There are throngs of varying types and sources of alternative data.
Unlike traditional models – those made available by financial exchanges and indexes, SEC filings, financial statements, corporate filings, analyst predictions, press releases, management presentations and other well-entrenched mainstream sources – today’s breed of alternative data sets are being compiled from wide ranging and disparate sources. Everything from financial transactions, satellites, sensors and IoT-enabled devices to e-commerce portals, public records, mobile devices, social media, web traffic and more are tapped. However, web scraping and financial transactions are, by far, the most common methods of alternative data procurement.
Even as alternative data, including ESG (environmental, social and governance), has made inroads into the investment, risk, marketing and other key processes, firms vary in their experience with effectively integrating alt-data into their infrastructure and decision-making.
Julia Valentine, Managing Partner, AlphaMille
There are two main approaches to alternative data integration: The accelerated approach that is based on selecting one process or using case as a pilot; and a comprehensive approach.
Both have merit.
For example, a private equity fund that wants to incorporate alt-data into its investment process may identify its equity growth strategy as a pilot and focus on choosing relevant vendors based on a list of over 400 providers, while deriving insights that create massive business value through a proprietary informational advantage.
Re-evaluation Step
A comprehensive alt-data approach is appropriate when a firm wants to re-evaluate its current technology and data capabilities, understand its current appetite for deriving data-driven insights and incorporate those into decision-making processes. This aids in developing the capabilities for ingesting unstructured data and building out machine learning models – ultimately strengthening its data analytics and reporting capabilities. Building in risk evaluation and implementing appropriate controls is an essential part of the process.
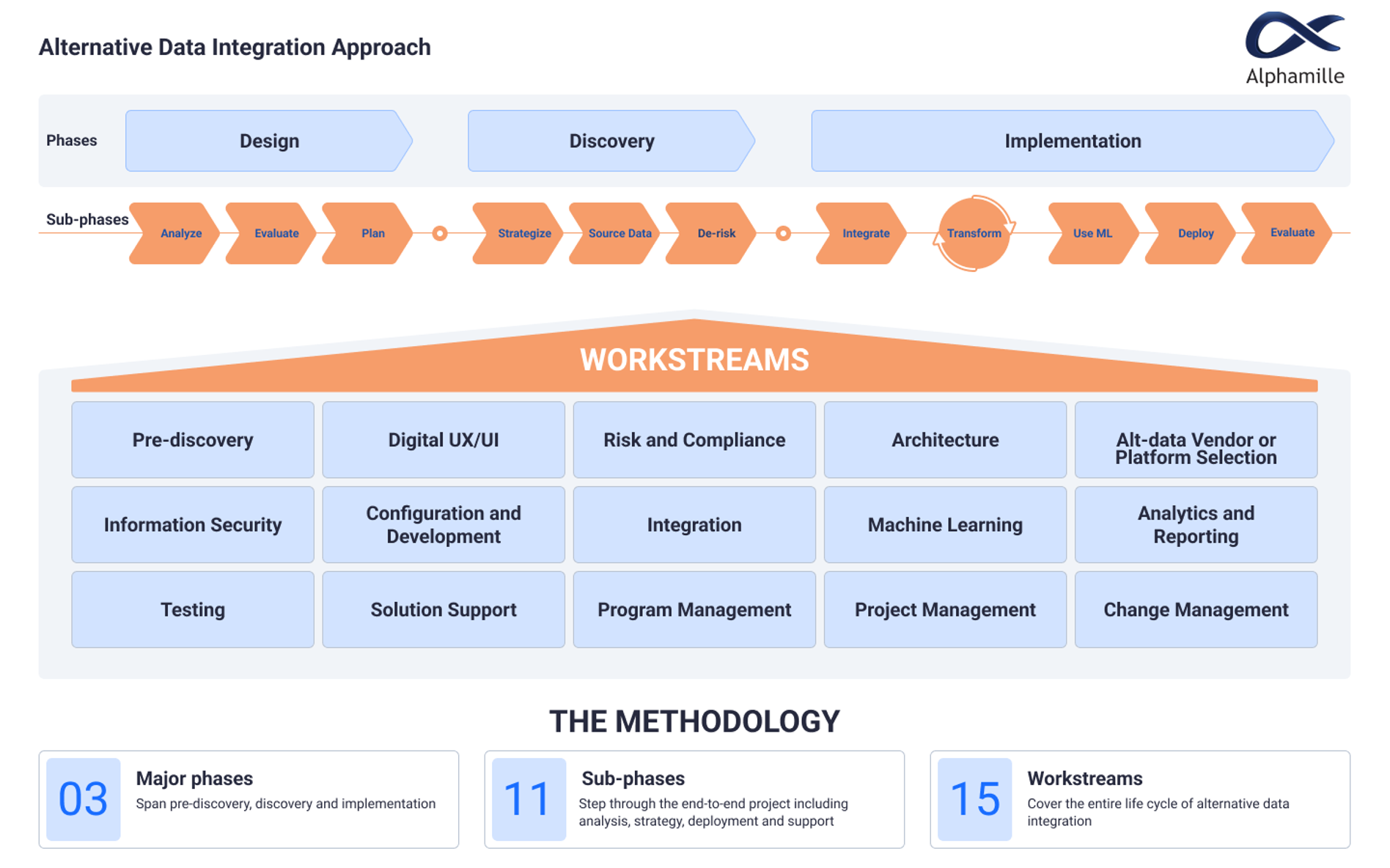
The following are the three main stages involved in a comprehensive alternative data implementation.
