Amid the pandemic, as banks rapidly transformed their core businesses to serve their customers, the digitization of banking has given rise to new model risk management challenges.
Decision-making has become more automated with the increased adoption of algorithmic, AI-driven models. But model risk managers have had to vet and validate every new model adopted, adding to the standard model risk management challenges.
Let's now take a look three broad model risk management lessons from the crisis, and their impact on current and future models.
- Traditional models didn't work
The economic disruption caused by COVID-19 had specific implications for measuring, managing and mitigating risk exposures.
Most retail credit models captured extreme movements in the economic time series that occurred in early stages of the pandemic: e.g., collapsing GDP numbers and exploding unemployment levels. These changes propagated throughout risk models, which projected unrealistic loss estimates. Indeed, considering the uneven economic impact of the shutdowns in different regions across the U.S., comparing even the reasonable outcomes of these models across states became difficult.
Traditional credit risk models, moreover, showed limited use in forecasting defaults during the crisis. Part of the problem was that banks had to factor in rapid changes to the lending and economic environment, such as the impact of government-imposed shutdowns and restrictions. Travel, hospitality and transportation were among the industries that were disproportionally impacted.
The ineffectiveness of traditional models is not today's greatest risk management challenge; rather, it's how to adapt alternative models to the changing environment.
While some risk managers believe that the consequences of crises can be best addressed through incrementally-changing models, others prefer bolder approaches - including the development of ensemble models and challenger models.
This varied approach to modeling is particularly evident in the world of automated analytics.
- Automated analytics are a prominent source of model risk
The pandemic forced banks to be more forward-looking and innovative in response to the evolution of customer demand and customer behavior. To effectively deliver digital, customer-driven products and services, banks relied heavily on algorithmic decision-making driven by automated analytics.
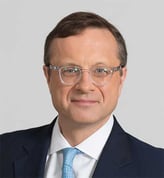
By combining intelligent automation with advanced analytics, banks were able to provide uninterrupted services to their customers amid the pandemic.
However, as banks deployed their automated tools across different segments of their business lines, model risk managers had to validate vastly different uses cases - including KYC processes, robotic process automation and regulatory compliance processes. These digital- and algorithmically-driven workflows carry a slew of compound, interrelated and obscure model risks that needed to be appropriately mapped.
Indeed, model risk managers had to measure the risks of the automated analytics chain - e.g., how was the model built, what data does it use, and how are various predictive factors weighted? They've also had to assess the reasonableness of the estimates that determine decisions, and gain an understanding of how tech-driven operational risks (such as data engineering and machine-learning ops) interact with each other.
As highlighted by automated analytics, the complexity of data and modeling goes beyond the simple engineering challenges. In fact, some of the data that has been fed into these models has proved problematic. Heavy assumptions guiding pre-pandemic practices influenced this data, but behaviors amid the pandemic changed rapidly - carrying tremendous implications for analytic performance.
As the digitization of banking grows, model risk managers will have to manage the model risk embedded in automated analytics.
- Models will only become more complex
While the reliability of automated analytics is likely to improve over time and get even more user-friendly, the underlying modeling will only become more complicated. Model risks will therefore be harder to manage with currently available tools.
Predicting a reasonable spectrum of possible outcomes is challenging - and the more complex the analytical model, the less transparent the model and its results. While explainability tools can help in understanding the workings and the outcomes of models, less powerful models are often preferred for their simplicity.
More complex models increase the likelihood and magnitude of modeling problems: both in the sheer number of model risk failures and the complexity of these failures. Consequently, model risk managers must develop a deeper understanding of the limitations of sophisticated algorithms.
Nowhere is this more pronounced than in the field of artificial intelligence. AI models carry a higher level of risk because of their design complexity and comparative opacity.
In the future, these models will become even more advanced, making them even more difficult to understand, explain and manage. As more complex models are rolled out and more decisions are made by autonomous models, model risk management will evolve into a strategic risk, creating challenges that go beyond simple model risk management.
Increased digitization and more complex modeling will also stimulate greater demand for dedicated risk management talent, who'll need to apply robust practices throughout the model development and deployment cycle.
Looking Ahead
Banking digitization has opened up new concerns for model risk management. While advanced and automated analytics have unquestionably added value during the COVID-19 pandemic, they also have clear limitations.
It is increasingly likely that traditional model validation will change in wake of digitization. Consequently, the role of model risk managers is likely to change, partly to account for more unconventional risks.
The next chapter of model risk management will focus on prioritizing the redevelopment and adjustment of models. Banks, moreover, should expect more supervisory scrutiny and more stringent regulation of their automated models - particularly key models with strategic importance.
Peter Went (PhD, CFA) lectures at Columbia University on disruptive technologies, such as artificial intelligence and machine learning and their impact on financial services and financial risk management.