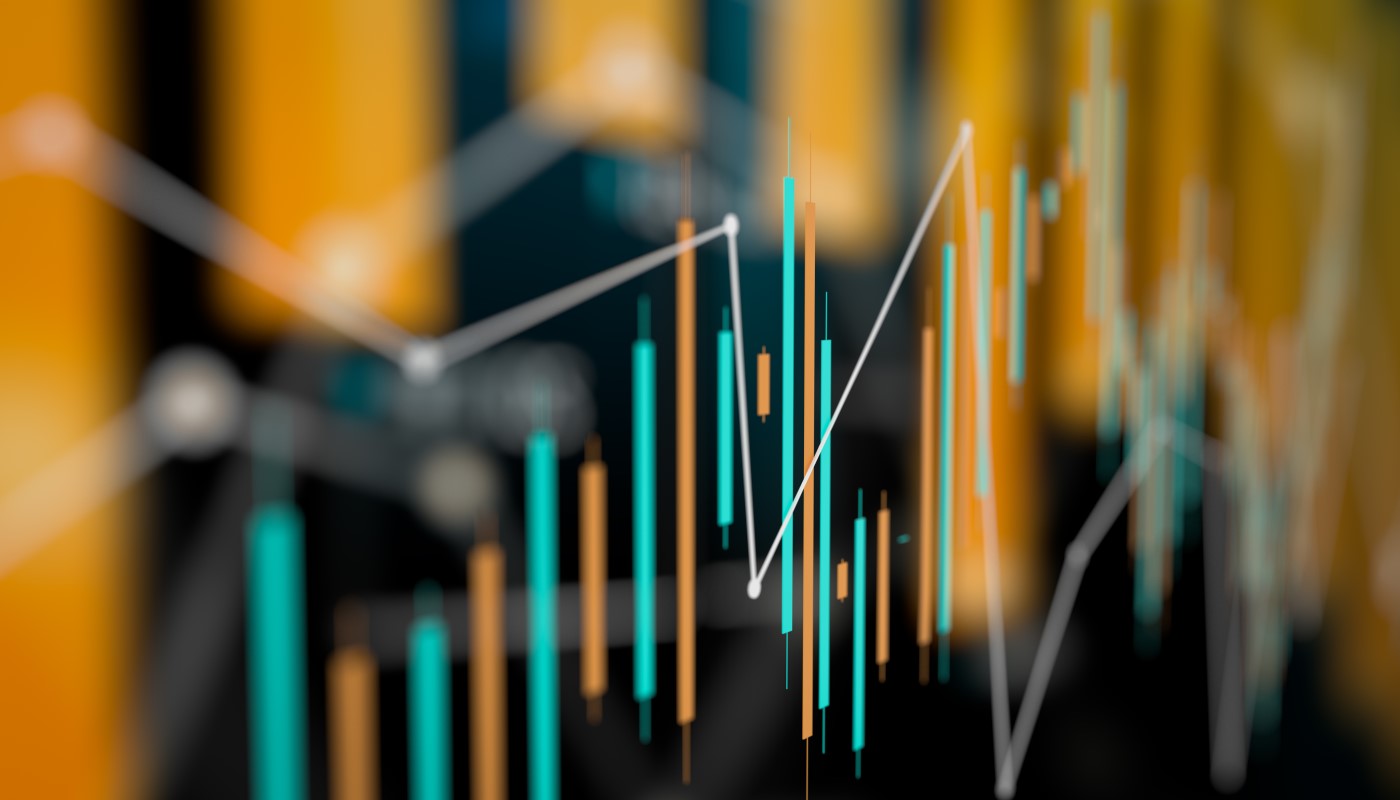
Last month, the European Central Bank published the results of its monumental TRIM project - a detailed five-year exercise to assess the internal models used by large banks to determine risk weights and regulatory capital charges.
Based on the findings, regulators identified a number of institutions they considered to be excessively optimistic in their loss assessments. These banks were asked to respecify their models and also, more importantly, to set aside a considerable amount of additional capital.
There are many other examples of model benchmarking exercises initiated by regulators. For example, IFRS 9 models and the projections they produce are currently facing scrutiny in various jurisdictions around the world.
In benchmarking exercises, regulators are trying to apply a set of common standards across a very disparate and heterogeneous industry. You can immediately understand why this is desirable: when calculating capital or loan loss reserves, banks have a clear incentive to downplay the riskiness of their exposures, and complex statistical models provide an obvious way to camouflage excess risk.
