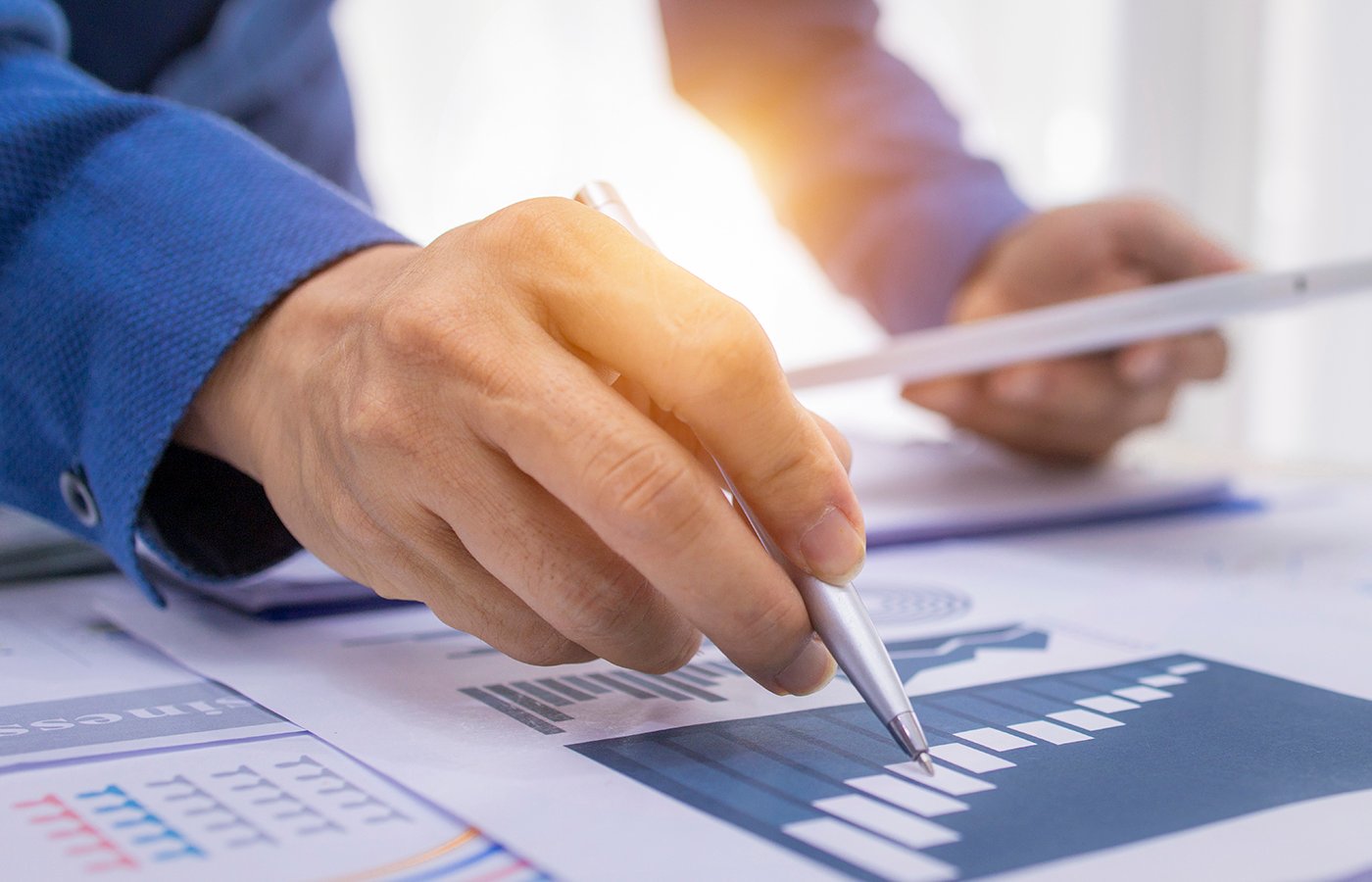
Choice is the root of all evil in statistics. We require bankers to use a single model, which ultimately means that one must be selected. It’s unrealistic, however, to believe that this choice will be made in a completely disinterested, scientific manner.
Just consider, for example, the recent bank failures and everything they have taught us about the current state of risk models and risk culture.
When we sift through the ashes of Silicon Valley Bank (SVB), it becomes clear that management was warned, at least once, of the extreme interest rate risk at the heart of the bank’s business model. It was, in retrospect, a case study in how to lie with statistics.
