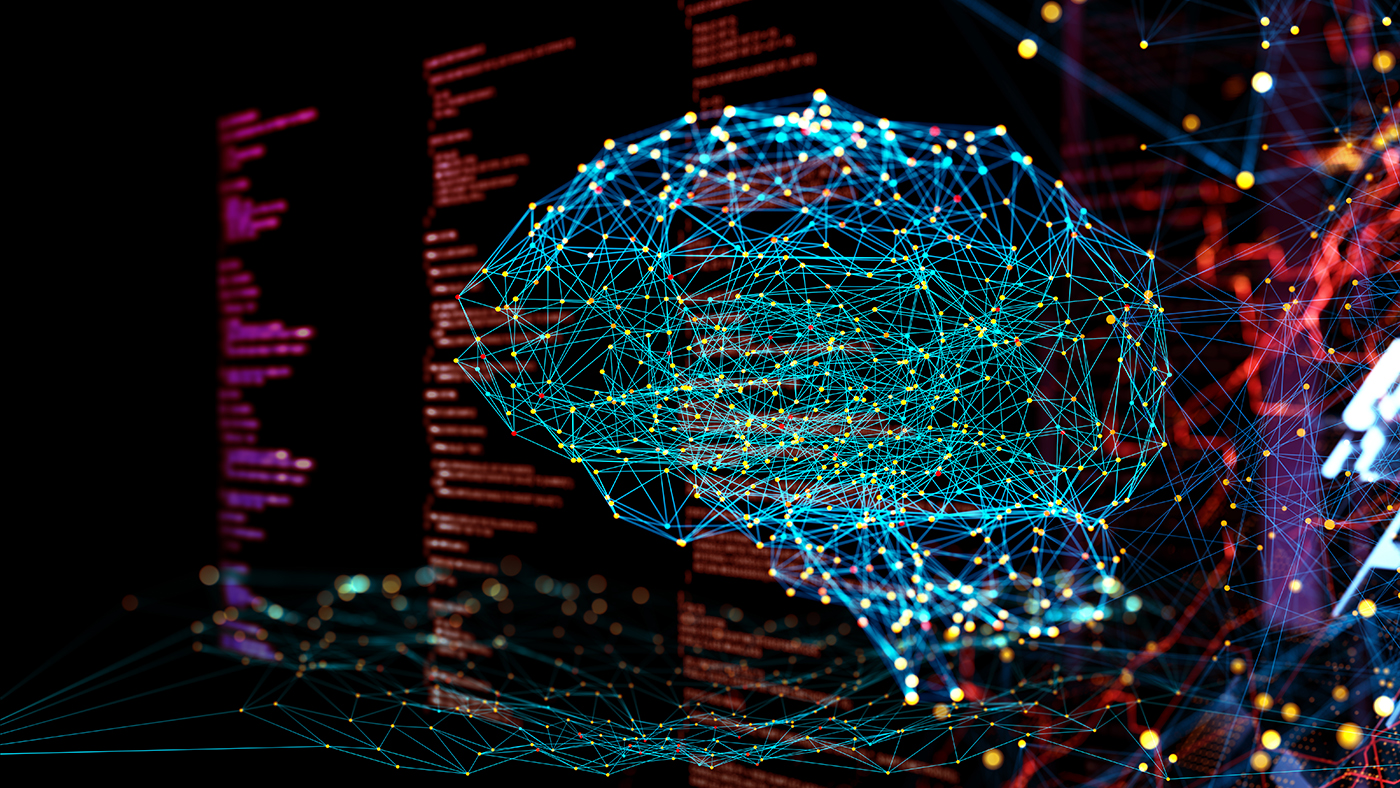
Machine-learning (ML) models have become increasingly prominent within financial services firms, and their adoption is unlikely to slow anytime soon.
What does this mean for the employment prospects and necessary skill sets for risk managers? How can they navigate the complex challenges emerging from disruptive technologies?
Professor John Hull
To help answer these questions, we spoke with John Hull, Professor of Finance at the University of Toronto’s Rotman School of Management. Hull, the recipient of the 1999 Financial Engineer of the Year Award from the International Association for Quantitative Finance, currently teaches ML to graduate students targeting financial careers. His book, “Risk Management in Business: An Introduction to the World of Data Science,” is used in the courses he teaches.
