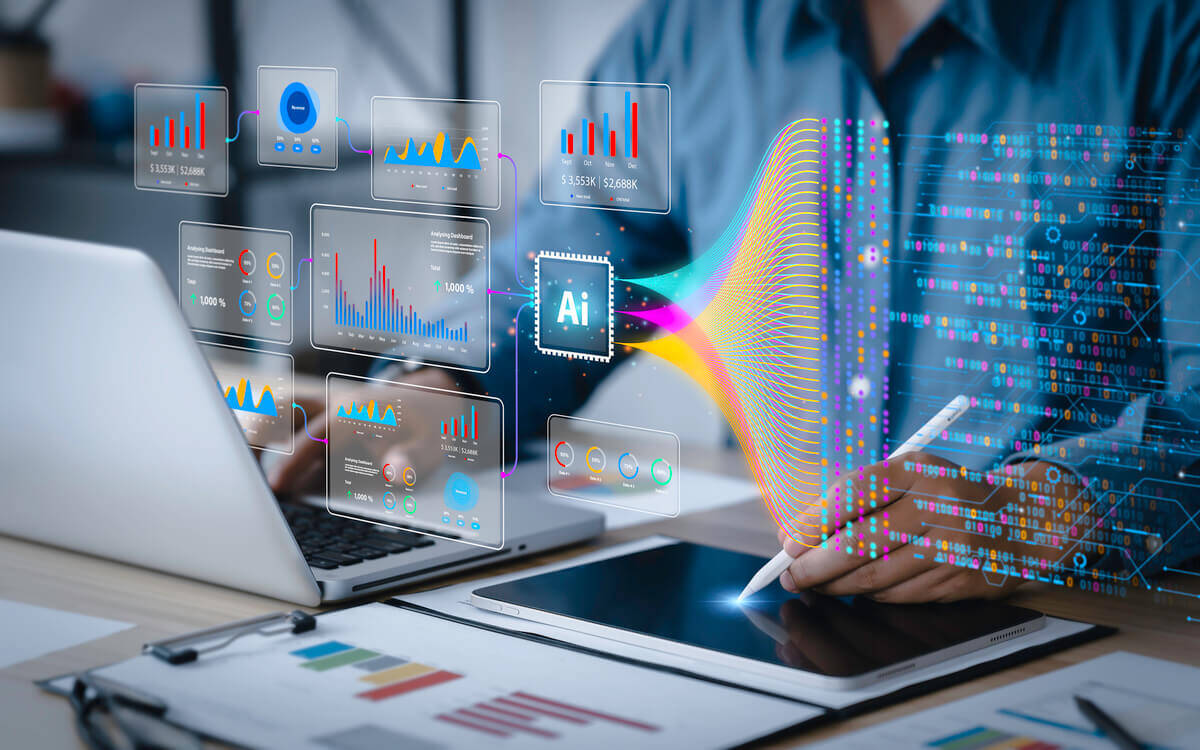
The executive title of chief artificial intelligence officer (CAIO) is gaining acceptance in the public and private sector alike, and with a holistic regard for the desired range of technical knowledge and leadership skills. (See Chief, Czar, or Committee: Who Rules Over AI?)
AllianceBernstein (AB) showed the way when it announced on July 1 the appointment of Andrew Chin as the $777 billion asset manager’s first CAIO, a move still unusual outside the technology sector.
A 27-year AB veteran who is based in New York and also worked in London, Chin had served previously as head of quantitative research and chief data scientist, and was chief risk officer for more than a decade. He was most recently head of Investment Solutions and Sciences and an operating committee member.
“This new role signifies the evolution of not only my career path at AB, but the broadening role that data science and AI are playing across the financial services industry,” said Chin, who holds a BA degree in math and computer science, and an MBA in finance, from Cornell University.
Interviewed recently for GARP Risk Intelligence, Chin advises looking at AI as a “suit of armor” which can help augment decision-making as business roles and challenges evolve. With the new responsibilities come sophisticated tools to synthesize data and recommend actions across many different types of risks.
Why were you a logical choice for CAIO?
My diverse background leading many different businesses has helped me understand how AI can transform our organization.
I have previously led our quantitative research, risk management, asset allocation and index management functions. Heading quantitative research informed me on how AI can be used to uncover successful alpha factors, to construct successful investment models, and to construct better portfolios.
AB’s Andrew Chin: “Transformation is difficult and needs to be managed well.”
As the firm’s prior chief risk officer, I developed a strong understanding of how data science tools and techniques can help asset managers better identify, manage and monitor risks. Specifically, AI can be leveraged to identify anomalies in market movements and portfolio risks.
