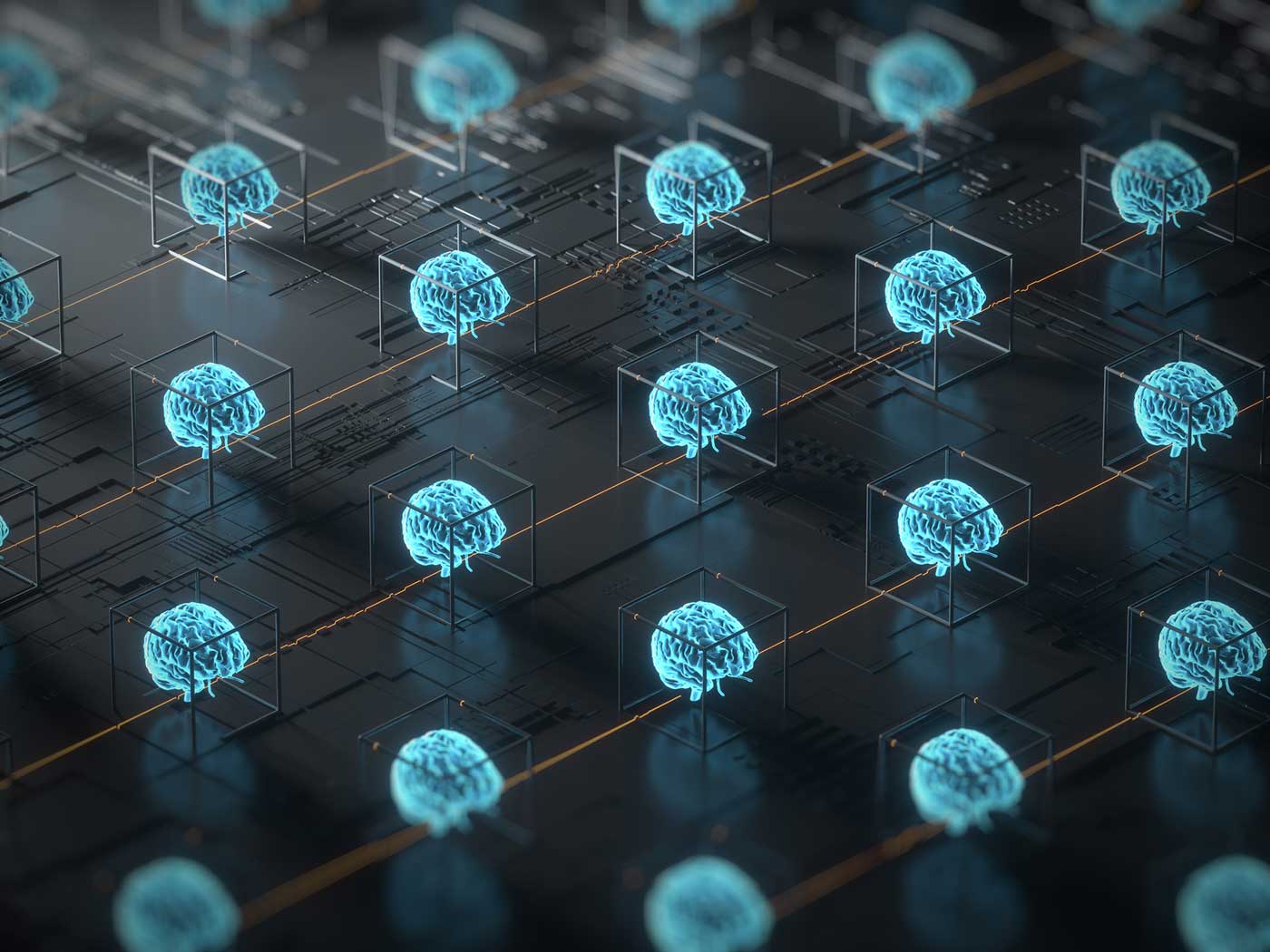
In the latest Star Wars film, “The Rise of Skywalker,” a droid robot is summoned more than once to ingest and sort through vast volumes of documentation to identify and extract critical data that helps the central characters win their battles in a galaxy far, far away.
It's not just science fiction. Advances in natural language processing, or NLP, are putting that kind information-sifting and insight-generating capacity within reach of corporate enterprises, promising significant efficiencies and time savings in risk management, compliance and other functions.
