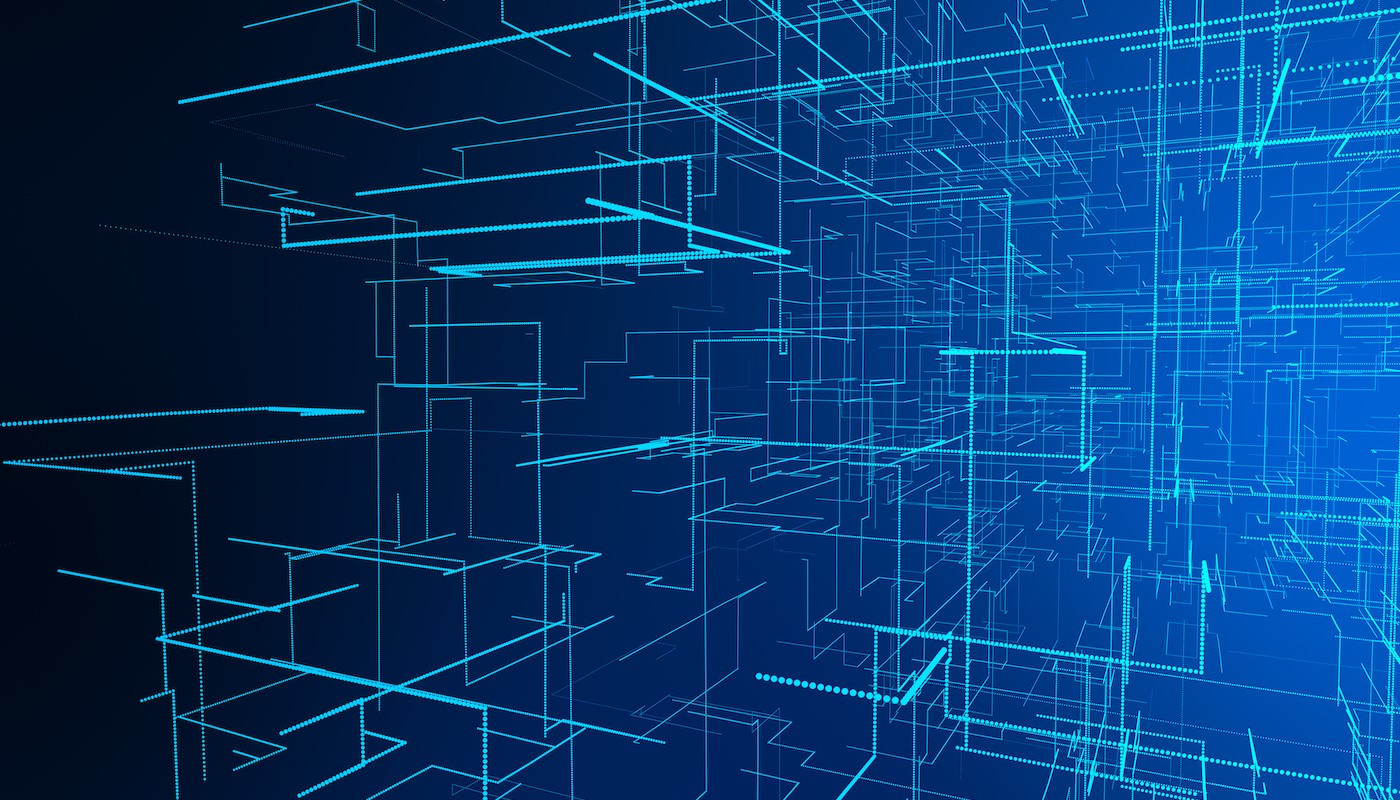
Advances in natural language processing (NLP), a form of artificial intelligence that companies like Facebook and Google have applied in online search and voice recognition, are helping to accelerate decision-making in risk management and other analytical financial functions.
The technology, which can enable machines to ingest and extract meaning from unstructured datasets hundreds of times faster than human readers, is getting a commercial boost from companies such as Amenity Analytics, Behavox, Eigen Technologies and Scienaptic. They bring expertise in machine learning and neural networks as well as proprietary NLP models specific to finance. They often make use of new, open source NLP tools, coming out of Big Tech, that are enhancing the precision, scale and speed of risk management and compliance processes.