Benefits and Detriments of Model Regulation
Exercises like TRIM provide critical oversight of industry behavior, ensuring that banks play by the rules on a broadly level playing field. With that said, it's important not to lose sight of the potential downside to these types of regulatory interactions.
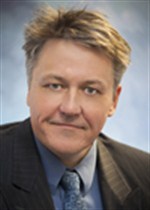
What might be some of the consequences if overzealous benchmarking were to lead to excessive model homogeneity within our industry? Exploring the difficulties faced by regulators during the TRIM project will allow us to highlight some of the real benefits of model heterogeneity across banks.
If regulators can adequately control for interbank differences in risk appetite, underwriting skill and loss mitigation efforts, a true apples-to-apples model comparison will begin to materialize. One bank, for example, may have an active forbearance program while another uses a broader set of attributes to score prospective loans at acquisition. However, it's very difficult to tell whether the institution with the lower loss projection is being overly optimistic in their modeling efforts or whether they actually do hold an advantage over their competitors when originating and servicing loans.
There are so many qualitative factors that must be considered when comparing models across banks. For example, since every institution has a unique history, the internal database available to the banks' respective modeling teams will be distinct, even if current portfolios are broadly comparable. These differing histories will impact the observed dynamics of the portfolio, causing divergent projections for seemingly similar portfolios.
As modelers, we know that comparing specifications from the same database is sometimes very difficult; industry benchmarking projects, which instead seek to compare models built using completely different databases sourced from different underlying populations, face an even more challenging task.
What should be clear from this discussion is that the very best models will normally be built by insiders who can tailor their efforts to the specific vagaries of the institutions they are purporting to model.
Regulators, meanwhile, must seek to gain sufficient mastery of each bank's models to enable them to make reasonable interbank comparisons. This is the main reason model benchmarking is such a laborious and time-consuming exercise - and it is why the TRIM project took five years to complete.
Innovation in Risk Modeling
Now suppose that a pair of banks happen to have identical risk profiles and are equally adept at managing at-risk clients. One of these banks then sets out to overhaul their loss forecasting and stress testing models, developing new data sources and innovative methodologies that dramatically decrease the width of underlying prediction intervals. They have the work independently vetted and the gains are found to be real and robust.
Should the bank then enjoy lower capital than their less innovative but otherwise identical peer?
Capital is meant to be proportionate to risk, and since improved modeling tools reduce the uncertainty inherent in future loss projections, the innovative bank should be duly rewarded for their creative efforts.
Now suppose the two banks enter a new round of model benchmarking. In this situation, there will be a heavy onus on the innovative bank to clearly document and explain their new methodology. There will then be an equal burden, moreover, on the regulator to understand exactly what the bank did and why it justifies a reduction in risk weighting.
To a casual observer, it would appear that the innovative bank is simply trying to reduce its perceived riskiness, using fancy stats to hide the evidence. Fearing the casual response, many banks do not want to risk the admonishment of their regulator, and will thus shelve plans for innovative model rejuvenation in favor of more mundane options.
This is one of the unavoidable downsides of benchmarking and industry standards enforcement - that the incentive to innovate is inevitably curtailed as a direct consequence of regulatory intervention.
It should be noted that these forces could also potentially stifle competition for new lending.
Suppose that, under the standard modeling tools, a prospective borrower has an estimated PD of 20%. A bank, though, has developed a new model that uses a broader set of risk drivers, allowing them to see that the default probability is actually around 5%. Under these circumstances, the capital reserves based on the standard model would make the loan unprofitable, disappointing the worthy prospective borrower and curtailing industry growth.
Parting Thoughts
After controlling for risk appetite, banks with lower-than-average loss estimates fall into two separate groups. One group we can describe as 'cheats,' because they use model complexity to lower perceived - but not actual - levels of risk. The other group can be described as `geniuses,' because they discover ways to actually reduce realized losses for a given amount of underlying risk.
Society wants to eradicate the cheats and actively encourage the actions of the geniuses.
The problem is that it is almost impossible to tell the two groups apart. What's more, in attempting to suppress the cheats, regulators may instead be curtailing innovation in risk management techniques that hold the potential to reduce borrowing costs for consumers and enhance credit availability for underserved populations.
A truth we've all known since elementary school is thus revealed: cheating, whether successful or not, inevitably spoils the game for absolutely everybody.
Tony Hughes is an expert risk modeler for Grant Thornton in London, UK. His team specializes in model risk management, model build/validation and quantitative climate risk solutions. He has extensive experience as a senior risk professional in North America, Europe and Australia.