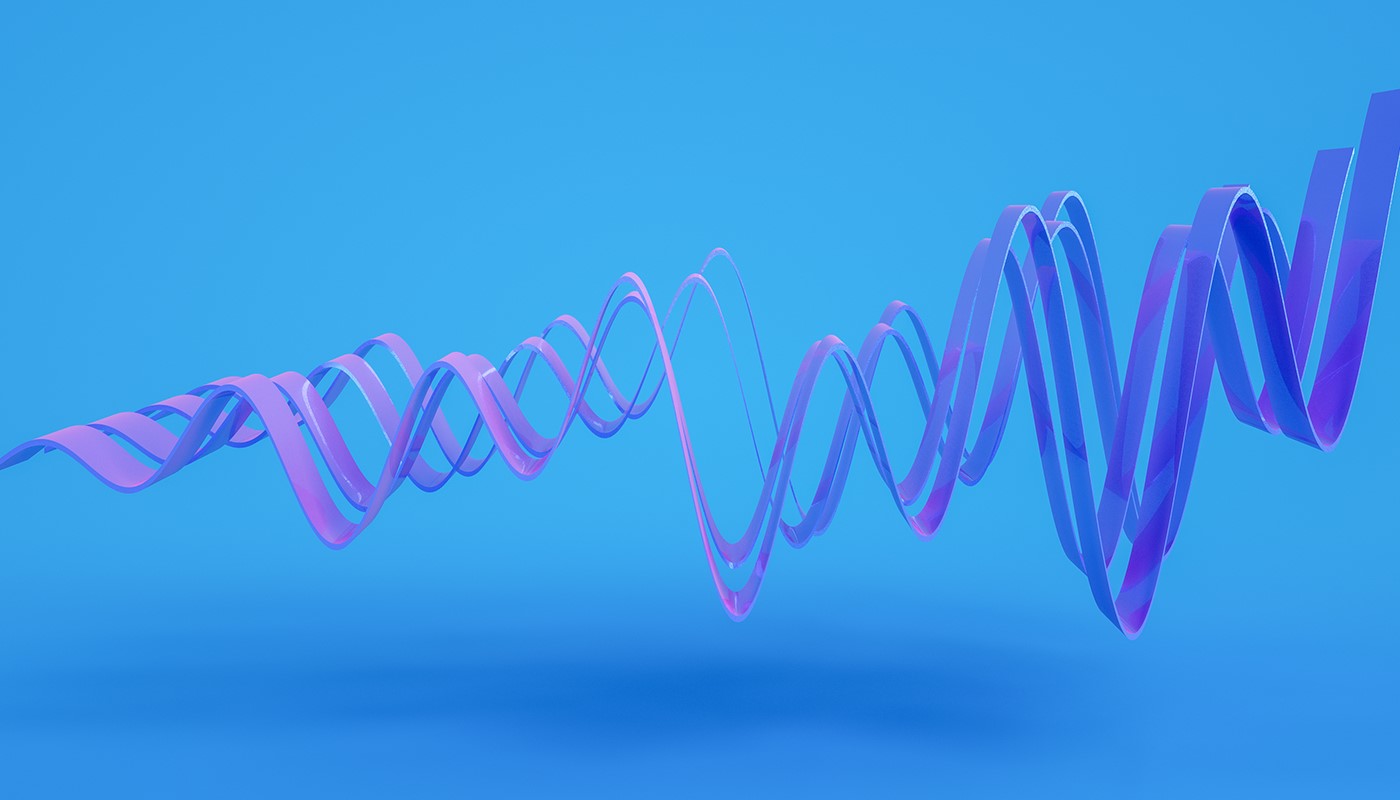
What do Carly Simon's 1971 hit single and Amazon's 2013 patent for forecasting product demand have in common? They both focus on the concept of anticipation. As data and models permit faster, more accurate forecasts, the opportunity for companies to foresee both the needs and risks of their customers is greater than ever.
Indeed, continuing advances in machine learning and artificial intelligence are opening a new economic era: the anticipation economy.
