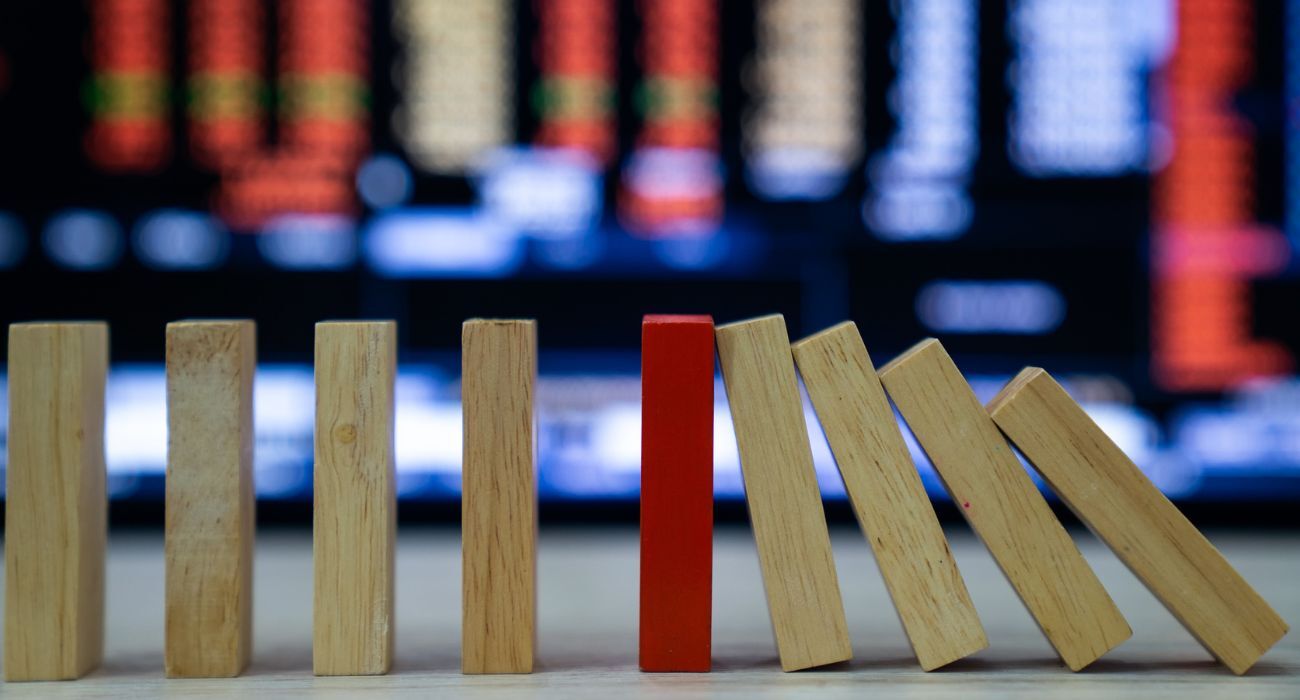
Whenever a bank considers the construction or purchase of a new model, the first decision they must take is always, “should we or shouldn’t we?” This decision, which is not well covered by existing model risk management practices, actually carries quite a bit of risk for a bank.
Tony Hughes
To illustrate, consider two hypothetical banks with the same target market, business model and broad attitude to risk.
The first bank has a crack team of bankers who assiduously assess every application for credit. It provides staff with a deep data lake but chooses not to employ any statisticians. Its philosophy is that human intuition is more relevant than statistical models when making critical business decisions.
The second bank also has a top-notch team of business professionals and a similarly extensive range of data assets. The difference is that this bank has a talented group of analysts tasked with producing quality models, the output from which is fed to the bankers. The underwriters have the authority to override the forecasts and credit scores produced by the data science team, but only when there are compelling reasons for doing so.
This comparison is somewhat artificial (I’m assuming my example banks are unregulated), but it highlights a key point about the use of analytics in our industry. Thoughtful modeling and its careful application is – always and everywhere – risk reducing.
An expert banker with a good model will always make decisions that are at least as good as the lender that lacks such tools. The model must only be helpful some of the time for this to be true.
Modeling vs. 'Brain Chemistry Regression'
When weighing the merits of modeling versus human intuition, we need to be careful when defining what we mean by a “model.” Regulators and banks use a tight definition – a model is something that can be coded up and run on a computer.