“NLP technology is a machine that enables people - and risk managers in particular - to do more things quicker and better and frees them up to focus on higher-value activities,” says Warren Breakstone, managing director of data management solutions at S&P Global Market Intelligence.
An example of NLP in everyday use, he says, is Facebook's trending topics data extraction and organization tool.
NLP is embedded in Bloomberg's news and financial information network. “Our engineering teams have built state-of-the-art NLP technology for core document understanding, recommendation and customer-facing systems,” including a significant investment in “tools that simplify client interaction,” the company says in the Tech at Bloomberg section of its website.
Robimon Varughese, head of technology and data analytics for Accenture's North American compliance consulting practice, calls NLP “one of the predominant technologies of our time that is changing how we interact with computers.” Voice recognition technologies are demonstrating NLP's ability to improve and streamline the human-computer interface.
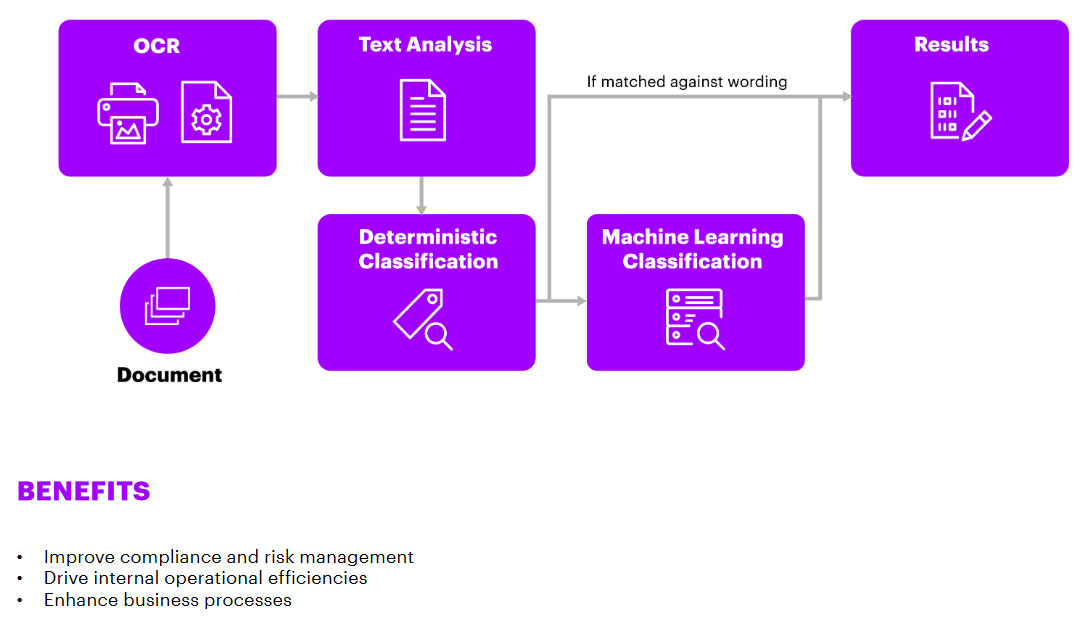
Structured versus Unstructured
A subset of artificial intelligence, NLP leverages computer processes and statistical rules to analyze and make sense of unstructured data, whether written or spoken. It solves translational complexities far beyond those presented in structured or standardized documents or databases, and can work in any number of formats: plain text, email messages, video, audio, social media, web pages, as well as financial reports, SEC filings, trader and broker conversations, phone calls and online chats.
“It's getting more and more complicated in terms of the number of channels that financial firms have to monitor for regulatory purposes,” says Steve Logalbo, director of the communications surveillance practice at compliance solutions provider NICE Actimize. “That's why they increasingly need NLP technology, combined with machine learning capabilities, to help be more efficient in these efforts.”
Indeed, when combined with machine learning, NLP or NLP-powered algorithms can analyze inputs quickly and, over time, get better at discerning deeper or more precise meaning, context and tone.
Boosting Business Intelligence
Knowledgeable observers say there is a considerable range of ways in which NLP can support risk management.
According to Accenture, in Top Natural Language Processing Applications in Business: Unlocking Value from Unstructured Data, the technology can be applied in detecting insider risk, fraud and money laundering; conducting sentiment analysis with employee, customer and investor communications; and speeding up the analysis of text, documents or voice interactions to extract critical data.
