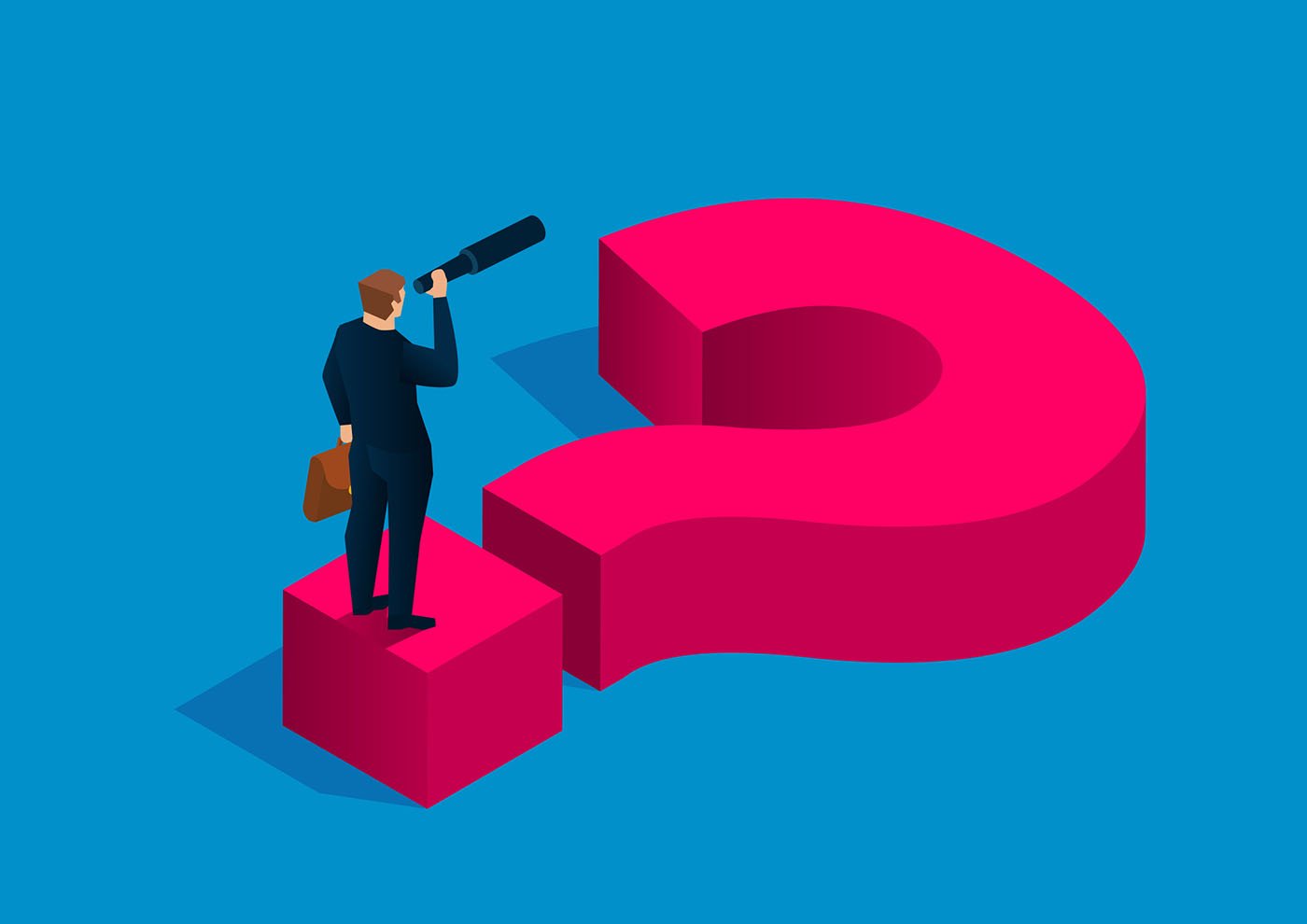
Financial institutions need scenarios to measure future risks and returns, as well as to understand how to prepare for whatever comes their way. Given the latest regulations and accounting rules (particularly IFRS 17) requiring some type of scenario analysis, the question of how to generate future scenarios has become even more critical.
The new rules and regulations are primarily intended to improve comparability and to increase transparency in financial markets. But they also aim to provide institutions, investors and regulators with an advanced understanding of both their risk exposure and the implications of strategic management actions.
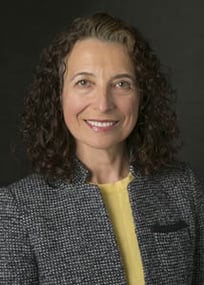
Basel IV, bank stress testing, CECL, Solvency II, and IFRS 9 and 17 are all pursuing the same objective: evaluating potential risks through the cycle, via the use of potential future scenarios (among other tools).
There are two types of such scenario generation methods: Risk Neutral (RN) and Real World (RW). Both play an important role in assessing future risks.
Each scenario is a path among many possible paths in the future. The difference between risk-neutral scenarios and real-world scenarios is not the individual scenarios themselves, but, rather, the probability of those scenarios occurring.
RN: Pros and Cons
RN probabilities are needed to price market instruments, like bonds, derivatives and structured products. The expected value of all future asset cashflows under RN probabilities equals the price of the asset.
As a consequence of the fundamental theorem of asset pricing, the RN probability measure represents a necessary and sufficient condition for no-arbitrage pricing - i.e., prices must be consistent with observable market instruments. Absence of arbitrage implies the Law of One Price, which states that two assets with the same payoff must have the same market price.
