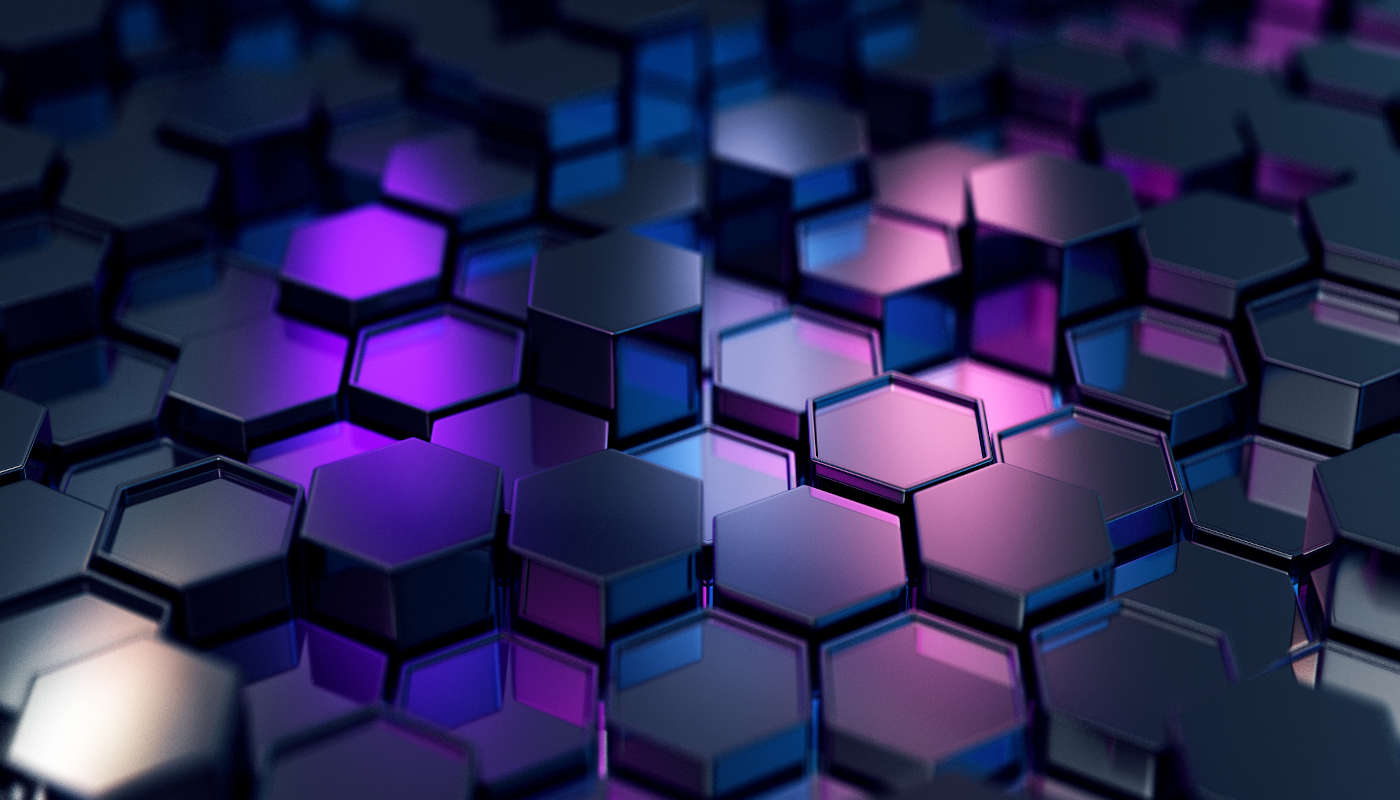
The end of the year is an opportune time to assess the risk management implications of three evolutionary trends that defined 2020 for fintechs: the increased commercialization of artificial intelligence (AI), the further proliferation of automated analytics, and the rapid integration of digital payment systems.
The broad, strategic aspects of increased AI adoption across multiple industries were detailed in McKinsey & Company's "State of AI in 2020" report, which identifies a widening gap between companies that integrate AI in their businesses and those that do not.
Increased adoption of AI (compared to the previous year) was reported by 28% of respondents, and 8% indicated they have incorporated AI in their capital allocation processes. Finance, the McKinsey report elaborates, is now the third largest industry for AI use, behind technology and manufacturing. Risk modeling and analytics (with a 16% adoption rate) and fraud and debt analytics (12%) are among the groups that used AI most prevalently, according to the report.
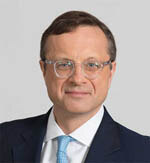
These trends illuminate the increasingly broader use of AI in financial services, which is partially driven by fintechs. Indeed, many financial firms have turned to fintechs for hard-to-come-by AI product development expertise, eschewing in-house development.
