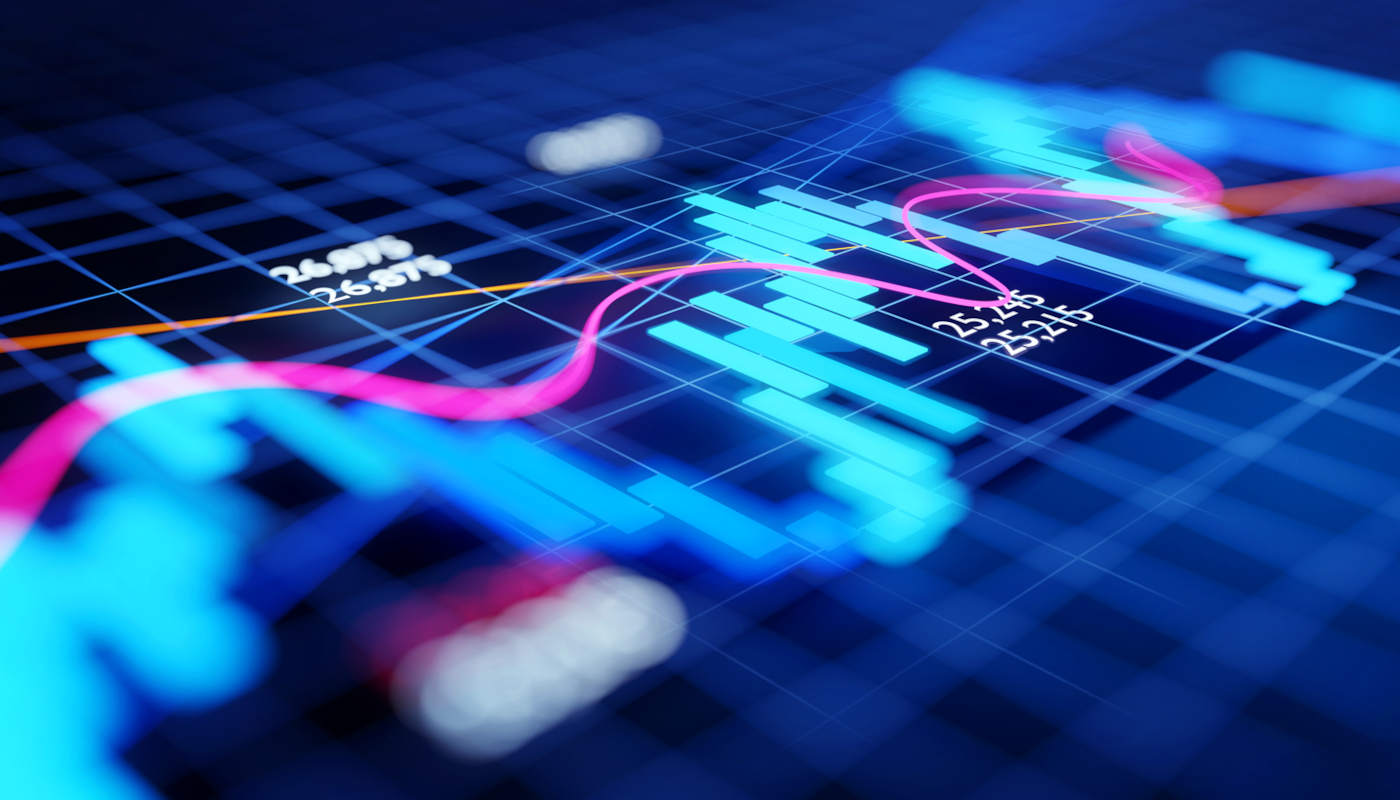
The current COVID-19 crisis is leading to questions about the accuracy and efficacy of value-at-risk (VaR) as a risk management tool and as an input to capital computation.
VaR measures the maximum loss in the value of a portfolio over a predetermined time period for a given confidence interval. Using a probability of 99% percent and a holding period of 10 days, an entity's VaR is the loss that is expected to be exceeded (with a probability of only 1%) during the next 10-day period.
