices.
Furthermore, under this scenario, the increased correlations between defaults would have had a huge impact on structured credit tranches - which would have shifted, overnight, from being AAA-rated to junk.
Keeping all of this in mind, there is a reasonable probability that properly-generated scenarios - built exclusively on pre-GFC data - would have captured proper outcomes. In other words, we could have foreseen the devastating domino effect of subprime mortgages, which would ultimately sap market liquidity and cripple banks.
We can similarly prepare for other unprecedented stresses, as long as we don't insist on giving them exact names and don't demand a precise prediction of how events are going to unfold.
Parting Thoughts
Building up narratives for unprecedented events, and then expecting them to transpire, is totally unrealistic.
When the pandemic struck, everyone expected markets to decline, or at least to remain subdued, for some time. That didn't happen. But it could have happened, and any responsible risk manager should have been ready for the much worse outcome that thankfully failed to materialize.
Stress testing for unprecedented shocks is unavoidable these days. We do it already - via, for example, hypothetical, long-range climate scenarios where very complex dependencies must be estimated without any precedents.
History is all we've got. But if it's analyzed using modern machine-learning techniques, you can build thousands of possible futures, ensuring that you're better prepared for unprecedented, market-altering events.
Alla Gil is co-founder and CEO of Straterix, which provides unique scenario tools for strategic planning and risk management. Prior to forming Straterix, Gil was the global head of Strategic Advisory at Goldman Sachs, Citigroup and Nomura, where she advised financial institutions and corporations on stress testing, economic capital, ALM, long-term risk projections and optimal capital allocation.