An April 2 report published by the Washington Post stated that models produced by the bank in 2020 showed that SVB’s cash flow from deposits would fall by more than 27% given a scenario involving rate rises of only 200 basis points. That model was allegedly thrown out by management and replaced; the new projections suggested that cash flow would fall by less than 5% under the rising rates scenario.
“If they see a model they don’t like, they scrap it,” said one former SVB employee quoted in the article.
The situation is slightly more nuanced than it seems. According to the report, the model change “made several mid-level bank officials uncomfortable ... though there was historical data on deposits to support it.”
Hang on a second. If there was historical data to support the new model – the one showing SVB’s deposit base was likely to be robust under a rising rates scenario – why did the original modelers predict an implosion? Given that SVB’s deposit base eventually imploded, what does this say about the relevance of historical data in assessing the risks posed by banks?
Model Selection and Validation Pitfalls
To answer these questions, we need to dig into the vagaries of model selection and how our personal interests impact on the model development process. In the banking industry, regulatory rules and an arcane set of conventions have given rise to a model validation process that can be readily exploited by those with a desire to do so.
For a model to be deemed “valid,” a number of hoops must be successfully navigated. Most validation teams will assess the models for goodness of fit and then apply a number of diagnostic tests that must be passed for the model to be deemed acceptable. The models must “make sense” from an intuitive standpoint, and the process used to assess this is necessarily fairly subjective.
The problem is that a lot of different models built on a wide range of behavioral assumptions can all claim to be “sensible.” In terms of the relationship between deposits and interest rates, some of these specifications will indicate a relatively weak correlation and some will suggest something much stronger. But according to the established validation process, they are all perfectly acceptable.
Tony Hughes
With a number of valid model projections available (well, they're available implicitly, someone still has to build them), it is a simple matter to choose your favorite. A neutral outsider would make a fairly moderate choice, while an insider whose bonus was at stake would probably choose something bolder. A conservative investor, meanwhile, would want management to use a model very much on the pessimistic side of things.
I have no doubt that both of the aforementioned models proposed by SVB staff were capable of passing current model validation protocols.
The solution to this dilemma, obviously, is to jettison the requirement that a single model must be used. This has been a point of discussion for many years in the industry. Most senior modelers are aware that modern model averaging techniques are far superior to methods that require a single model to be plucked from the huge set of reasonable alternatives.
As a thought exercise, imagine for a moment that the full set of models capable of passing validation was available to SVB stakeholders in 2020. If, say, 90% of these models indicated that a 200 basis point jump in interest rates would kill the bank, it would be difficult for management to claim that their optimistic forecast was appropriate. On the other hand, if this was a feature of only 20% of the models, it would be much easier for management to make their case.
However, even if 99% of the models suggested that the bank was safe, I’d still be interested in the findings from the 1%. If a single model predicts disaster – so long as it is empirically supported and broadly sensible – then it cannot be completely discounted from a proper assessment of risk.
Realistic Reform
Given current rules, it’s unrealistic to imagine a risk team building hundreds of independent models of every feature of the bank. Such an approach would only be practical if regulators dropped the requirement that every individual specification be subject to a detailed validation and documentation process. The alternative would be to validate the entire process as a single, coherent exercise.
If we stick with small numbers of models, more modest reforms are also possible.
Traditionally, banks have been encouraged to build champions and challengers, but the SVB experience suggests that a preferred and a pessimistic model should instead be required. It will be difficult to police this process, because there will be a strong incentive for banks to use the most optimistic pessimistic model they can possibly get away with.
But if a bank could demonstrate soundness with a truly downbeat set of models in the background, it would give interested observers heart that the bank was truly sound.
Ideally, banks should implement what I describe as a "whole menu" approach: running many different models and then constructing an average of the results, with higher weight given to better models. This suggestion, however, would require a change in the way risk models must be documented and validated. The second best solution is what I like to call the "spinach" – forcing banks to find and use pessimistic model specifications.
The core problem at SVB was that management were able to choose their models from an unseen menu of many alternatives. To avoid a repeat, banks could either sample the whole menu or be forced to stick with the spinach.
From an analytical perspective, the whole menu approach is by far the most nutritious.
Tony Hughes is an expert risk modeler. He has more than 20 years of experience as a senior risk professional in North America, Europe and Australia, specializing in model risk management, model build/validation and quantitative climate risk solutions.
Topics: Model Risk