“NLP technology is starting to touch many aspects of finance, thanks in part to advances in computing power and machine learning capabilities,” says Robert Meyer, co-director of the Wharton Risk Management and Decision Processes Center, University of Pennsylvania. He believes NLP's use as a risk management and compliance tool will only grow over time.
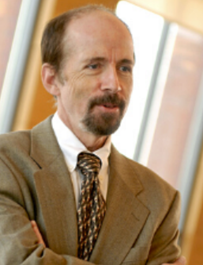
“A lot of the state-of-the-art research in natural language processing comes from firms like Google,” says Georg Kucsko, head of machine learning R&D at Kensho, an artificial intelligence start-up that is now part of S&P Global. Technology inherent in the Google search engine, Kucsko notes, likewise “underpins a lot of NLP usage in finance” and allows for greater sophistication in NLP's ability to extract signals or identify disparate connections or inferences in documents.
Both open source and proprietary advances in NLP technology facilitate a range of activities: text extraction and summation, voice surveillance of traders and other employees, compliance or fraud monitoring, sentiment analysis in social media and other communications, and analyses of CEO statements and Q&A sessions during earnings calls.
NLP took a forward leap last year with the open-sourcing of GPT-3, a model enabling machines to better understand contextual relationships between words. Resulting capabilities, such as converting natural language into programming code and translating verbal descriptions into graphs, could free up risk managers to attend to more demanding tasks.
Saving Time
“The use of NLP technologies is helping to reduce time spent on information-gathering tasks by 20% to 50%,” says Bryan Richardson, senior knowledge expert and chief data officer of McKinsey/Risk Dynamics. He adds that the tools are meant to augment, and not replace, risk managers' decision-making: “You still need a human risk manager to investigate and work through problems when they arise and assess things in a nuanced manner.”
Amenity Analytics CEO and co-founder Nathaniel Storch describes environmental, social and governance (ESG) information as a “firehose,” an example of “too much information” for risk managers to handle. Amenity's platform can take “the millions of ESG articles that appear every day and make the information manageable,” he explains, by identifying and flagging disclosures that can be market-moving.
Amenity also offers an NLP-powered disclosure deception model to indicate if there is a gap between what an analyst is saying about a company and what the company is willing to disclose.
“Our view is that companies that are run professionally and that adhere to the highest level of governance will have a clear discourse with the analyst community,” Storch says. “Others have a constant gap between what information Wall Street wants and what they are willing to disclose.”
Behavioral Deviations
Behavox's software allows firms to aggregate, analyze and act on internal data - including online chat, emails and voice -- for compliance and risk management purposes. According to Fahreen Kurji, head of customer success, EMEA and APAC, Behavox's holistic detection approach involves the aggregation of all company communication data, the building of employee relationship maps, and an assessment of all employees' behavioral patterns.
This includes how employees may display stress or excitement or deviate from normal behavior. NLP models layered with machine learning are used in identifying such deviations.
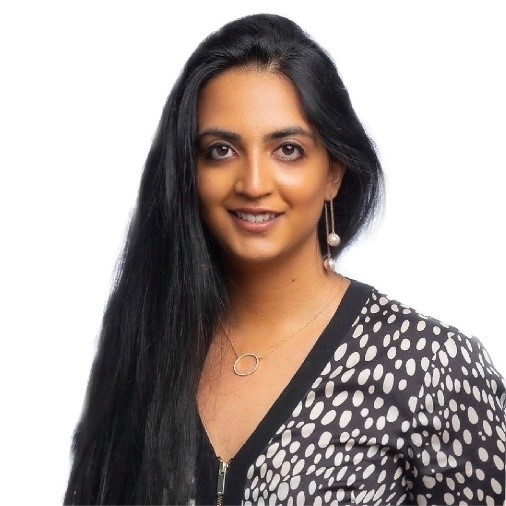
The models' sentiment analysis is critical, because “the sentiment you display when you are speaking, whether you are angry or upset, shouting or dominating a phone call,” may be indicative of bad behavior, Kurji says. “These are NLP capabilities that were not possible five years ago.”
She emphasizes that Behavox layers NLP models with supervised rather than unsupervised machine learning capabilities, so “we are never a black box for our clients, and we are able to justify why the NLP model highlighted a particular action.”
