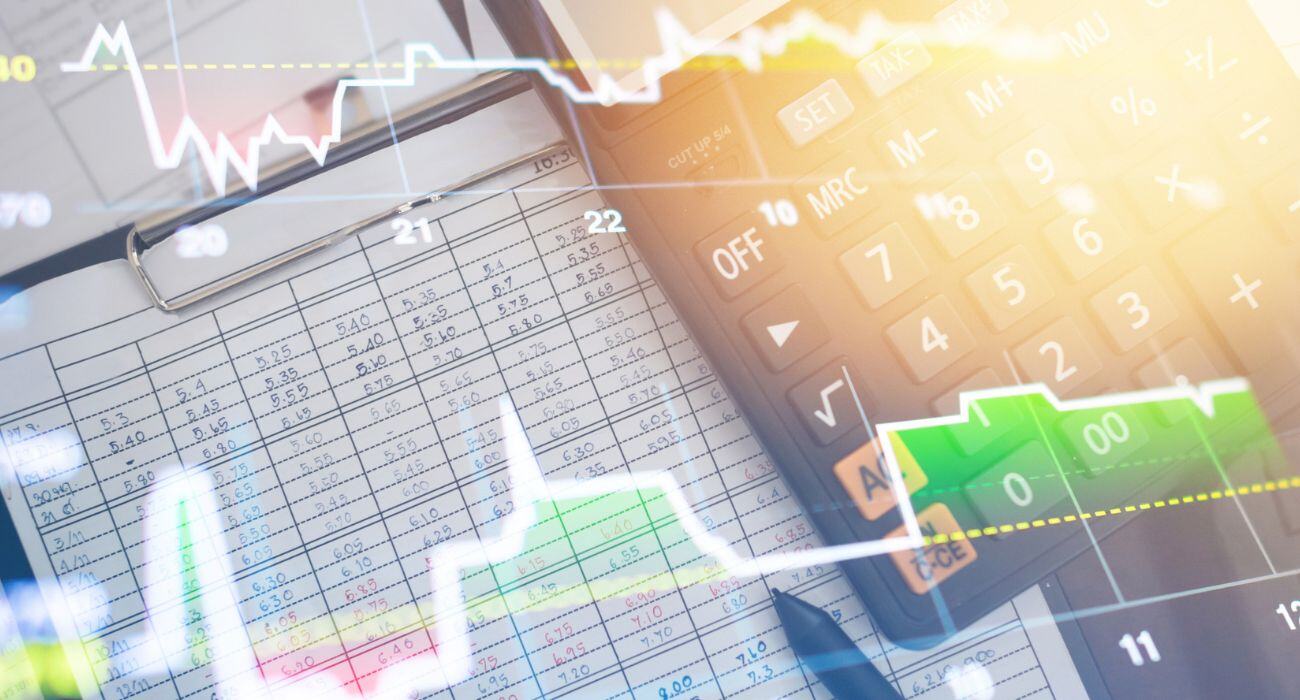
Over half a century has passed since electronic communication networks (ECNs) disrupted the traditional floor-based model of stock exchanges and ushered in the era of electronic trading. Since Regulation NMS (National Market System) was adopted almost 20 years ago, the honorable goal of promoting venue-by-venue competition and fair price formation across securities markets turned into a latency arms race with rising market data and connectivity costs.
In the quest to gain an edge over competitors, trading venues may offer market-making rebates, introduce a speed bump, proliferate order types, come up with new business models (e.g. market-on-close) and create other privileges (e.g. exclusive access to certain pegging orders). There is jockeying to make money by altering the queuing and wait times at the “checkout counters.” This has widened the gap between haves and have-nots.
It is a fitting moment to reflect on the evolution of capital markets. Several trends are emerging that could push today's market structure from a focus on latency to one driven by algorithmic and artificial intelligence (AI) technologies.
HFTs Into Outsourced Execution
A decade ago, then-SEC Chair Mary Jo White famously said, “Deemphasize speed as a key to trading success.”
Whether it is regulatory inaction, or the current administration’s proposing a questionable prescription, market participants today still find it hard to beat the high-frequency trading (HFT) firms. So long as the Consolidated Tape is not a reasonable compromise, if not a close substitute of exchanges’ proprietary feeds, everyone is and will continue be subservient to telecom infrastructure vendors.