“Analyzing structured data alone is no longer enough,” Accenture concludes. “Sophisticated business analyses, predictions, and decision-making all need more.” Use cases “show how unstructured content can be used to unlock tremendous new insights. With a well-implemented NLP solution in place, organizations can enable a deeper understanding of unstructured content, providing enhanced BI [business intelligence] and analytics.”
Research in 2017 by Toronto-based Global Risk Institute said Lloyds Banking Group was using NLP with machine learning to identify fraudulent phone calls, while Deutsche Bank was improving quantitative investing models and stock price predictions.
PanAgora Asset Management has said it uses NLP technology to analyze how companies publicly discuss management of environmental, social and governance risks, as a measure of their commitment to sustainability.
Institutional investment platform Liquidnet in June 2019 acquired Prattle, an NLP company that is now part of Liquidnet Investment Analytics.
“Prattle is yet another powerful tool for asset managers to discover actionable insight from the enormous amounts of unstructured data that is produced throughout capital markets,” Brian Conroy, president of Liquidnet, said at the time of the acquisition.
In a recent Wall Street Journal article, Prattle founder and president Evan Schnidman said that clients were using the tool as a “sanity check” in analyzing central bank policy statements.
Detecting Patterns
Smarsh, a specialist in communications compliance systems, has announced that it can offer the benefits of NLP technology through a partnership with Brainspace. According to Smarsh vice president of business development Adam Miller-Howard, “Customers can harness Brainspace's machine learning and natural language processing to visualize their communications data, revealing hidden themes and relationships between various messages, terms and participants. In this way, in-house discovery teams can quickly uncover hidden patterns in their data to reduce the need for expensive e-discovery software and outside counsel.”
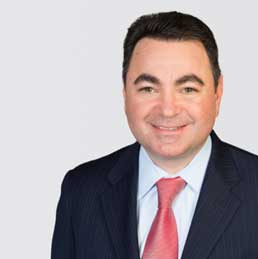
Also in the compliance arena, Naeem Siddiqi, senior adviser for risk and quantitative solutions at analytics provider SAS, sees NLP as useful in model governance and enabling firms to analyze hundreds of pages of regulatory documentation, looking for key words and references to streamline the compliance process.
Others, Siddiqi says, may employ NLP to derive insights from financial statements and annual reports. It can analyze hundreds of online reviews about a small business to help create a credit risk rating, or filter through hundreds of online news reports as part of a credit assessment of a corporation. In turn, risk managers are using the technology to assist in building collection or credit risk models.
“Reading unstructured data using NLP to derive very basic information is quite common, but others may also employ more advanced NLP to derive the intrinsic meaning of sentences and words, beyond just identifying or counting them,” what some now call natural language understanding, or NLU, Siddiqi says.
Some insurance companies employ the technology to assess accident reports for specific words with negative or positive connotations, or specific patterns of words that are correlated with fraud. They may also take into account an individual's tone of voice and other speech characteristics to detect deception.
“There are high false positives in these approaches, but this is where the industry is headed,” Siddiqi adds.
Establishing a Risk Score
Sabina Munnelly, a managing director and leader of Accenture's Innovation Center for Finance and Risk in New York, says that in partnership with Hoboken, New Jersey's Stevens Institute of Technology, the firm has been helping clients use NLP in combination with other technologies for threat detection purposes.
“We have been extracting information from emails, looking for code words from a trade surveillance perspective and then merging those risks with internal risk concerns,” Munnelly says. She adds that results get more interesting when they merge choice of language and word-pattern assessment with sentiment analysis.
The technology can tell if people are happy or sad and establish a baseline of behavior, allowing comparisons with episodes of anger or volatility. Based on such data, Accenture has established and patented an employee risk score system.
“When looking across the data, a flag is raised, and that's when a decision can be made about whether further investigation needs to be done,” she said, allowing for a more proactive, rather than reactive, threat analysis process.
Respecting Privacy
Accenture keeps in contact with regulators regarding their current thinking on employee monitoring and surveillance.
“What they are trying to figure out is the balance between threat and potential risks versus the individual's privacy,” Munnelly says, noting that while businesses are on high alert for internal threats, they are cautious and protective of their employees.
Varughese of Accenture stressed that most of the data collected is used to inform, rather than make a final decision, about an insider threats. “It gives people in surveillance or compliance the ability to look at more risk-prone people, versus simply looking at oceans of data,” he says.