There are, however, risks to the approach of relying on fintechs for externally-vended AI solutions. These vendors are frequently new to the business, with limited expertise and experience in providing solutions to the financial services industry. What's more, beyond being start-ups, many fintechs that offer AI services culturally do not understand the inherent challenges of developing new products in a regulated environment.
Of course, as more and more AI-driven products and services are rolled out, third-party risk management (e.g., of fintech-provided AI solutions) will become a strategic risk that risk managers will increasingly have to shoulder. But the challenge goes beyond simple AI risk management or third-party risk management.
Banks need to consider the coincidental and interdependent nature of externally vended, complex AI solutions, many of which have just breached the commercialization threshold. Simply put, risk managers at banks will need to learn how to identify, measure, manage, and mitigate these third-party risks, some of which are already on the regulatory radar.
While third-party risk management is an important component of this equation, firms also must better understand the limitations of what AI can do today. Though AI use is on the rise, management of this disruptive technology remains on the backburner at many firms.
Specifically, many of the McKinsey survey respondents stated they have yet to address comprehensively the known, universal risks of AI - such as algorithmic bias, misspecification and other modeling shortcomings. Indeed, only 25% of respondents indicated that they have taken steps to mitigate concerns around AI explainability, while just 30% have addressed AI privacy issues. Given these facts, it should not come as a surprise that only 22% of respondents stated that they are actively trying to manage reputation concerns tied to AI implementation.
Further Proliferation of Automated Analytics
With the rapid shift to digital customer interactions during COVID-19, many financial institutions quickly implemented new (retail) customer-facing chatbot solutions that are driven by automated analytics.
Chatbots help clients, customers and staff interact seamlessly with each other - but do not always behave as they should. Since chatbots rely on the complex integration of natural language processing with dynamic data analysis, they model different data types using complex tools, and rely on model-dependent results.
Effectively, the performance of any chatbot depends on the performance of series of interlinked - and sometimes interdependent - models. But when there are gaps across these interlinked and interdependent models, chatbots do not work as intended. These gaps in performance raise questions about the overall reliability of these complex automated solutions.
Automated analytics have also been deployed in support of fraud detection, know-your-customer (KYC) processes, robotic process automation, and regulatory compliance at fintechs. To varying degrees, when driven by analytics, all of these functions suffer from the same problems as chatbots, and all face complex model risk management challenges.
To address challenges with automated analytics, sequential data and algorithmic dependency, risk managers must continue to take a deliberate, well-balanced, multidisciplinary approach. While the reliability of automated analytics is likely to improve over time and become even more user friendly, the underlying modeling will only become more complicated. That is why many leading organizations have now defined their own internal standards on how to best explain underlying algorithms to non-technical staff, senior executives and their boards.
The Integration of Digital Payment Systems
COVID-19 shifted spending patterns: consumers moved into the digital world and paid for their online purchases using digital and contactless payments. Meanwhile, cash usage continued to decline, and fintechs increased the global use of digital payments across all industries and sectors.
The commercial benefits of integrated payment technologies were illuminated by COVID-19: fintech payment providers can act as an intermediary between banks and their customers, offering seamless flexibility.
Unlike banks, fintechs can rapidly scale their operations to meet customer demand due to their nimble size, their lack of legacy technologies, and their lower operational cost ratio. Banks that do not embrace partnerships with digital payment providers (including fintechs) as part of their operational strategy may find themselves behind the curve.
There is a big caveat, though: the WireCard debacle. As German regulators continue to uncover the collapse of this global payment fintech, a more than likely reaction will be the introduction of some oversight - if not outright regulation - of fintech payment providers.
The scope of this regulation remains to be seen, and will depend on the outcome of governmental and regulatory scrutiny. But increased regulatory oversight will introduce more model risk and third-party risk into the risk management frameworks of digital payment providers, both in the U.S. and Europe.
Parting Thoughts
No year-end review should end without some prognostication. One of the breakthrough technologies to keep an eye on in 2021 and beyond is GTP-3, an autoregressive language model (with 175 billion parameters) that could have a transformational impact on the development of human-assisted cognitive technologies.
This next-generation technology relies on an advanced algorithm to create intelligent text, and can potentially be used for everything from authoring tweets and emails to translating languages to writing code. Indeed, GTP-3 may become a disruptive force in a world that hopefully is COVID-19 free, and the risk management implications of its expected rise are significant.
Peter Went (PhD, CFA) lectures at Columbia University on disruptive technologies, such as artificial intelligence and machine learning and their impact on financial services and financial risk management. He also advises privately-owned and publicly-traded companies in the U.S., and elsewhere, on the impact of artificial intelligence.