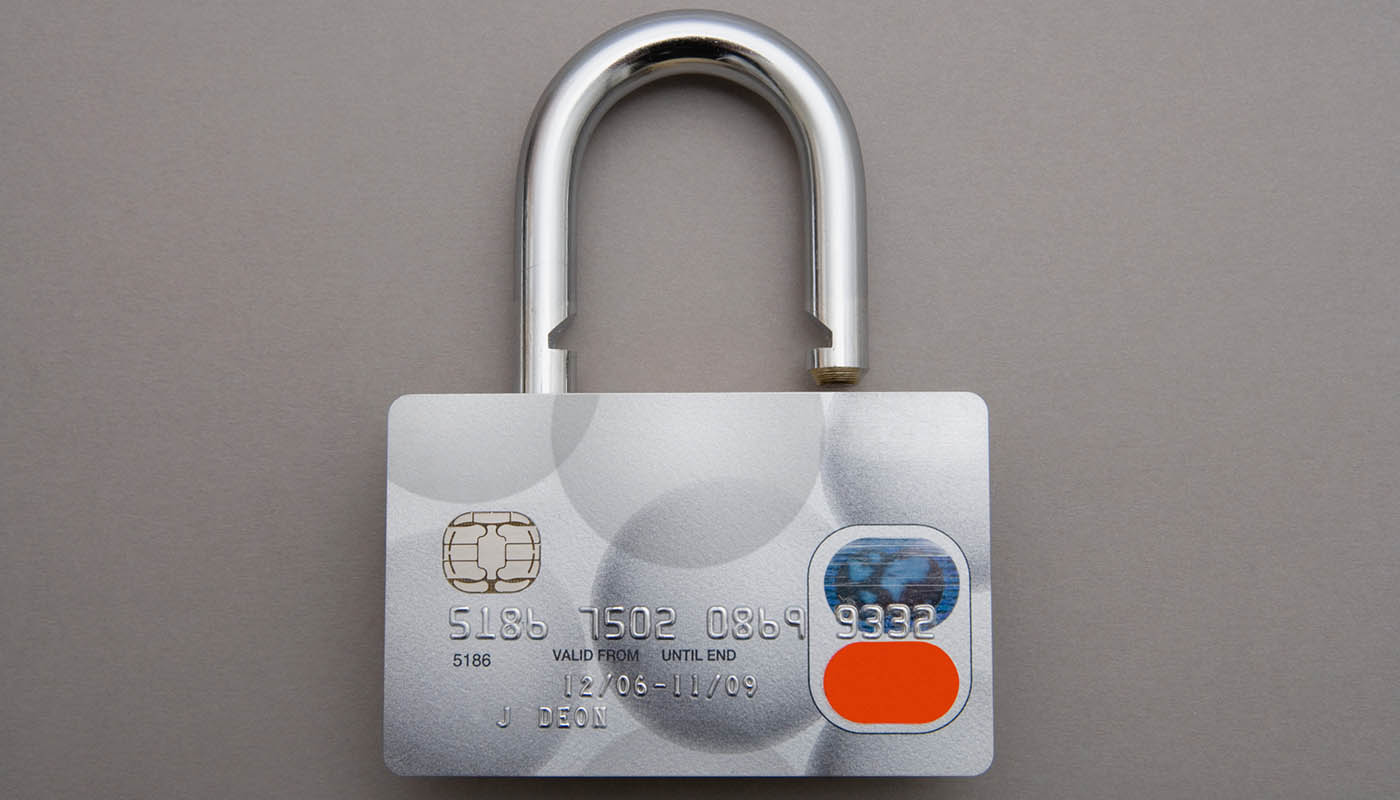
Over the past year, European courts have been grappling with the way data privacy laws impact the credit scoring industry on the continent. The rulings of the Court of Justice of the European Union (CJEU), published in December, may effectively remove the ability for European lenders to score and rank prospective clients, which is likely to have a huge impact on consumer finance in the region.
The CJEU considered two complaints against a German scoring firm, Schufa, which operates a similar business model to FICO in the U.S. The first complaint contends that Schufa was retaining data from the public insolvency register for three years, while the register itself retained the information for only six months. This meant that credit scores of insolvent individuals were impacted for a substantially longer period than their official sanction. The judgment concluded that “...at the end of the six months, the rights and interests of the data subject take precedence over those of the public to have access to that information.”
The second ruling prohibits Schufa’s ability to produce “automated individual decisions” for determining the granting of credit to individuals – a practice that is explicitly banned by the European Union’s landmark data laws. At face value, on the heels of these decisions, it seems the very practice of credit scoring is now illegal in the EU.
The Data Privacy Dilemma
To fully understand the consequences of these rulings, and to identify the winners and losers, we first need some background.