Kelvin To of Data Boiler Technologies: AI as paradigm shift.
There is not enough alpha for the HFTs to justify the costs and benefits in just doing proprietary trading. Thus, HFTs get into the business of outsourced trading execution services. With stock exchanges optimally restricting access to price information by exploiting the inelasticity of demand of proprietary products, many choose to collaborate with the haves for outsourced execution, rather than compete.
This collaboration aims to maximize/segment order flow for negotiation of tier rebates and other incentives that pose potential conflicts of interest and best-execution issues. The latency arms race would subside only if time-lock encryption is adopted to protect time-sensitive data from being decrypted prematurely.
Power and Rebellion
The voluntary collaboration among the outsourcers and HFTs further complicates the markets. Aside from using transaction cost analyzers, liquidity sourcing and other tools amid fragmented markets, market participants are increasingly leveraging technologies such as AI, algo wheels and quant models to stay afloat.
The 20th century saw the start of a “cyber punk” era. Elite corporate messaging conflicted with individual independent thinking. So the rebellious mobilized in online communities. This was exemplified by the meme stock phenomenon, advancing a controversial agenda.
There are digital nomads, infusing “trust” into digital assets on related financial infrastructures. With foreign adversaries looking at “unhealthy” competition as a way to erode the U.S.'s prominent market position, many do not seem to realize the potential threat against capitalism. DeFi (decentralized finance) and de-dollarization movements are on the rise and stand to reap benefits out of chaos. Witnessing this dynamic, market participants would need their algos and AI to guide them in discerning who is who and the market impacts.
One Size Does Not Fit All
Different trading venues (lit exchanges, dark pools, systematic internalizers, single-dealer platforms) are like different streaming platforms providing various contents (e.g. block trading, exotic, passive, conditional) that fit the appetite of respective subscribers. While we are generally supportive of standardization and harmonization of regimes, policymakers should be warned that open access and interoperability could inadvertently be a path to monopoly or reinforce the elites’ oligopoly that hurts fair competition.
SEC Commissioner Hester Peirce has said, with respect to implementing the Financial Data Transparency Act, “Hardwiring a technology into a rule runs the risk of preserving that requirement far after that technology’s expiration date.”
Moreover, no matter how Data Expert Group(s) are working with regulatory authorities in calibrating the consolidated tapes, reference price arbitrage is inevitable when there is more than one de facto best bid and offer under a competing consolidators model. If the public markets opt to serve only the most liquid and static form of investments, the private markets will prevail for their flexibility to dynamically customize deals for the investing communities.
“Too Much Data”
We agree that automation historically benefited the elites in achieving economies of scale. However, federated learning and real-time analytic platforms are more efficient and effective in providing timely decisions and rapid response in modern dynamic markets.
Today, many resources are devoted to, or wasted in, sourcing alternate data. Too much data and inherent problems (timestamp synchronization) of data imprecision cause centralized intelligence to take forever to achieve a “golden source.” Valuable insights may inadvertently be removed from the dataset.
Non-developers are now able to use no- or low-code generation with AI/large language models (LLMs) to do some ad hoc tasks, rather than relying on the development team. Whereas the development team uses these tools to take on more complex projects, concentrate on quant modeling, reverse engineering of others’ algorithms, better Boolean logic, taxonomy etc.
Volatility, Hallucinations, and Music & Trading
Trading teams are poking holes in the initial results of AI, which they are right to do because there are different machine learning models. Firms should not go blindly with neural network, deep learning black boxes, then go about craving after ever more data to feed the models.
While extraordinary market volatility and suspicions of AI hallucinations persist, there is also a growing recognition of the opportunities presented by newfound signals and liquidity amid chaos.
Machine-learning performance will improve over time. It may discover unknown and hidden opportunities which were previously nonsensical to humans. It is a paradigm shift from a latency-driven capital market to one powered by algorithmic and AI/machine learning technologies.
Be open-minded to explore the surprising similarities between music and trading: The sound that data makes is well suited to time series.
Kelvin To (kelvin.to@databoiler.com) is a big data and financial technology platform innovator and founder and president of Data Boiler Technologies.
Topics: Financial Markets