Reaching the Underbanked
Scienaptic, whose credit underwriting and fraud detection platform is powered by AI, machine learning and NLP, is led, and was founded, by Pankaj Kulshreshtha, a former chief risk officer of GE Money in the U.K. The company's general manager of credit risk solutions, Vinay Bhaskar says NLP automation can vastly expand the population served.
“In other countries across the world, where many applicants are underbanked and do not have a historical credit record, NLP models can be applied to text messages to create signals that predict the borrower's credit risk and allow you to underwrite loans in an effective way,” Bhaskar says.
“We have done a proof of concept of this based on the frequency of words used, the sequence of words, and the punctuation given,” he says, which can be applied for underwriting purposes in markets like Asia where such systems are permitted.
For fraud detection, when someone notifies a financial institution to report a lost credit card and request a replacement, an NLP system assesses the voice call or text to determine if it may be fraudulent.
Efficiency in Training
Eigen Technologies employs a combination of AI, machine learning and NLP for a range of risk-related challenges including the shift from Libor to alternative reference rates in transaction documents and analysis of credit agreements across multiple systems.
Founder Lewis Z. Liu based the firm's original Document AI platform on research he conducted as a Ph.D. student at the University of Oxford, and subsequent refinements have resulted in what he calls “small-data machine learning.” Thus, NLP-powered models may be trained on as few as two to 250 document samples, rather than 10,000, in enabling the platform to analyze and effectively respond to complex questions about derivatives contracts and loan documents.
“What's truly innovative is that even with small amounts of training data for our NLP models, you can increase the accuracy rates to the point where a customer is comfortable with not having a human review the answers,” Dr. Liu says.
Guarding Against Bias
In some instances, NLP technology appears to be advancing ahead of financial regulatory requirements or fair lending laws in the U.S.
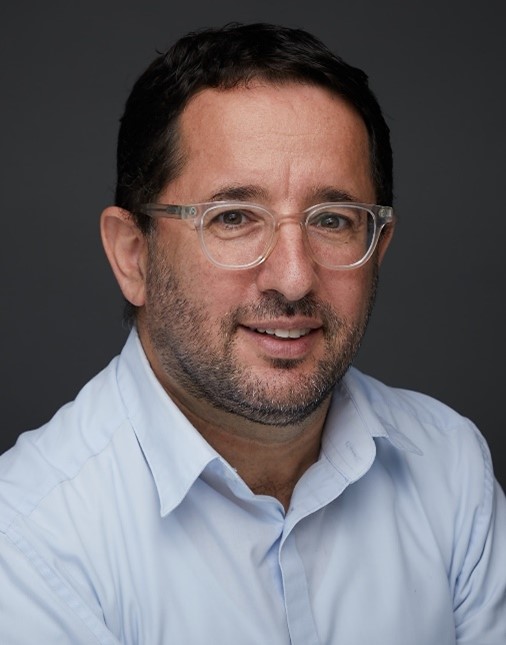
When Words Sweat: Identifying Signals for Loan Default in the Text of Loan Applications, a paper co-written by Columbia Business School professor Oded Netzer and based on an NLP analysis of 120,000 loan requests submitted to marketplace lender Prosper, concluded that consumer text can accurately predict whether a loan is likely to be repaid. The words in a loan application “are much like body language,” Netzer says. “It allows us to identify state of mind and traits of individuals who are writing about the future,” with predictability above and beyond that of a conventional FICO score.
However, Netzer says, the legality of such models is uncertain in the U.S., and there is a risk that gender or racial biases will be built in.
There are other challenges that come with NLP models. Richardson of McKinsey says they “are not good at general reasoning or problem solving. That is what risk managers do well.”
What's more, risk managers need to regularly assess and monitor NLP models to keep unwanted biases from creeping in.
Bhaskar of Scienaptic adds that “NLP is still in the evolutionary stage where the ability of the models to understand context is still far from the stage I would want it to be,” says Scienaptic's Bhaskar. “Caution is to be highlighted.”
Still, says Storch of Amenity Analytics, “As a field, there are improvements going on all the time in terms of the question-and-answer systems being developed and different levels of natural language understanding. I am excited to see what we can do next.”
Katherine Heires is a freelance business journalist and founder of MediaKat llc.