Perhaps the most valuable data that we generate as consumers is our credit histories. If detailed data on a population can be collected, statisticians can build scores that allow the credit performance of population members to be predicted with high degrees of accuracy. Over time, we have discovered that such credit scores are predictive of many desirable human characteristics, like reliability, that are strong indicators of success in other areas of human endeavor.
Credit scores can be used to exclude people from employment or from access to credit. Those who experience adverse events in their credit histories therefore have a powerful incentive to keep the information private to the greatest extent that the law allows. But data privacy comes with a cost.
Over the past decade, data privacy legislation has become increasingly strict and widespread. The EU led the way, introducing the GDPR laws in 2018 that gave individuals significant control over the manner in which businesses and government agencies can store and use their data.
Ostensibly, these rules were retained by the UK after Brexit, though it is unclear whether UK courts will take a similar stance to that of their continental colleagues. The U.S. has been slower to adopt privacy legislation, but California adopted a significantly watered-down version of GDPR.
Data privacy substantially reduces the statistical utility of credit scores. Most obviously, if many or most individuals opt to keep their data private, this will reduce the number of observations available to statisticians to build their credit scoring models.
What’s more, the type of person most likely to opt out via GDPR – those with a history of credit problems – are by far the most valuable people to have in a credit scoring sample. Delinquencies, defaults and bankruptcies are all rare events, and are thus difficult to analyze.
Statisticians will often use a technique called preference sampling to maximize the value of any defaults for which they have observations. Clean accounts can often be dropped to preserve computing resources, but defaults are the data equivalent of natural pearls.
A sane credit modeler will guard their defaults data like a mother hen. They understand that expunging insolvent individuals from the dataset will reduce the accuracy of any predictions made using the resultant score.
To build a proper credit scoring model, you must be able to populate the relevant data fields for the prospective client. If the data are public or proprietary to the scoring agency, this can be done automatically. If the data are private, on the other hand, the client must give permission for the data to be used or they must provide the data to the scorer. Under such circumstances, the issue of data verification becomes problematic.
People could also opt out of the scoring process altogether, though this would restrict the number of institutions willing to provide them credit.
Winners and Losers
Those with sound credit performance are the consumers who benefit most from a robust credit scoring infrastructure. My own experience demonstrates this very succinctly.
I emigrated from Australia – which lacked a proper credit scoring industry at the time – to the U.S. when I was in my thirties. I had a long, unblemished Australian credit history, which would have given me a high score if the technology had been available.
When I landed in the U.S., however, I was effectively a credit newbie. I took out my first U.S. auto loan at a 10% rate, which happened to be precisely the same as that which I had been paying Down Under.
In other words, if my experience is representative, those with strong credit in non-scoring countries pay roughly the same amount of interest as a novice in locations with powerful credit scores. (My second U.S. auto loan, by the way, was priced at 0%.)
As data availability is throttled and scores become less predictive, the cost of data privacy laws for those with strong credit will increase. There will be powerful incentives for companies like Schufa to try to reduce the impact of data restrictions through the use of clever statistical strategies. However, the ability of EU credit scoring companies to execute such strategies is, and will likely remain, limited.
If the very practice of credit scoring is outlawed, of course, millions of European consumers with strong credit will have to get accustomed to being treated much like recently arrived immigrants.
Setting aside corporate interests (which obviously favor liberal laws for the acquisition and use of data), we can easily identify winners and losers among consumers following the recent data privacy rulings from the European courts.
People who are excluded from credit markets, either due to past blemishes in their credit history or because of a lack of credit experience, will be the winners. Those with long, unblemished credit histories – who want their past success to be recorded and rewarded – will be the losers. (The latter group will pay much higher rates of interest as a result of the judgments.)
Parting Thoughts
When viewed through the scoring prism, data privacy laws are highly progressive in nature. Poor people, after all, are excluded from financial markets at a much higher rate than their rich compatriots – and allowing them to keep their data private will have the effect of partially redressing this imbalance.
The laws, though, make the provision of credit far less efficient for lenders and those who are credit active.
Research would be needed to confirm this, but it seems that restricting the ability of scorers to access data is sharply suboptimal to the alternative option of allowing scoring to proceed with liberal access to data. As such, the CJEO’s rulings are likely to be negative factors for economic growth in the EU.
Data privacy laws feel like they should be a no brainer. In many situations, such laws will empower individuals to control what is known about them, and thus reduce the harm inflicted by others.
Privacy, however, is not cost free. In the wake of the CJEO’s rulings, many Europeans will soon be paying higher rates on a range of consumer finance products.
It is in this form that the cost of data privacy will become due.
Tony Hughes is an expert risk modeler. He has more than 20 years of experience as a senior risk professional in North America, Europe and Australia, specializing in model risk management, model build/validation and quantitative climate risk solutions.
Topics: Counterparty