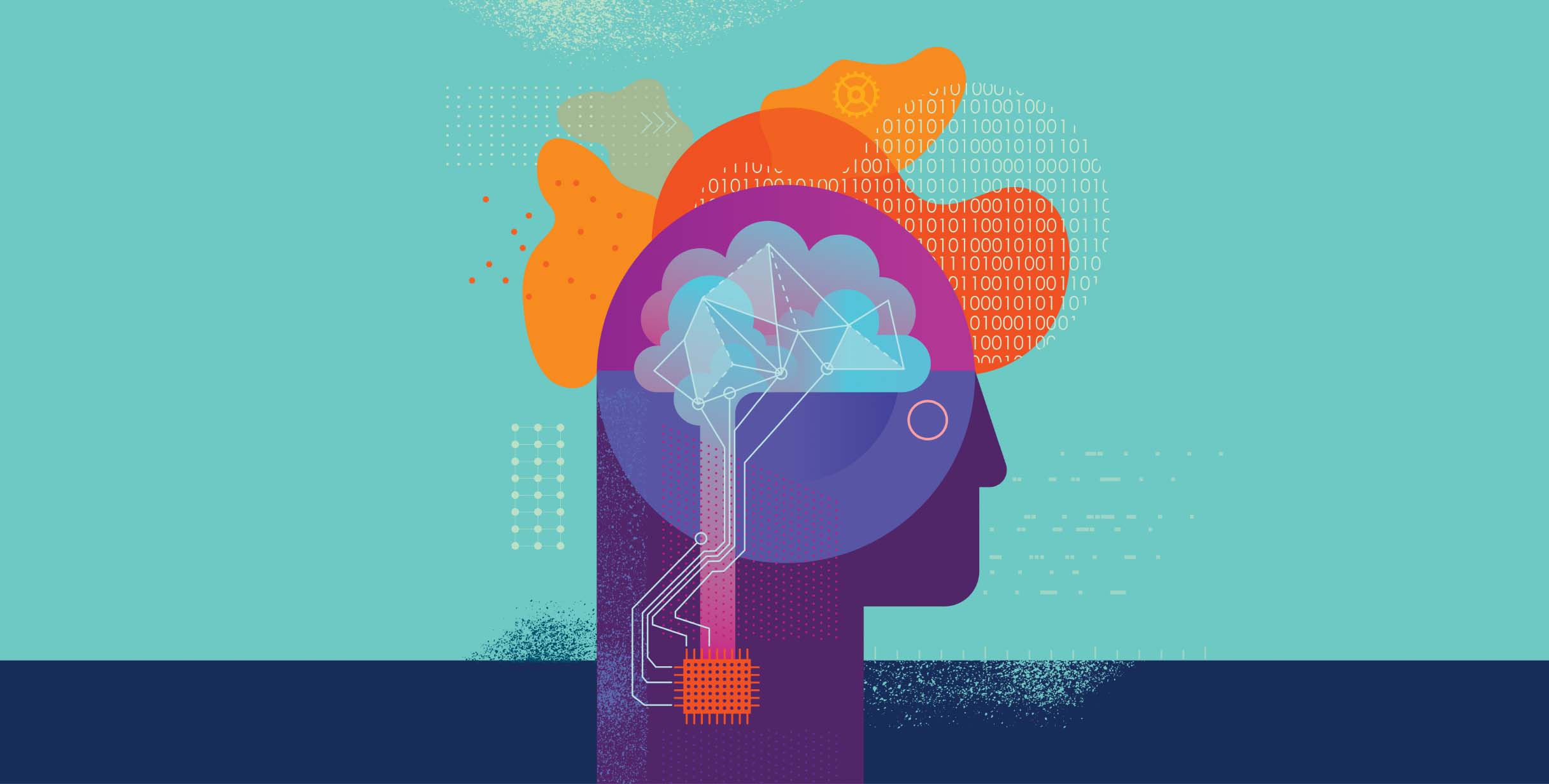
Until now, European banks have been hesitant to apply machine learning (ML) to their internal-ratings-based (IRB) models for credit risk. The supervisory requirements they had to meet to ensure they have enough capital to cover their credit risks restricted the use of models driven by disruptive technologies, but a discussion paper recently released by the European Banking Authority (EBA) may be a game-changer for banks’ deployment of ML-driven IRB models.
Marco Folpmers
The adoption of ML-driven IRB models has, to date, been slow – and, in the case of many European banks, non-existent. To appreciate the change the EBA discussion paper brings, let’s first assess the current difficulties of applying ML to IRB models.
