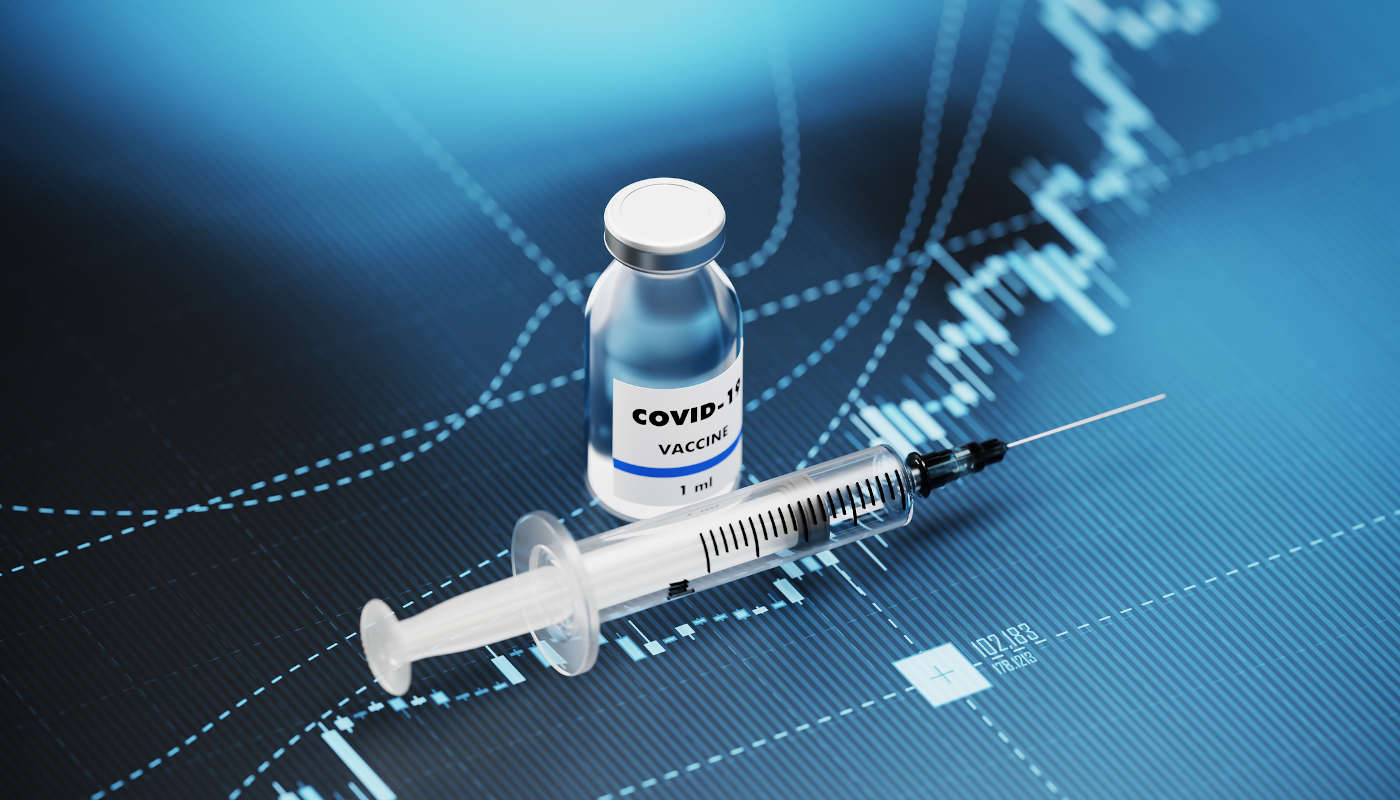
Positive news on vaccine development from Pfizer/BioNTech, Moderna and AstraZeneca/Oxford is a major step in combating the COVID-19 public health and economic crises. At the same time, coronavirus infection rates are accelerating across the United States and many European countries.
Given these conflicting forces, how should risk modelers adjust their credit loss forecasts for 2021, and beyond? Let's now consider adjustments that might be made to both economic inputs and credit loss-forecasting models.
Evaluating the Economy
Given the dependency of credit loss forecasts on the economy, accounting for the COVID-19 pandemic within the economic scenarios driving these forecasts is a reasonable approach. But this is easier said than done, as there is no history of pandemic that we can use to improve our economic forecasting models. Indeed, over the past year, even simple correlations between infection rates and economic activity have been dynamic and unstable due to changes in testing, government responses, and individual behavior.
