Artificial intelligence is growing rapidly and could have a transformational impact on the banking industry, but according to McKinsey & Co. risk practice experts, it is not a risk-free event.
If AI-based, machine learning models are not properly designed and validated, their inherent complexity, the decisions they make and the vast quantities of data they employ can give rise to a variety of unintended - and difficult-to-control - consequences and risks.
Banks could, for example, find themselves unintentionally in violation of anti-discrimination laws, or not complying with anti-fraud or anti-money-laundering regulations, or taking high-risk or unprofitable investment positions. The McKinsey consultants, however, are confident that a solution is at hand.
Derisking Machine Learning and Artificial Intelligence, an article by McKinsey partner Derek Waldron and several colleagues, makes the case for a model risk management exercise: The added risk brought on by machine learning can be effectively mitigated - most notably, in the financial sector - by making well-targeted modifications to existing validation frameworks, as required by regulators - in short, by de-risking the models
In Line with SR 11-7
The de-risking steps proposed by McKinsey would apply to the validation frameworks already employed by banks and supervisors - specifically, those consistent with the SR 11-7 guidance of the Federal Reserve Board and Office of the Comptroller of the Currency. Banking organizations must be attentive to possible adverse consequences of all - not just machine learning - models. The regulators also call for active model risk management, including effective validation.
“These enhancements would basically help manage the risk associated with machine learning and artificial intelligence models,” Waldron says of the McKinsey recommendations.
He notes that overall model risk governance and validation processes are “owned” by the chief risk officer and, in that person's organization, the head of model risk management.
“The head of model risk management owns the framework of how to think about model risks and the specific requirements that go into validation,” Waldron says. Under his or her guidance, there potentially can be hundreds of individual validators who then need to go through each of the steps necessary to validate the models. Many of these individuals would bring relevant technical skills.
Six New Elements
Specifically, McKinsey is proposing the addition of six elements to the validation process: model interpretability, model bias, feature engineering, hyperparameters, production readiness, and dynamic model calibration
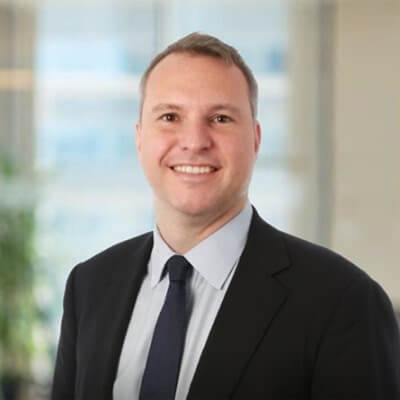
The firm also advises the modification of 12 elements, such as in areas of modeling techniques and assumptions, that are part of traditional validation frameworks.
“The good news is that banks do not need to reinvent a whole new validation framework for this,” Waldron says. “The added risk can be mitigated with some very well targeted enhancements to the existing frameworks, with six specific new elements.”
For example, there are four main types of model bias that can occur: sample, measurement, algorithmic bias, and bias against groups or classes of people. The McKinsey article points out that when machine learning models are employed, both algorithmic bias and bias against people can be significantly amplified.
In the case of algorithmic bias, McKinsey suggests the development of “challenger” models that use alternative algorithms to benchmark and ultimately correct model performance.
Defining Fairness
To address instances of possible bias against groups or classes or people, McKinsey advises that banks first decide what constitutes fairness for a specific model, and whether that would require demographic blindness, demographic parity, equal opportunity, or equal odds. Validators then need to decide whether developers have taken the necessary steps to ensure fairness
The article explains that models can then be tested for fairness and, if necessary, corrected at each stage of the model development process, from the design phase through to performance monitoring
Feature engineering is the process of creating and manipulating predictors or predictor variables, which guide machine learning models so that an effective predictive model is produced. Careful validation is important, as this is a process that can go terribly awry. The article notes that auto-machine learning, or AutoML, packages, which are designed to automate feature engineering, generate “large numbers of complex features to test many transformations of the data. Models produced using these features run the risk of being unnecessarily complex, contributing to overfitting.”
An instance cited in the report: an AutoML-built model that “found that specific sequences of letters in a product application were predictive of fraud. This was a completely spurious result caused by the algorithm's maximizing the model's out-of-sample performance.”
Waldron says that better validation of the model's feature engineering could have readily picked up the miscalculation.
“Feature engineering is often much more complex in the development of machine learning models than in traditional models,” Waldron explains. Thus, with AutoML, ”there is a risk that transformations which appear predictive at first may in fact just be overfitting the data.”
Supporting Rationale
To facilitate effective validation processes involving machine learning models and, in particular, feature engineering practices, McKinsey recommends the creation of a policy about how much supporting rationale may be required from each predictive feature in their machine learning models.
“Some banks may take a conservative stance and require that every predictive feature in each model, wherever possible, have supporting rationale,” Waldron says. “Alternatively, banks may require such support only for their highest-risk models,” such as those involved in making credit risk decisions.
Waldron acknowledges that there are challenges in addressing a range of validation issues. He notes that in a McKinsey survey last year of model risk management leaders, 50% believed that insufficient technical talent was a top challenge for managing model risks that are amplified by machine learning and artificial intelligence. Clearly, risk management executives are concerned about their ability to identify staff with the requisite skills.
At the same time, Waldron stresses the importance of banks taking steps sooner rather than later to improve their validation processes, to help ensure the accuracy of machine learning models.
“Only two or three years ago, the use of machine learning and artificial intelligence was more theoretical and in the future,” the McKinsey partner notes. “Today, we are seeing leading banks being confronted with pipelines of machine learning and artificial intelligence models that need to be validated, so this is really a problem in the here and now that we expect to see continue to grow.”
Katherine Heires is a freelance business journalist and founder of MediaKat llc.
Article Updated: November 5, 2021