Yet another application of NLP involves analyzing external data to determine if a potential or current client may be engaged in money laundering. This amounts to real-time scanning of information about limited liability entities, coupled with social and media data to advance the investigative process. “NLP can help us to obtain a 360-degree view of all the [entities], securities and subsidiaries” in question, Munnelly says.
Libor Transition and Other Finance
Accenture is working with clients on intelligent document analysis, powered by NLP, to search through documents that employ Libor, which is used to benchmark upwards of $300 trillion in financial contracts and which regulators want to see replaced by a new set of risk-free rates by the end of 2021.
“To find these documents, you either have to keep employing more people to look for them, or you can run NPL technology in combination with machine learning to look at all the documents with Libor references and find the exposures,” Varughese explains. “One particular loan transaction might have 90 different documents associated with it and so, the use of NLP eliminates all the mundane work.”
Elsewhere in finance, Breakstone of S&P Global Market Intelligence says that over many years of compiling corporate earnings calls, his firm has meta-tagged all of them and can effectively employ NLP to compare and analyze sentiment - the positive and negative connotation of the spoken words.
He notes that clients will use the NLP product to obtain an S&P's proprietary sentiment analysis score of a call or, in some instances, will use the score to compare or benchmark their own assessments.
“We are less concerned about the prepared remarks on these calls, and far more interested in the Q&A,” Breakstone says, to get from less-rehearsed parts of the presentation indications of analysts' sentiment and, from executives' voices, their confidence levels.
“You can score earnings calls in a number of ways, in their entirety, by individual section, and then start to compare a present-day call with past performance to assess risk,” says Michael Patton, vice president, data management solutions at S&P Global Market Intelligence. He also sees some companies using NLP-derived scores to assess CEOs' and CFOs' presentations and what they can do better to respond more positively to analysts and better drive company performance.
“One client used NLP to gauge the time it took for a CEO to reply to questions,” says Patton. “If more than three seconds, they used it as a trading signal. It may be far-fetched, but this client had back-tested it and that was their theory.”
Diverse Sources and Uses
Investment managers, banks and insurance companies are looking to NLP to produce better risk models, and corporations in various sectors are starting to use such tools to support risk management decisions.
“The application of NLP tools on top of other forms of data - news articles, web site ratings, social media comments - can help to anticipate problems with supply chain vendors,” says Breakstone, leading them to take steps to diversify their suppliers and partners.
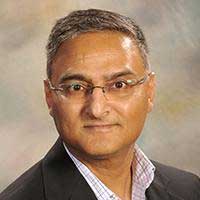
With NLP applications in finance rising in number, start-ups are springing up. One, Toronto-based “culture analytics” firm Receptiviti, applies NLP to linguistic patterns that psychological research says can be indicative of excessive risk-taking, as well as cases of satisfied workers and high productivity levels. Other start-ups assisting with a range of NLP applications include Cortical.io, Pool Party and Smart Logic.
Feedstock, which in January raised early stage financing, touts NLP and deep learning for both compliance purposes and investment insight in capital markets.
“Decisions should not be made with discrete textual data,” S&P's Breakstone advises. “They are most effective when NLP data comes together and is combined with one's own experience and expertise” - in other words, augment NLP with other insights.
Accenture's Munnelly has several pieces of advice for risk managers. They should, for example: employ model data that is clean and in digital format; understand that not all data can utilize the same NLP model, and that there are different types of NLP (such as syntactic, semantic and pragmatic); have enough data sets to effectively train NLP models, recognizing the importance of historical data; rely on human monitors to periodically review findings and speak up if a model needs to be optimized or adjusted; and carefully select ontology or data definitions, so that data tags are carefully matched to the definitions and misinterpretations of context are avoided.
“My message to risk managers,” Munnelly says, “is that you can employ NLP technology to drive more efficiencies into your business, but you should also use it to inform your risk indicators.”
A key takeaway from Accenture's 2019 Global Risk Management Study, she notes, is that new and emerging risks impacting the financial services industry are ever increasing. “Using NLP technology to be able to better inform this landscape is a critical skill for risk managers,” Munnelly says, “allowing them handle all the emerging risks.”
Katherine Heires is a business journalist and founder of MediaKat llc.
Article Updated: November 17, 2021