In recent years, attention has increasingly turned to the promise of artificial intelligence (AI) to further increase credit availability and to improve the profitability of banks and other lenders. But what is AI?
The most accessible way to answer this is by reference to the Turing Test. In its standard formulation, the test involves a human observer interacting with a computer and another human being. If the computer can fool the observer into thinking it is actually human, the test will be positive for AI.
However, I argue here that the test doesn't work for the risk space. We don't really want credit‐decisioning systems to be truly human, mainly because human reasoning is insufficiently transparent.
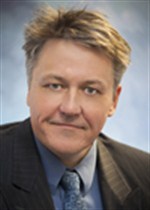
If you go back a few decades, credit decisioning was primarily based on patronage and presentation. Those who desired a loan would apply to their local bank manager for funds and the manager would decide whether to grant the applicant's wishes.
The decision may have been backed by some financial analysis, based largely on assertions made by the applicant, but would have also been based on whether the manager liked “the cut of the applicant's jib” and fit the manager's view of what a sound debtor looked like.
Good bank managers may have performed reasonably well under such a system, but bad managers would have missed solid business opportunities and dashed the hopes of many a deserving applicant. The inefficiencies and inequities of this approach hardly need to be catalogued here.
Over time, regulatory and consumer pressure and the development of sound statistical methodologies have gradually improved this system. These days subjective assessments of the applicant's jib play a small role for some lenders, while most apply a modern “moneyball”‐style statistical assessment of the profitability of lending to particular individuals and businesses.
In the credit space, we are not concerned about whether the computer thinks MÖtley CrÜe rocks harder than Metallica. We only care about the nature of the loans it chooses to fund, the profits that stem from the loans funded and the reasons it gives for accepting or rejecting certain applications.
Applying the Turing test, if the robot can fool us into thinking that it is human based only on its responses to these factors, does this necessarily imply that it is “intelligent” when viewed through a credit‐oriented lens?
AI vs. Humans
To explore this, consider two hypothetical mortgage lenders. The first exclusively uses old-school, “cut‐of‐the‐jib‐style underwriting, while the second relies exclusively on the FICO score to rank prospective loan applicants. In this analogy, the FICO‐based methodology constitutes the approach we are testing for artificial intelligence.
If we were simply trying to identify the human in this game, it would likely be straightforward. When pressed for a reason for rejecting a particular application, the robot would offer dry assessments about there being too many recent credit applications or cite a particular short‐term delinquency gleaned from the credit report. The human's reasoning would be more idiosyncratic, focusing on things like the applicant's demeanor when being interviewed.
If, however, we measure “intelligence” using only the risk‐adjusted profitability of the resultant portfolio, the FICO‐robot is probably much smarter than its human adversary. This could be due to increased speed of the decision process, or a decrease in the per‐application cost of actually making the decision. The computer may not be making better decisions than the human, per se, though it probably is. Either way, the bank's shareholders are likely to prefer the more automated approach.
We next turn to a more realistic framework, where the human player is also given access to the FICO score and can use it as they see fit. In this case, so long as the human is good at their job, they will often be able to achieve higher profitability than the robot that exclusively relies on the credit score. The human being, in this case, is doing something intelligent that allows them to beat the brute force statistical methodology.
If a proposed technique can uncover these insights and thus reduce the number of instances where the evaluator overrides the score, it is displaying a form of credit‐focused artificial intelligence.
This is clearly much weaker than the classic Turing Test. The FICO score would resoundingly pass this modified test, but it would not be able to fool a human observer into thinking it was human. This is a good thing.
The Future of Credit Decisioning
The history of the statistical development of credit scores has involved the gradual removal of human emotion and prejudice from the lending decision. The promise of true AI is, in some ways, the reverse of this. It is trying to automate the emotional intelligence of a successful lending officer of the old school to augment the now standard credit scoring methodologies that have become so successful in intervening decades.
If this is done in good faith, with high levels of transparency, there is no reason why AI advances could not be as great as those made by early credit score developers, even if they fail the classic Turing Test. AI researchers are simply looking for new sources of data and new statistical techniques that hold the potential to improve the efficiency and profitability of the lending business, just as their forebears did. If they can be shown to be helpful, they will be widely adopted, and society will be richer for their efforts.
If, on the contrary, the complexity of AI is used as a smokescreen for a return to the bad old days of banking, where your credit application is rejected because the robot does not like the cut of your jib, then it will fail, either through regulatory fiat or by consumer revolt.
In banking, being human is not the right challenge for the AI revolution. Rather, credit decisioning should be dispassionate, transparent and meritocratic.
These are robotic traits, but merely aspirations for most humans.
Tony Hughes is a managing director of economic research and credit analytics at Moody's Analytics. His work over the past 15 years has spanned the world of financial risk modeling, from corporate and retail exposures to deposits and revenues. He has also engaged in forecasting of asset prices and general macroeconomic analysis.