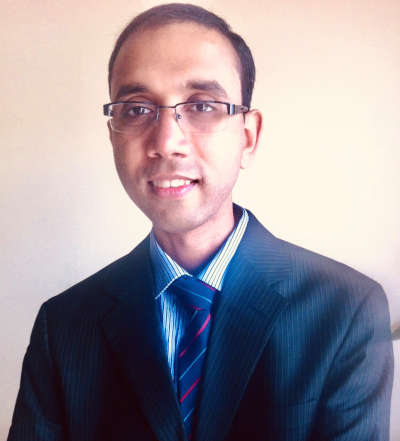
The pandemic has caused an extreme downfall in global markets, leading to several banks breaching their VaR. Indeed, in recent fillings, HSBC reported 15 VAR back-testing breaches during January-March 2020 period, while BNP Paribas reported nine such incidents for the same period. Furthermore, other large banks, including ABN Amro, Deutsche Bank and UBS, have also reported back-testing outliers.
The issue here is that VaR is calculated using the historical data, and assumes that the future will follow the same pattern as the past. Hence, it is backward looking. Moreover, it is also procyclical, which means that before a crisis, when higher capital is required, VaR is typically under-estimated and, hence, banks' market risk capital requirements are low.
In the same way, when a crisis has passed and banks are required to free up capital to inject liquidity in the market, we see high VaR numbers and a high capital requirement. This can be clearly seen in Figure 1 (below), where we have shown the VaR and actual P&Ls of a hypothetical portfolio consisting of S&P 500 basket.
Figure 1: VaR and P&L for a Hypothetical Portfolio
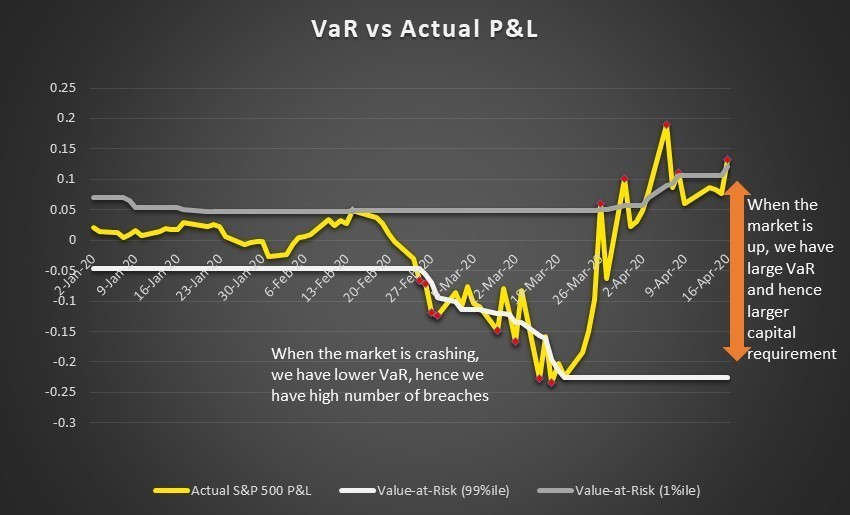
As depicted in Figure 1, we can see that when the market crashed in March 2020, VaR was lower. Later, after the market has started to recover, VaR is very high. The graph also depicts multiple breaches during March, when P&L losses were higher than VaR.
Risk managers have clearly become over-reliant on VaR, partly because they seem to be lulled into a false security when the metric remains within its limits. However, while keeping their portfolios within VaR limits, traders can still take excessive (but remote) risks.
Currently, there are three approaches to VaR computation: historical simulation, Monte Carlo simulation and the parametric approach. The historical simulation method is the most favored method within banks, while the parametric approach is rarely used.
In the historical simulation method, VaR is computed using returns for the past 252 days. In Monte Carlo simulation method, instead of using the historical data, asset returns are generated using an underlying stochastic model - e.g., Geometric Brownian Motion (GBM) is used to forecast stock prices. However, the parameters of these processes are calibrated using historical data only.
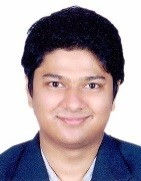
That's why, unfortunately, none of the existing VaR approaches capture tail risk. For example, during the COVID-19 pandemic, trading losses at the U.S. units of Deutsche, RBC exceeded VaR by 1,000%.