But when humans make decisions, informal models are constructed in our brains. A person will weigh various factors in their minds, discounting some variables while giving considerable weight to others. You could call this process a “brain chemistry regression” if you were so inclined.
Sometimes these informal models are wise and insightful; too often they are fanciful and biased. They rely on human judgement to forecast an outcome and then take action.
One key difference between human and artificial intelligence is that, at least with the latter, it’s always possible to inspect the code and amend it if a prejudice is identified. AI processes can be documented, even if they are highly complex; the human brain cannot be mapped as easily or as precisely.
In this sense, the two hypothetical banks both use models. If you were to argue that the second bank is just as risky as the first, you would have to explain why an undocumented, unstructured, possibly haphazard use of data is somehow less volatile than a systematic, rigorous approach that can be documented and reproduced.
Are Bad Models Worse Than No Models?
For the rest of the article, we will stick to the standard industry definition of “model.”
There’s no doubt that bad models carry more risk than good models. After all, considerable attention is paid by modern banks and regulators to the potential for financial losses stemming from the use of poor-quality analytics.
But what about the threat posed by not using a model? Why isn’t this managed in the same way, with the same diligence?
If a bad model is better than nothing, the whole field of model risk management may be assiduously controlling the second-order risk while ignoring the bigger problem. (Of course, I’m not talking about situations where banks are obliged to maintain models, perhaps for capital allocation or loss reserve calculations.)
In answering the above questions, we must be a little clearer about what we mean by a “bad” model. Models could be ineffective because of circumstance, sloppiness or sabotage.
In some cases, the model looks bad because the data are very difficult to model. You may be in a situation where observed losses are very lumpy or where there is only a small amount of relevant data available.
A good example of this would be loss given default (LGD) modeling for large commercial loans. Another example would be situations where the data being modeled appear to be irrelevant due to changed market conditions, much like the way models were perceived to fail during the COVID-19 pandemic.
If the models are failing due to circumstance, I would argue that they should still be built and maintained to the highest possible standard. The reason for the failure may provide clues about possible portfolio performance. Even though the model might not be especially trustworthy, it still provides a baseline view from which subjective overlays can be applied.
It is also important to keep in mind that modeling is most helpful when it’s difficult to model! Making easy predictions never adds a lot of value to any organization.
When I reference “sloppy” models, I’m talking about situations where the modeling team is not biased in any particular direction, but is just not very good at performing their duties. They may lack expertise, experience or proper organization, to the point where the models being produced are in some way suboptimal. This includes situations where models are poorly documented, meaning that high-quality output may be incorrectly used by the non-quants at the coalface.
Again, I would argue that in many cases, building and using sloppy models is better than doing nothing. The signal may be weak, but it is still a signal. If bad models are well documented, the managers in the field can make an informed decision and decline the use of the models if they are just too untrustworthy.
There are, according to Mark Twain and Benjamin Disraeli, lies, damn lies and statistics. If the results of a modeling exercise are in any way prejudged or if modelers are incentivized or instructed to produce a certain outcome, I would describe this as a form of sabotage.
The key point here is that if the model is a lie, deliberately obscuring the signal from the data, it is unquestionably harmful. In this situation, it would undoubtedly be better to not have a model.
Parting Thoughts
In many situations, banks are required or expected to build models. In these cases, the standard principles of model risk management apply – good models are always better than bad models. These principles also increasingly apply to situations where banks are free to choose whether they want to use a formal model or to rely on the brain chemistry of their trusted managers.
If they choose to build a model, there are an enormous number of hurdles that need to be cleared. They must produce voluminous documentation, conduct a rigorous and detailed validation exercise, and then update their model inventory to include the new specification. The model must be tested and implemented – and subsequently monitored to ensure its ongoing suitability.
Contrarily, if the bank decides to take the brain chemistry (aka “no model”) path, the regulations are considerably less onerous.
Consequently, in most circumstances, the use of bad models is much less dangerous than using nothing at all. Unfortunately, however, the net result of banking regulation is that the construction of potentially useful quantitative models is being actively discouraged.
In short, no-model risk must become a key focus of model risk management. In cases where no-model risk trumps bad-model risk, regulators should be much less fastidious about model risk and lower the hurdles for banks to use quantitative methods.
Tony Hughes is an expert risk modeler. He has more than 20 years of experience as a senior risk professional in North America, Europe and Australia, specializing in model risk management, model build/validation and quantitative climate risk solutions. He writes regularly on climate-related risk management issues at UnpackingClimateRisk.com.
Topics: Modeling