RN probabilities are also used in insurance to discount liability cashflows. Sometimes, there is not enough market data to produce all necessary risk-neutral probabilities. Under such circumstances, the closest possible substitutes are used for practical purposes.
The techniques used in such cases include expert views, historical data, and interpolation or extrapolation. Probabilities derived from these tools are used in market-consistent valuations for pricing complex financial derivatives and insurance contracts with embedded options (aka market-consistent embedded value).
But there are a few problems with relying exclusively on RN scenarios. First, the results might be quite sensitive to the date that was selected for valuation. The RN approach requires matching all available market data, but the market values of assets and their volatilities can change drastically from day to day.
Second, RN scenarios miss some of the most important features that are required for a realistic risk understanding: e.g., the incorporation of shock events and their consequences; the heavy asymmetric tails of risk distribution and increased correlations in the tails; and mean reversion and aftershock recovery.
Lastly, the local character of market consistency prevents RN scenarios from answering the key strategic risk question: what is the range of potential outcomes over the longer-term horizon? Since RN scenarios do not consider longer-term market dynamics, they omit many different aspects of reality.
For example, to generate risk-neutral scenarios for interest rates, one would need to use market-implied forwards as the mean values of future rates. But the implied forwards are poor predictors of future realized rates.
As shown in Figure 1, we observe significant deviation if we compare the implied forward rate for the three-month treasury rate, calculated three months in advance, with the realized rate for the same point in time. Moreover, the deviation is not symmetric. Implied forwards are consistently higher than the realized rates (about 83% of the time) - and, on average, exceed the realized rates by 124%.
Figure 1: RN Scenarios - Poor Predictors of Future Realized Rates
RN scenarios must therefore be used only when their purpose is to come up with a market valuation for insurance policies (i.e., the market-consistent value of liabilities) or for marking-to-market bonds, derivatives and structured products.
Breaking Down the Real-World Approach
To capture realistic market dynamics, one needs to use RW scenarios generated with the adequate incorporation of all the features listed above.
The reason this is very important is because missing potential unprecedented outcomes can lead to disastrous consequences. The life insurance crisis in Europe in the early 2000s was one such example.
In the late 90s, when interest rates were fairly high and insurance companies were competing for business, many of them sold products with high guaranteed returns (up to 7%). But when rates dropped sharply in 2002-2003, many insurance companies defaulted because, by the time they realized the scale of the disaster, it was too late to act.
Around the same time, similar crises crippled many pension funds in the U.S. Specifically, when interest rates decreased, the value of discounted liabilities went up, dropping the funded status of some pension plans (a regulatory measure of their financial health) into negative territory. However, if the pension funds had run a proper RW scenario analysis, this situation would have been prevented.
RW scenarios must reproduce all observed features of financial market dynamics both in normal and stressed conditions, through the cycle - while going beyond historical behavior. Driven by consistent models, scenarios should be able to accommodate a broad range of applications that use both real-world and market-consistent probabilities.
Benefits of a Hybrid Strategy
Different aspects of valuation and risk assessment require both real-world and risk-neutral scenarios. To combine the best properties of RW scenarios with the need to perform market-consistent (i.e., risk-neutral or its best estimate) valuation of various instruments and cashflow streams, we suggest a hybrid approach.
There are several ways to implement a hybrid approach, including (1) generating RN scenarios and adjusting them to include real-world features; (2) modifying the probabilities of RW scenarios to be market-consistent; or (3) algorithmically generating RW scenarios with RN properties.
The latter method is the most transparent and tractable and allows for the application of market-consistent valuations to both the asset and liability sides. It requires arbitrage-free discounting curves to be constructed for each scenario at each point in time, where all needed variables must be generated with appropriate and realistic dynamics.
Under this automatically-generated scenario methodology, we get a fluid interconnection of macroeconomic and market variables, forming forward-looking potential paths for RW scenarios. Subsequently, depending on the portfolio at hand, we can construct the necessary formulaic relationships needed for pricing, accounting or hedging evaluation, consistent with the market that was created on that scenario at a particular point in time.
Parting Thoughts
A comprehensive understanding of risk exposures and effective hedging demands the full distribution of scenarios that combine the advantages of the RW and RN approaches.
The risk-neutrality hypothesis is acceptable for pricing, but not for forecasting the future value of an asset. A real-world scenario must be applied for back-testing and out-of-sample validation. Consistency between these two approaches leads to consistency between asset and liability modeling.
Across the past 25 years, different financial crises have led to scenario analysis regulations that emphasized the need for consistency. Insurance crises of the early 2000s, for example, led to the development of Solvency II regulation - similar to how the LTCM crisis of the late 90s paved the way to Basel II. The global financial crisis of 2008/09, meanwhile, led not only to stress testing regulations but also CECL, IFRS 9 and, most recently, IFRS 17.
Real scenarios with path-dependent market consistency allow us to build the full distribution of risk-based capital, and to estimate its impact on earnings. Financial institutions can use this data to identify problems and adjust strategies ahead of time.
Alla Gil is co-founder and CEO of Straterix, which provides unique scenario tools for strategic planning and risk management. Prior to forming Straterix, Gil was the global head of Strategic Advisory at Goldman Sachs, Citigroup, and Nomura, where she advised financial institutions and corporations on stress testing, economic capital, ALM, long-term risk projections and optimal capital allocation.