To check accuracy of VaR models, banks must perform back testing to compare calculated VaR with actual P&L movement. The number of breaches allowed depends on the confidence interval assumed while computing VaR. When VaR is computed at 99%, it is typical for a firm to experience no more than three breaches per year. Any firm that experiences more VaR breaches than expected will receive a capital penalty, per the Basel Committee on Banking Supervision's (BCBS) traffic-light approach.
Regulatory Approaches, and the Impact of VaR on Market Risk Capital
VaR is not only used to set trading limits but also to determine a firm's market risk capital requirements. It is, in short, an essential tool used across banks.
Regulators' approach toward the shortcomings of VaR have traditionally been reactive rather than proactive. After 2008 recession, for example, the BCBS introduced stressed VaR (SVAR), upon learning that many banks fell short of their required capital. The idea behind SVAR was that, under stressed conditions, banks may require more capital, and such capital requirements aren't fully captured in normal VaR calculations.
However, when a market collapses, banks' VaR numbers increase to the level of SVAR to reflect the losses during a crisis (see Figure 2) - creating an issue of double counting.
Figure 2: VaR and SVAR of a Hypothetical Portfolio
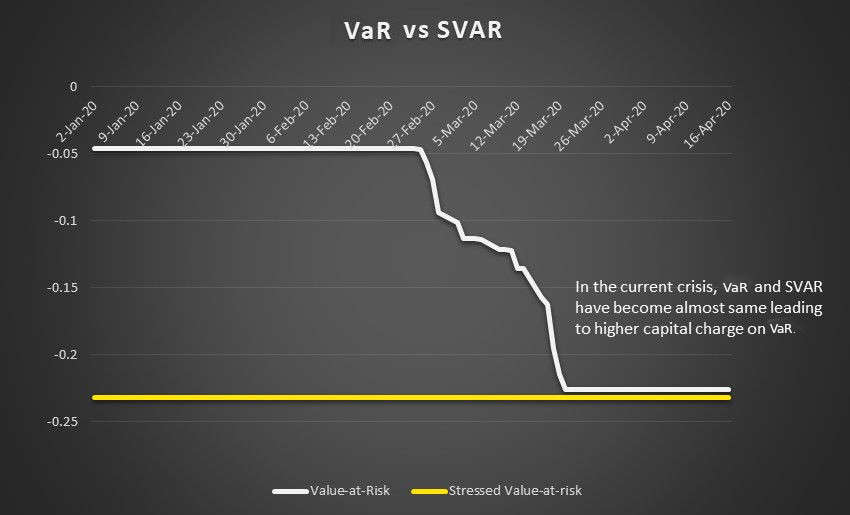
Typically, more back-testing breaches are observed during a crisis, increasing the multiplier and leading to additional capital requirements. For example, due to excess breaches observed by ABN Amro in the first quarter of 2020, its capital multiplier increased from 3x to 3.5x. As a result, its capital charge was 57% higher than three months prior.
This stopgap method for calculating market risk-weighted assets was first outlined in the in Basel 2.5 accord, and is supposed to be remediated by the scheduled 2022 introduction of the BCBS' fundamental review of the trading book (FRTB) framework. At that time, the FRTB is expected to replace VaR with expected shortfall (ES) - a risk measure that gives more weight to the losses in tail events, and is calibrated to the worst year of a bank's portfolio.
ES does not have a double-counting issue, and therefore is expected to reduce capital volatility during a crisis. On the other hand, one major disadvantage of ES is that it is difficult to back test.
Therefore, even under the FRTB, banks' risk models will still be back-tested using VaR. So, while FRTB approach to capital computation would solve the dilemma of double-counting, VaR's backward‐looking and procyclical approach will continue to pose a problem.
The recent increase in VaR breaches is also problematic on another level. In the FRTB framework, higher breaches in VaR require banks to switch to standardized approach (SA) from an internal modeling approach (IMA) for the calculation of risk-weighted assets (RWA). This could lead to higher capital requirements for banks, because the SA approach incurs a higher capital charge.
Potential Forward-Looking Solutions
To make VaR responsive, an exponentially weighted moving average (EWMA) approach can be used. Though this will increase the weight given to recent events, it still does not incorporate truly forward-looking events.