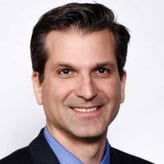
Manufacturers, financial institutions and other companies have always had to forecast future demand for their products and services - to ensure they produced enough and staffed up appropriately. The anticipation economy goes one step further to predict demand at an individual level, accounting for both the specific time and place that demand will occur.
In the financial services space, we're already seeing evidence of the anticipation economy in areas like credit loss forecasting (more on this, below). In the broader world, Amazon's Prime Now is a prominent example.
Timely and accurate forecasting is critical for this service, which guarantees free delivery of household items and essentials within two hours in many metropolitan areas. Amazon must not only anticipate demand for, say dish soap, within a broad region of the country, but also position the product as closely as possible to potential customers - before the customers themselves have realized their own needs.
Based on the growing volume of data being collected, it's entirely possible that Amazon will start making pre-emptive deliveries. That is, dish soap will show up on a customer's doorstep without having been ordered. While customers will have the option to send it back, Amazon may have the algorithmic confidence that nine times out of 10, the shipment will be appreciated and kept.
Deriving Value from IFRS 9 and CECL
Whether or not you agree with the adoption of IFRS 9 and current expected credit losses (CECL) as accounting standards, you should recognize that complying with these rules can help firms increase revenues, decrease losses and expand profitability by anticipating the financial services customers will need in the future.
.jpg?width=164&name=a2r1W000000x4SxQAIBiradeLaurentCW5431(002).jpg)
What's more, some of the benefits of improved credit loss forecasting (via IFRS 9/CECL compliance) are obvious and immediate. Better information systems reduce the time required to analyze portfolios, allowing for more timely and accurate answers to strategic questions. Frequent updates to expected credit loss forecasts, meanwhile, enable greater consistency in credit risk assessment throughout the lifecycle of each loan booked.
Origination, pricing and servicing activities, moreover, can now be synchronized through expected credit loss models, forcing consistency throughout the institution - including financial reporting.
But the benefits from CECL and IFRS 9 have the potential to go far beyond these tasks. Upgrades in systems and algorithms can play an integral role in the rise of the real-time forecasting that drives the anticipatory economy.
How to Adapt to the Anticipation Economy
In the anticipation economy, financial institutions must take advantage of what they know about their customers to guide these actions - via, for example, creating a business strategy; setting rules for how the institution interacts with its customers; and establishing an approach for the way the institution manages customers through the loan lifecycle.
By improving how they serve customers and manage their risk-adjusted returns, financial institutions can make better decisions to reach their profitability goals.
In the examples below, we focus on the capabilities and information that CECL and IFRS 9 implementations have provided to financial institutions about customer behavior and expected performance. We propose using that information to streamline how institutions interact with customers along five key dimensions: relationship pricing, portfolio management, credit review, deal targeting and deal structuring.
Let's now take a closer look at each:
- Relationship pricing can drive our understanding of the profitability of each customer. In the anticipation economy, customers want to be rewarded for the relationships they bring to a financial institution - whether that's a checking account, an auto loan or a business payroll.
Just as Netflix subscribers expect a personalized selection of content, customers will expect financial institutions to offer a variety of customized insights and services. Improved credit models developed for IFRS 9 and CECL can facilitate mass customization, with lenders able to provide more competitive features, loan options and interest rates. Key insights can drive the way loan officers expand relationships, via aligning incentives and focusing on profitable growth business strategies.
- Portfolio management is an essential part of managing a financial institution's credit exposure. Establishing a “flood gauge” based on credit loss estimates, and aligning risk appetite with levels of expected default, will enable portfolio managers to better understand where the book stands and what types of mitigating actions they can take.
For example, a portfolio manager with a weighted average default of 95 bps on his or her book might be deemed as not taking enough risk. Instead of waiting for portfolio analytics to be run after loans have closed, loan-specific loss estimates developed for CECL can provide a mechanism to drive increased, real-time risk taking by the originations staff. Doing so will help increase the yield and optimize risk taking - and may enable the production staff to tap into a market (e.g, less-creditworthy borrowers) they would not have otherwise considered entering.