While ML models (e.g., random forests or neural networks) may lead to higher discriminatory power as compared to “traditional models” (say, logistic regression), they are lacking a number of attributes that are needed for easy adoption within a regulatory IRB context. Let’s now take a look at five reasons why ML-driven models have had difficulty gaining traction in the IRB credit-risk realm:
First, IRB models need to be “intuitive” (see CRR, art. 179). There must be an easy-to-understand link between the risk drivers and the default indicator for PD models. Traditional models fit the bill: they often demonstrate very clear and immediately quantifiable relationships between a risk driver (e.g., loan-to-income) and default “Yes/No.”
Second, IRB models require careful documentation. This documentation, moreover, must be validated and combined with the model code. Traditional models are easy to document. Although model documentation can be hundreds of pages, one can summarize the core functioning with only one equation (the equation for the logistic regression) and explain what risk drivers the model inputs () represent. ML-based models tend to have more difficulty documenting the design, the operational details, the underlying theory of the model and the modeling assumptions (see CRR, art. 175).
Third, a traditional model operates on a limited number of risk drivers – say, 10 or 20, rather than 100+ drivers typically used by ML-driven models. The limited dimensionality of this input data makes it easier to comply with the EBA-mandated obligation to have five years of data history. The difficulty of this task increases exponentially when a bank uses more risk drivers, since the easy risk drivers, with easily accessible history, are used first.
Fourth, there’s the issue of governance. Senior management is supposed to have a high-level understanding of the bank’s IRB model for credit risk. For example, in the EU, article 189 of the CRR states that senior management needs to have a “good understanding of the rating systems designs and operations.” For logistic-regression-based PD models, this is feasible, because one does not need a Ph.D. in applied mathematics to understand the basic relationships and dependencies.
Fifth, there is regulation that limits the possibilities of collection and processing of big data repositories about “natural persons.” In the EU, this will be regulated in the AI-act, which attaches the highest risk to AI systems that assess a natural person’s credit worthiness.
Current, Limited Uses of ML
Given the limitations outlined above, how can ML actually be applied to credit risk today? ML models within credit risk currently serve three primary purposes for banks.
First, ML is used to develop challenger models for validation. (These are called “model challengers” by the EBA, by the way.) These challenger methodologies are models than can be set up quickly, via reusable code, to establish a most optimistic or upper boundary for discriminatory power during “laboratory conditions.”
A second application is variable preprocessing. With the help of ML, credit risk variables can be selected or combined – to establish a highly predictive set or combination of variables for subsequent use in a traditional logistic regression. (We discussed this type of application in a previous article.)
Lastly, ML can be applied to internal credit risk models outside of IRB – e.g., for credit approval processes.
How Can ML be Properly Leveraged?
The EBA discussion paper will undoubtedly stimulate discussion on further use of ML within IRB models. Though it acknowledges the aforementioned obstacles, the purpose of the paper is to develop a perspective on how to advance ML-driven models while mitigating any negative impact.
The EBA expressed optimism that ML-driven models for IRB can “improve risk differentiation.” Since better risk differentiation leads to more stable banks, this advantage does not only benefit banks but also society as a whole.
Of course, the apparent advantages of using ML to drive IRB models must be weighed against perceived disadvantages of disruptive technologies – including, most notably, low explainability. Interestingly, the EBA mentions a number of tools that can help mitigate the risks of ML-driven IRB models.
Firstly, the fundamentals of the model must be robust. Staff need to be trained on ML techniques, and cross-validations need to be in place to avoid overfitting. Moreover, the economic relationships that drive the model should be assessed – e.g., one should make sure that a lower income increases the probability of default, rather than a higher income.
To improve the explainability of ML-driven models, banks need to invest in specific techniques (e.g., visualization and importance scores ) that can lead to a better understanding of model dynamics. The EBA specifically mentions Shapley values, which can indicate the drivers that have been most important in the establishment of the final (i.e., default “Yes/No”) model outcome.
With the help of visualization and Shapely scores, the underlying dynamics of ML-driven methodologies can be better explained to senior management, so that they can have a sufficient understanding of the model.
To further improve this understanding, the EBA also recommends “local explanations” and “counterfactual explanations.” Local explanations capture the behavior in a specific area in the vicinity of an observation, by way of an approximation. Counterfactual explanations explore how a specific prediction (e.g., default) may have been altered by small changes in the values of the risk drivers.
Parting Thoughts
Whereas supervisors were previously, at best, lukewarm to ML-based IRB models, the EBA's recent paper offers a clearly positive view of ML – citing the ability of this technology, potentially, to improve risk differentiation in IRB models.
It is up to the financial services industry to evaluate the EBA’s discussion paper and to answer the questions contained in it. The idea is to determine whether the adoption of ML within IRB will ultimately lead to more effective regulatory models for credit risk.
Dr. Marco Folpmers (FRM) is a partner for Financial Risk Management at Deloitte Netherlands.