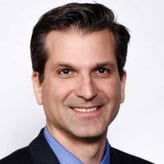
Considering these limitations, an external shock like COVID-19 can best be incorporated in a model indirectly through impact channels: the sociological, policy and confidence factors that indirectly affect “hard” economic data such as consumer spending. After these channels are identified, assumptions on these indirect drivers - such as the number of COVID-19 hospitalizations - can then be translated and incorporated into a macroeconomic forecasting model.
Though imperfect, this approach provides a logical and transparent framework for incorporating assumptions. As conditions change, shifts in the forecast can be easily explained and described in our scenario narrative.
We must first identify several key sociological and policy channels through which COVID-19 affects the economy, including consumer and business confidence, sector-specific employment and government financial support to households and businesses.
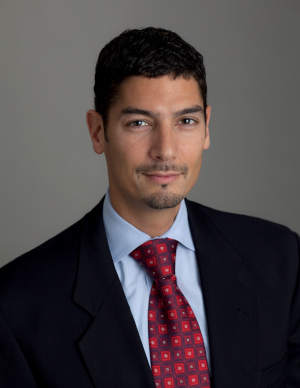
Some of these assumptions are uncertain but still measurable. For example, we might assume Congress passes fiscal relief packages of varying amounts and with different timing. Other channels, such as confidence, are not directly observed but may be inferred through surveys. All of these channels can be incorporated into our macroeconomic forecasting models.
Updating Assumptions
Dynamics complicate the analysis. We know consumer psychology plays a large role in spending and saving decisions. But our emotions and our reactions to them are constantly shifting. Case in point: Despite significantly higher levels of coronavirus infections, consumers were more inclined to go about their day-to-day business last November than they were in April.
Having identified the key impact channels, we can make assumptions and run mathematical simulations to quantify possible outcomes resulting from the COVID-19 shock, along with estimated probabilities.
Figure 1: COVID-19 Scenario Analysis
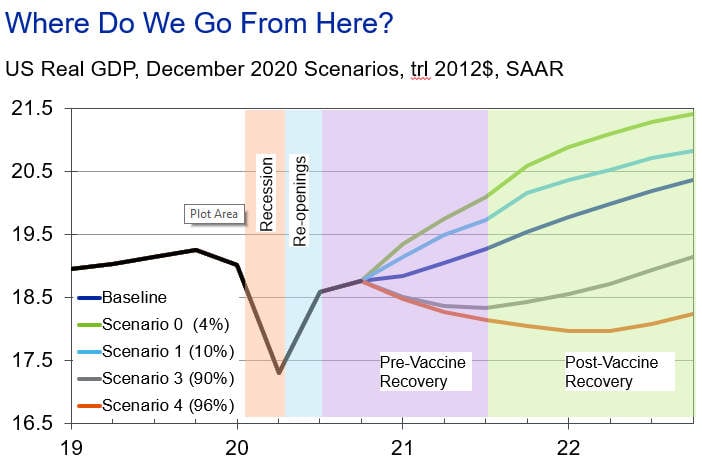
The recent vaccine announcements provide a good case study. Given the views of epidemiologists and pharmaceutical company executives, Moody's Analytics had been assuming a widely available vaccine would be distributed in mid-2021. With recent developments, we moved up our baseline assumption to mid-February 2021.
At the same time, we updated our assumptions on the trajectory of new infections to account for the recent acceleration in the spread of the coronavirus. Finally, our impact channel approach permitted us to alter assumptions around the provision of government fiscal support in the wake of US elections and a slowdown in the pace of hiring.
The framework allowed us to construct a range of upside and downside scenarios by changing assumptions for each of the impact channels. Having produced a range of plausible economic scenarios to serve as model inputs, we turn our attention to methods for translating economic assumptions into forecasted credit losses, accounting for the unique characteristics and exposures of different asset classes.
Adjusting Loss Forecasts
With economic forecasts in hand, the question of how credit risk modelers can adjust their credit loss forecasts for 2021 and beyond remains. Established, well-developed quantitative approaches used by banks, insurance companies and regulators to evaluate the credit environment have proved inadequate during the COVID-19 crisis.
Using historic relationships from the dot-com bust or the Great Recession to infer how the credit environment might play out has been insufficient. Each crisis and each recovery, by their very nature, reveal behavior incongruent to historical patterns, and require alternative data to describe their unique dynamics.