- Credit review can be a time-consuming process for any institution. But firms that have invested in CECL and IFRS 9 models (as well as forecasting processes) can continuously review credit exposures based on the latest data updates and economic scenarios - rather than probing exposures at fixed monthly, quarterly or annual intervals.
More frequent updates of credit loss estimates can establish a new paradigm for credit review, moving from periodic to trigger-based reviews where most high-risk credits would be reviewed monthly. What's more, the rest of the book could be prioritized in terms of riskiness, based on CECL or IFRS 9 estimates.
Under this approach, credit review staff can focus on those credits that require attention, instead of reviewing loans based on geographic location or industry segment.
- Deal targeting involves anticipatory models that can help lenders offer deals to potential customers based on their specific needs and opportunities. Looking at credit and deposit data from millions of customers can lead to insights on the next best anticipatory step for specific customers - to either produce a positive outcome or mitigate a negative one. Financial institutions can use this approach to support a variety of customers, including those who are ready to buy a new home, renovate an existing one, find a new job or deal with a layoff.
A deeper understanding of the customer lifecycle, moreover, may soon enable financial institutions to take a page out of Amazon's playbook with pre-emptive financial products - not just pre-approvals. A lender might take the initiative, for example, to consolidate a borrower's revolving debt into a fixed-rate installment loan automatically, when an algorithm signals a high propensity for the borrower to consolidate their debt with another lender. Similarly, lenders can improve customer retention by offering one-click mortgage refinancing to highly-qualified borrowers.
- Deal structuring takes advantage of new tools, enabling loan originators to anticipate the needs of insurance companies, institutional investors and buyers of their loans. These tools permit originators to create asset-backed securities (ABS) that satisfy real-time demands for credit, yield and duration.
Understanding what drives cost and profitability under the accounting standards can help loan production staff generate optimal deal structures for both lenders and investors. The right combination of product type, maturity and renewal mix, payment structure and prepayment options can help lenders maximize profitability and improve customer relationships before deals are closed. This could help firms produce optimal strategies for both capital allocation and origination in real time.
Parting Thoughts
Long ago, ancient humans learned to predict the seasons - and we've been “anticipating” ever since. The only difference now is that our algorithms have become faster and more precise.
Taken to an extreme, the idea of an anticipatory economy may sound dystopian, with each of our individual behaviors manipulated by some soulless algorithm. While the possibility of new tools being used to concentrate power or manipulate outcomes is real, we are still many years away from developing an AI that goes beyond optimizing a specified problem or strategy.
Competition and decentralization of technology can help check the abuse of algorithms and offer hope that these tools will be used to achieve broader goals - including managing climate change, reducing inequality and achieving greater financial access.
Arguably, the CECL standard was implemented at the worst possible time, but it provided key insights into how fast the credit cycle can turn. That experience, and the information gathered, should not go to waste. By instituting a common view of risk across the credit lifecycle, we can drastically change the way financial institutions operate, producing greater returns for both customers and lenders.
As Carly Simon's song suggests, anticipation can be both thrilling and anxiety-inducing. The anticipation economy has already transformed the retail and entertainment sectors - and is spreading to every other industry, including financial services.
Will we be part of the transformation or will we be transformed by it? There is no time like the present to anticipate the future.
Cristian deRitis is the Deputy Chief Economist at Moody's Analytics. As the head of model research and development, he specializes in the analysis of current and future economic conditions, consumer credit markets and housing. Before joining Moody's Analytics, he worked for Fannie Mae. In addition to his published research, Cristian is named on two US patents for credit modeling techniques. He can be reached at cristian.deritis@moodys.com.
Laurent Birade is a Senior Director at Moody's Analytics. He has more than 20 years of experience in finance, credit, risk management, planning and IT. He is responsible for providing risk and finance integration expertise across solutions, products and services offered by Moody's Analytics in the United States and Canada. He has participated in numerous implementation projects and advised financial institutions on key regulatory issues such as CCAR and DFAST, as well as IFRS 9, CECL credit loss reserving and the credit lifecycle of loans. He can be reached at laurent.birade@moodys.com.
Article Updated: May 23, 2024