Tod Ginnis (TG): How can early career or aspiring risk managers prepare for a future that incorporates ML into their work?
John Hull (JH): Risk managers don’t need to become data scientists, but they will need to understand how the technology works and how to explain it to others. It’s important to have the technical skills to know what data scientists do and to ask the right questions. You want to know enough about the tools to know when they are appropriate and when they are being abused.
I like to make an analogy with accounting: we teach accounting to our MBA students not because most will become accountants, but so that they can work productively with accountants. Similarly, future risk managers need to know enough about machine learning to work productively with data scientists.
Many libraries have been developed in Python for implementing machine-learning algorithms. I require the students in my machine-learning courses to learn enough Python to do illustrative assignments. Python is becoming almost as prevalent as Excel in financial institutions, and aspiring risk managers should definitely consider learning it.
TG: As some functions of the profession become increasingly automated, how do you envision the role of risk managers working with ML?
JH: I believe we’re in the early stages of a fourth industrial revolution, which includes AI, ML, and creating intelligence from data. There are clear risks associated with it.
Can we maintain complete control over the intelligence we’re creating? It will be years before we know. What we do know is that it’s important to have human beings in charge.
Some people say that if you have a good ML model, you can just let it go and fire everyone who used to do the job. But oversight is important. Periodically, you must confirm your model is still appropriate.
ML uses historical data, and when we run into unprecedented situations — like the pandemic — the data may no longer be appropriate. It is important for risk managers who understand machine learning to periodically review the appropriateness of the models that have been developed.
TG: In what ways do ML models fall short in meeting an institution’s goals regarding risk management?
JH: Explainability is a big issue. Consider a credit risk model deciding loan approvals. The person using the model must understand in general how it works. It is also important to be able to communicate the results of the model to the potential borrower, especially when the loan is refused. It's not enough to say, “the algorithm says no.” You’ve got to explain the decision in normal language.
Bias is another challenge that can occur in multiple ways. It can relate to the data that you've used to build your model or biases on the part of the model builder.
Biases can also result from the features you've used as inputs to the model. Using race and gender are obviously unacceptable, but you must be careful, because the model might include features that are highly correlated with race or gender. So, even though you haven't explicitly used them as features, you could be introducing bias into your algorithm.
Another key factor is what statisticians call testing the model in-sample vs. out-of- sample. The danger is that somebody develops a model and says, “the model fits the data really well. Let's use it to make predictions.” That type of model may work great on the data that's been collected, but it hasn't been tested on new (i.e., out-of-sample) data.
There are procedures for using ML in risk management that try and overcome this problem. It's important that the risk manager understands those procedures and asks whether they've been followed properly. If the data scientist hasn't tested out-of-sample, then you shouldn't use the model.
TG: What specific skills or qualifications might make a new risk management hire appealing for financial institutions looking to better utilize ML?
JH: One thing we emphasize to our students is that communication skills are critical. And technical skills are becoming more important. Demonstrate that you've got some of these technical skills (like expertise in Python) we've been talking about.
Taking the FRM is another good idea. There are two chapters in the FRM study materials on ML that I assisted with. Working through those chapters will help give future risk managers the tools they need to work effectively with data scientists.
TG: What advice do you have for early career and aspiring risk managers who might be unsure if they’re ready for a brave new world filled with advanced technology?
JH: I remember the 1970s and the 1980s, when we were going through the third industrial revolution, namely digitization and computers. Some people embraced the changes and studied them. Others put their head in the sand. The people who did well in business tended to be those who took on the challenge. Most didn’t become programmers, but they learned how to use computers.
Risk managers should learn enough about the technology to understand what the data scientists are doing and how it may impact the organization.
Risk managers can be important intermediaries, because it’s part of their task to understand all the models the organization uses. As questions arise about particular models, they should be able to explain things in clear language to all the relevant stakeholders, including senior management. This will make the job of a risk manager increasingly important, as the algorithms become more complex.
Tod Ginnis is a content specialist at GARP. He is the author of a GARP blog that is aimed at early-career risk managers and professionals aspiring to earn their Financial Risk Manager (FRM)
Article Updated: June 7, 2023
Topics: Risks & Risk Factors