To overcome procyclical and backward-looking nature of current methodology, one must first enhance the current approach of historical VaR computation, and then add a forward-looking element.
Figure 3: Improving VaR - A Two-Step Process
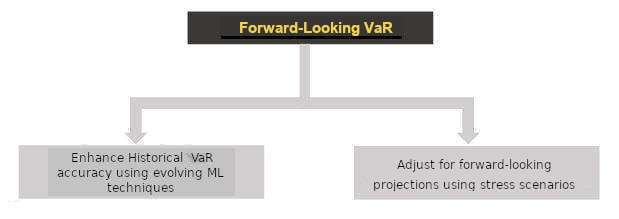
Let's now take a closer look at each step:
Enhancing Historical VaR Accuracy
Historical VaR uses data from more than one year ago to predict the worst loss on any day. However, as market conditions change, even one-month-old data may not accurately represent these recent conditions. We therefore need to make the VaR model more responsive by reducing its time horizon. Even with this change, however, a very small number of data points may not give statistically-reliable estimates.
Technology may offer a solution to this problem. Data scientists believe that advanced ML techniques (like neural networks) can capture more statistical parameters than are captured by traditional financial modeling techniques. ML-driven models incorporate fat tails closer to reality than assumed by normal distributions - or that may not be reflected in a given historical period.
The challenge here is to train such innovative models, which can require a large amount (e.g. 5,000 data points) of data. Diving that deep for financial data may not be possible, and may not prove extremely useful, given the constantly evolving nature of markets.
To meet these challenges - i.e., to more effectively train the ML models and to compute more accurate VaR - financial institutions can use synthetic data. This type of data can be generated via classical ML techniques, like support-vector machines and random forests, and more advanced techniques, such as Restricted Boltzmann machines.
Data generators give banks an additional advantage of populating time series data for new or illiquid securities.
Adjust Historical VaR for Forward-Looking Scenarios
The Federal Reserve Bank's CCAR stress test can serve as the inspiration for firms that want or need to integrate forward-looking scenarios into their VaR models. As part of CCAR, Fed comes up with projections of 28 macroeconomic variables for three scenarios: baseline, adverse, severely adverse.
Similarly, a bank's economic research team can develop forward-looking projections for a variety of macro-economic variables for different scenarios. The bank can then use its existing stress testing infrastructure to expand the shocks at a more granular level and compute the P&L impact on its existing portfolio.
Subsequently, banks will produce four numbers: one for historical VaR (computed using the aforementioned ML approach) and three P&L impacts corresponding to each scenario. Banks can then assign a suitable weight to each scenario, and come up with a weighted average P&L impact. Weights under this approach need to reflect the sentiment and extent of stress possible in the near future.
The basis for this strategy comes from the computation of expected credit loss (ECL), where banks first compute ECL numbers for base, best and worst scenarios. The final ECL impact should be computed as the weighted average of the impact from each scenario.
Parting Thoughts
Every crisis in the past has brought disruption - not only in people's lives but also in the ways we think. The disruption leads to thinking outside the box and questioning the status quo - and COVID-19 is following a similar path.
Recent market trends have shown that we have failed to mitigate all the weaknesses underlying widely-used risk metrics like VaR. Particularly with respect to market risk capital calculation, regulators globally need to reevaluate approaches to VaR. The goal should be to better align this outdated metric with the underlying risks of a bank's portfolio.
The eye-opening COVID-19 crisis has demonstrated the strong need for firms to incorporate forward-looking views in VaR computation. The metric should ideally play the role of a fortune teller, rather than a news anchor.
Subrahmanyam Oruganti is a partner at Ernst & Young India. He has more than 14 years of experience in financial services. Currently, he leads the Quantitative Advisory Services within EY India, focusing on risk management quantitative solutions like model development and validation, and newer regulatory programs like CCAR/FRTB/IBOR/IFRS 9.
Yashendra Tayal is a senior manager at Ernst & Young India. He has roughly nine years of experience in financial services, working extensively on market and liquidity risk, model development/validation, derivatives pricing, regulatory submissions, risk measurement tools (like VaR and stress testing), scenario expansion and counterparty risk. Currently, he is part of the Quantitative Advisory Services within EY India, focusing on risk management quantitative solutions.
Article Updated: November 17, 2021