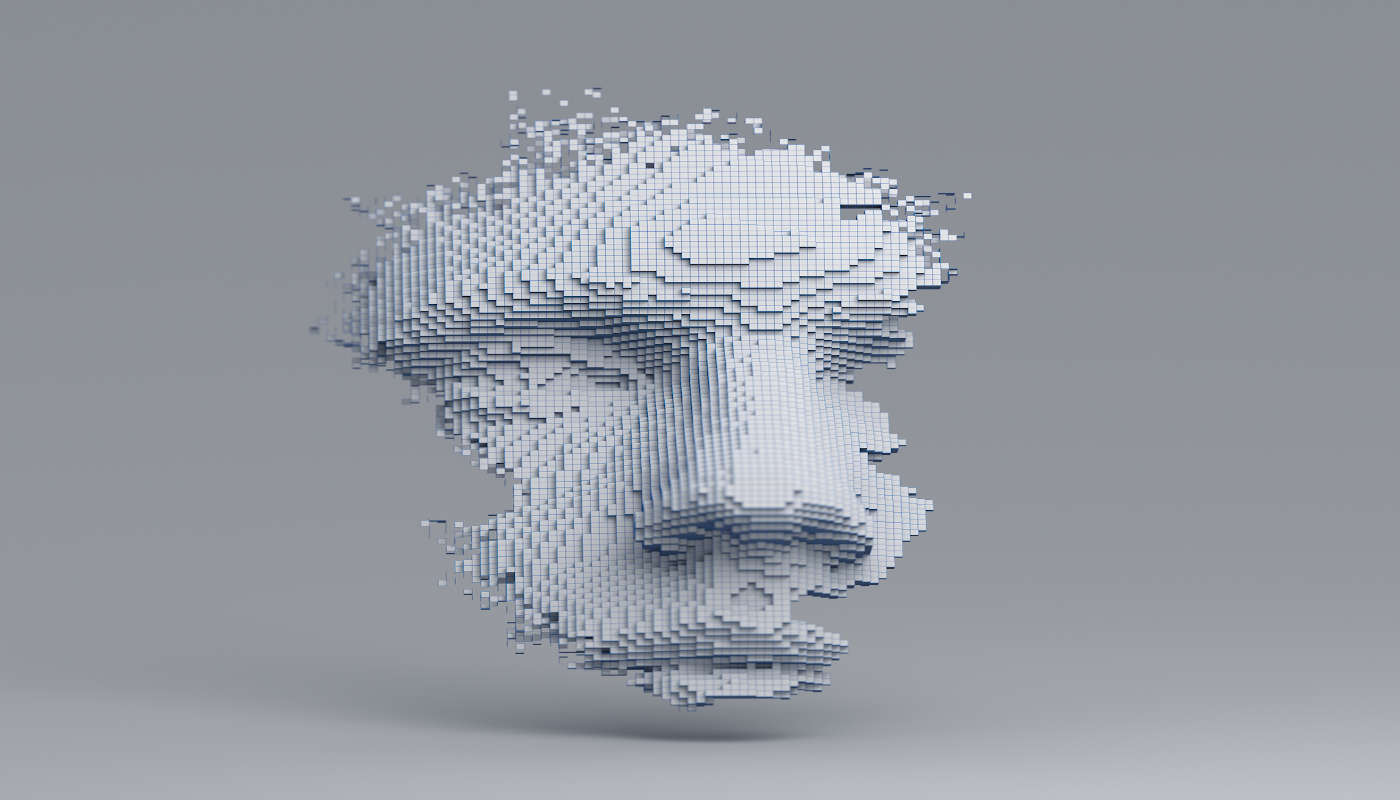
The earth is trembling below the feet of the chief risk officer, who is now facing huge risk assessment and risk integration challenges. While there are no simple solutions, artificial intelligence offers a way to make better decisions and more effectively manage today's biggest qualitative risks.
Before delving into how AI can address current risk obstacles, we must first understand the problems at hand. They are multifaceted, but cover four key issues.
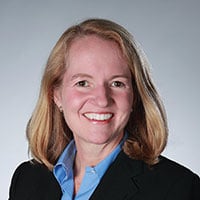
First, in the wake of the Basel Committee on Banking Supervision's decision to abandon the advanced measurement approach (AMA) for bank operational risk capital, C-suite executives are now wondering what possible business value can be derived from subjective, non-aggregatable, color-coded risk-and-control self-assessments.
Second, banks are challenged to integrate a host of risks - including resiliency, third-party supply chain, IT/cyber, regulatory compliance and financial-crime compliance - into their risk frameworks.
Third, banks have had difficulty manually contextualizing risk with strategy, and very smart analytics are required to align them.
Fourth, the velocity of business change has never been faster, and will not slow.
Overcoming these challenges requires smarter data management and advanced artificial intelligence.
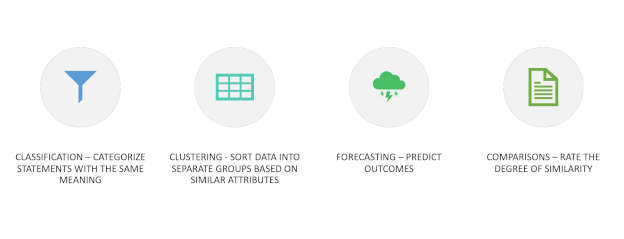
A.I. Approach
Building the right approach to AI starts with three key steps: strategic data acquisition, data lake unification, and automation/manual labor reduction. Let's now take a closer look at each.