- Design
Analyze: Often called the current state analysis, the first step of any technology and data implementation project is to understand the state of infrastructure readiness, or its capabilities.
Evaluate: The evaluation of current capabilities may result in the realization that the firm is ready to incorporate additional data, analytics and machine learning abilities into its technology and business processes, or it may identify additional capabilities that are necessary.
Plan: A revised target operating model and technical architecture are outlined, project management and technical teams are launched and change management principles are clarified.
- Discovery
Strategize: At this stage, the vision, strategy and main goals are outlined. The benefits of incorporating alt-data are understood, evaluated and documented, while the cost-benefit analysis is completed. Success criteria for using alt-data and machine learning model are defined.
Source data: Define the data requirements, identify vendors and platforms, evaluate cost and quality of data, perform the cost-benefit analysis and select the vendors/platforms. Ideally, acquire test data sets before purchasing the license. Perform due diligence on the vendor and data sources. Collect references from other firms currently using the data sets you have identified.
De-risk: Evaluate the types of risks involved in incorporating alt-data at the infrastructure and decision-making levels. Risk analysis may cover regulatory, financial, operational, strategic and technology risks. Design and implement controls over data acquisition, usage and management. Ensure information security related to data acquisition, use and dissemination.
- Implementation
Integrate: Ingest alt-data into the technology and data infrastructure. Design the digital user interface and user experience (UI/UX), including dashboard and reporting infrastructure.
Transform: Data transformation is required to blend structured and unstructured data within the firm’s infrastructure and data preparation for its use within machine learning models.
Use machine learning: Incorporate alternative data as inputs into new or existing machine learning models. Develop methods to test both data and model quality. Validate models against potential bias.
Deploy: Configuration and development, as well as testing, is conducted during this phase. Dashboarding and reporting is set up and ready for user testing.
Evaluate: Evaluate the prediction value and usefulness of the alt-data and machine learning models against the goals and success criteria set during the Discovery phase of the project. Continuous monitoring and re-evaluation of performance is required, data quality needs to be monitored, and adjustments need to be made to reflect any changes in the environment in which the firm operates.
Outside Providers
With such specialized tools and skill sets involved with mining and distilling alt-data, many understandably outsource the function.
A few key considerations for a prospective professional services partner involve their ability to quickly integrate new solutions with existing infrastructure; cost of data feeds; and proving what they deem to be optimal, uncorrelated data sets that genuinely add quantifiable value rather than noise. An outsourced partner should also demonstrate an aptitude for key requirements of an alternative data platform, such as rapid and efficient onboarding of data sources; combining structured, semi-structured and unstructured data sets; and data preparation and normalization, among others.
Collecting and analyzing alternative data sets certainly requires navigating any number of difficulties or outright obstacles. Even so, companies that are slow to incorporate alternative data into their risk analysis, R&D, marketing, investment and other key processes assume unnecessary risk and are likely to experience system inefficiencies – which could give competitors an avoidable informational advantage.
Julia Valentine, MBA, a solution-focused FinTech Advisor to boards and management teams, is managing partner at AlphaMille, a worldwide strategy, technology advisory and consulting services firm that specializes in alternative data, multi-modal and conversational AI, software development and implementation, data analytics and management, model valuation and more. She may be reached at www.AlphaMille.com.