COVID-19, for instance, is having a pronounced negative impact on businesses that rely on the physical closeness of their client base, such as cruise ships, amusement parks, dine-in restaurants and downtown hotels. On the other hand, take-out restaurants and roadside lodging have fared well.
Moody's Analytics tracks credit dynamics globally across 121 granular industries, and the rank-ordering of industry performance is incredibly consistent across countries. That is to say, the most impacted industries in the US are also the ones most impacted in the UK, Germany and Japan.
Surprising? Perhaps not. Leisure and entertainment are being impacted by COVID-19 more than supermarkets, regardless of location.
Leveraging this observation, we found there are well-represented regularities between dispersion in industry performance within a country and that nation's sociological reaction to the pandemic. For example, a sit-down restaurant located in a country or region that is more impacted by COVID-19 will deteriorate in credit quality to a higher degree than if it was in a country that is less impacted.
Singapore, China, and (until recently) South Korea, for example, have controlled the virus and have seen their downtown office space utilization rates return to pre-COVID levels. Conversely, utilization rates have remained depressed in the US and the UK due to those nations' failure to contain the pandemic.
There are two primary sociological factors driving COVID's cross-industry impact : (1) consumer willingness to physically interact, use public transportation or fly; and (2) consumer and business confidence. These factors can be incorporated into credit models through an overlay.
The overlay we've developed is anchored to economic forecasts, allowing us to account for targeted stimulus and to take a stance on cross-industry performance and, ultimately, the speed of recovery. The overlay approach allows for quantification of performance that can be used in building a narrative for qualitative adjustments; it can be fed directly into credit models to produce loss forecast benchmarks to support CCAR stress testing and CECL/IFRS 9 financial reporting.
Figure 2: Pandemic and Post-Pandemic Default Forecasting Across U.S. Industries
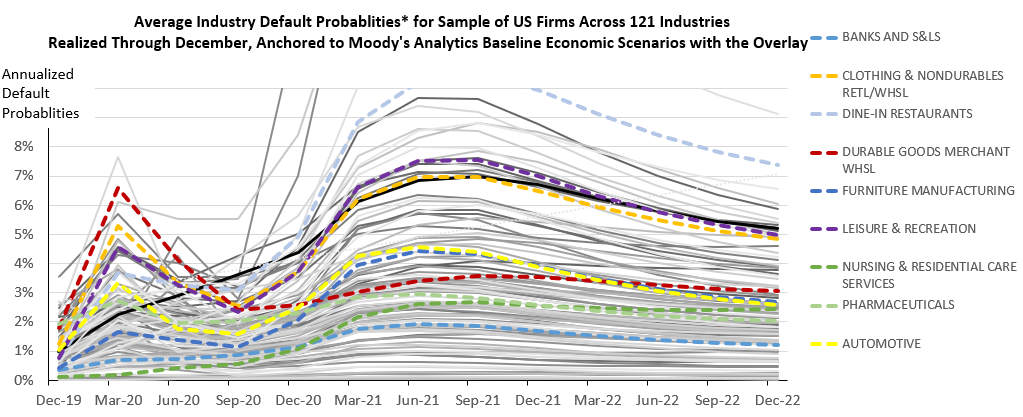
Staying Flexible for Future Shocks
The discovery, approval and distribution of effective vaccines against COVID-19 is obviously a positive development. Despite that good news, short-term risks to the economic outlook will remain elevated until vaccinations are widely available.
Given their unique characteristics, incorporating exogenous, pandemic-driven shocks into an economic forecast or a credit loss model is challenging. The methods we have proposed provide a transparent, logical framework for dealing with uncertainty, rather than over-engineering a specific solution.
A rational, flexible process is particularly relevant when thinking about managing a new shock that is subject to change and evolve like the pandemic. By adopting these approaches, we can adapt our credit loss forecasting processes to address whatever external shock comes next.
Cristian deRitis is the Deputy Chief Economist at Moody's Analytics. As the head of model research and development, he specializes in the analysis of current and future economic conditions, consumer credit markets and housing. Before joining Moody's Analytics, he worked for Fannie Mae. In addition to his published research, Cristian is named on two U.S. patents for credit modeling techniques. He can be reached at cristian.deritis@moodys.com.
Amnon Levy is a Managing Director in the Research and Modeling Group at Moody's Analytics. He heads the Portfolio and Balance Sheet Research group that is responsible for designing quantitative solutions and provides services related to Moody's Analytics credit portfolio, capital management, asset-liability management, credit valuation, impairment and stress testing solutions. He can be reached at Amnon.Levy@moodys.com.