Strategic Data Acquisition. Risk management data does not exist in a vacuum, and must be contextualized with relevant finance, HR, IT and supply-chain data. This internal data must be united with external information from suppliers, vendors, social media and experts.
All of this data must be curated to standardize the language and to recognize semantic similarities. The natural-language processing capabilities of AI can manage this task, and internal and external risk assessment data will provide a further strategic data boost.
Data Lake Unification. AI canunderstand and semantically relate, classify and cluster the natural language of the business for risk management, finance, IT, HR and other internal groups. External social media and regulatory data can be integrated with flexible APIs in a common cloud repository.
Moreover, AI can define key phrases and common ontology, integrating all elements of a firm's data lake. Deep learning, a statistical technique for classifying patterns based on using neural networks with multiple layers, can be effectively leveraged for improving analytical capabilities in risk management.
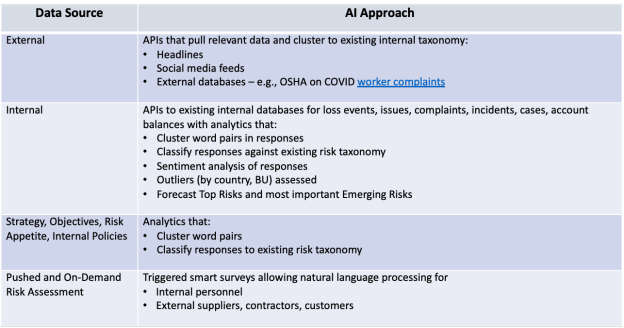
Manual Labor Reduction, via Automation. AI reduces the manual and effort-intensive tasks of making sense of unstructured data by (1) triggering risk assessment in “hot” areas of change; (2) identifying and prioritizing risk; (3) aligning strategy, business objectives and risk appetite with risk levels; (4) aligning business units to process to risks to controls to contextualize risk, aggregate risk levels and automate taxonomy changes; (5) identifying strategic and emerging risks; (6) automating the analysis of risk scenarios; and (7) performing risk impact assessments.
Moreover, patterns and priorities in complaints, cases, issues, risks assessments and test results can be proactively detected (at scale) by machine-learning applications.
The Role of AI: Reality-Based Examples
Let's now take a look at two practical cases of how AI can help banks properly measure and manage qualitative risks.
Example 1: Apply AI to Smart Surveys to Continuously Assess Risks
Leveraging analysis of anomalous relationships in the data lake, targeted surveys can be sent for completion by those closest to business growth and change. Using mobile devices, users can provide voice and touch-screen inputs.
The same approach also can be reversed, to allow internal and external users to submit on-demand risk intelligence and issue input. Key phrases can be identified, and natural language inputs vectorized, to allow the machine to detect patterns and trends and to relate key phrases to the existing taxonomy.
Example 2: Business Decision Support
The same AI-driven data management and analytics that ensure risk management can provide value to the business. These tools should expedite revenue cycles through speedier risk approvals of new and large transactions. What's more, they should give firms the ability to rationalize training requirements and to link the business process to risks and issues more transparently. (The latter could end, or at least decrease, “second-guessing.”)
These tasks are all part of the “value-added” CROs should bring to an organization. On top of meeting regulatory mandates, the CRO should strive to support business change in a manner that enhances organizational resilience in the face of competition from challenger banks. Similarly, he or she should triple-check risk controls to guarantee that correct actions are taken to ensure acceptable levels of risk, while minimizing bureaucracy.
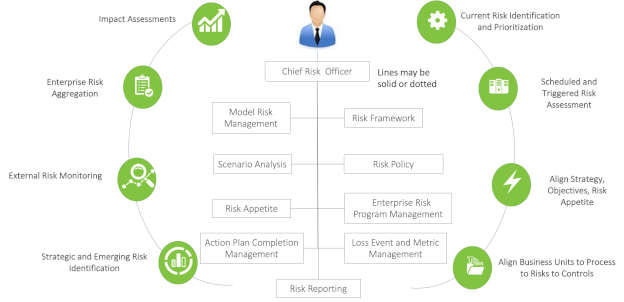
Parting Thoughts
Executive management and risk managers at banks face an urgent, two-pronged challenge: to find a real- or near-real-time method of correlating expected exposures to enterprise risks, and to determine the impact of these exposures on strategy.
Financial institutions that want to address these issues and gain an edge on competitors can use next-generation technology, like AI, to rethink and rebuild risk management.
Brenda Boultwood is an independent risk management consultant and company advisor. She is the former senior vice president and chief risk officer at Constellation Energy, and has served as a board member at both the Committee of Chief Risk Officers (CCRO) and GARP. Previously, she was a senior vice president of industry solutions at MetricStream, where she was responsible for a portfolio of key industry verticals, including energy and utilities, federal agencies, strategic banking and financial services. Before that, she worked in a number of risk management, business roles and as the global head of strategy, Alternative Investment Services, at JPMorgan Chase, where she developed the strategy for the company's hedge fund services, private equity fund services, leveraged loan services and global derivative services. She currently serves on the board of directors at the Anne Arundel Workforce Development Corporation.