In my most recent role, I ran a team managing $60 billion in assets. That experience showed me how AI can be used to help investment teams be more effective, with better returns, and be more efficient in managing client portfolios.
Also, my desire to build capabilities and businesses led me to AI. The asset management industry is highly competitive, and AI has the potential to transform how our industry operates, spanning across all functions in an asset management firm – from research to portfolio management, to trading, to settlements. AI can provide an edge in unlocking investment insights, help us engage with our clients more effectively, and generate operational efficiencies for our organization.
To whom do you report, and what are the expectations?
I report to the chief operating officer [Karl Sprules].
We have already achieved success in a variety of areas, including investments, distribution and operations. In the near term, we are training our staff to leverage AI, and especially generative AI, to augment their current roles. This step is critical because our employees are subject matter experts, and we believe that AI can enhance their roles.
Our success metrics over the next six to 12 months focus on the adoption of AI tools and techniques across the organization. We want to determine if teams are leveraging the new capabilities to create better investment and client engagement models, and employees using GenAI tools like Microsoft Copilot and proprietary applications to generate ideas, create first drafts of documents or check existing programming code.
Whom do you interact with most, and on what issues?
The heads of the various business units, including investments, distribution, and fiduciary functions, as well as the head of technology and operations. Use cases are driven by the real issues our business faces, so it’s critical that there is strong alignment on priorities, resources and projects.
Additionally, to scale AI capabilities across the organization, I need to partner with the head of technology to ensure that the tools and techniques we’re using are safe and well-supported. I have close collaboration with the IT organization to accelerate adoption, usage and impact.
What skills and personality traits best serve this role?
Being a subject matter expert on a wide range of business and technical competencies gives me credibility with our teams as I introduce opportunities for AI within their domains. A successful partnership starts with trust, and that foundation enables us to collaboratively test new ideas and create innovative solutions.
Another important skill is helping others to reimagine their roles and workflows. Many professionals in our industry are comfortable with roles as they’re structured today, and it may be hard to envision what the roles may look like with AI as an assistant. We face two obvious obstacles: We may not fully appreciate the broad capabilities that AI may engender; and while we have been successful in our existing roles, we haven’t delved deeply into how things can be different.
How will AI change employees’ jobs?
Before teams and employees can fully embrace or leverage the latest AI tools, they may need to transform how they run their business or conduct their activities. Real change requires transformation. It takes time for humans to understand the new technologies, to learn how they can be applied in their domains, and then re-imagine how their roles should change to leverage the new tools.
This transformation is difficult and needs to be managed well. Ultimately, we want to equip our whole organization with the relevant AI skills so that they can be more effective and efficient. This process requires significant skills in organizational behaviors and people transformations.
What are the most surprising lessons you have learned?
Some may believe that AI is magical and can somehow solve any problem. Similar to all successful models and tools, the data fed into the AI systems must be accurate, unbiased, representative and accessible. Much of the data we work with today do not satisfy one or more of those criteria, so the resulting models may not perform as well as we expect.
As a result, successful AI systems need clean and robust data. After that, we need to have patience and care in constructing the actual AI models. Models may need to be improved iteratively for them to be effective, requiring care and perseverance from the data scientists and users. Choosing the right model to use is an important consideration. AI tools shouldn’t be used simply because they’re available.
How are you and AB preparing for likely AI challenges over the next couple of years?
Training our staff to leverage the new AI capabilities is paramount. Our employees are subject matter experts in their fields, and I aim to provide them with augmented intelligence that can help them make better and faster decisions. I like to use a metaphor. “Iron-person” is a smart subject matter expert with a suit of armor that’s delivering data and synthesis very quickly. The human inside can choose to accept the recommendations or override them if needed.
My challenge becomes creating “iron-people” across the firm, building the suits of armor across different functions and empowering employees to leverage these suits to be more effective and efficient.
Another challenge is that AI tools and capabilities are improving at an incredible rate. As consumers, we need to build robust models and processes that can incorporate the latest technological improvements. For example, OpenAI may dominate the LLM [large language model] landscape today. But with several big tech companies introducing more powerful capabilities every day, the leader of the future is unknown. We therefore need to be model-agnostic in our infrastructure, so that we can incorporate the latest advances from the tech sector.
What is your advice to others about succeeding as CAIOs?
Deep subject matter expertise is critical. A deep understanding of our industry and our functions is foundational to success. AI tools are very powerful, but problems within finance are very different from other industries.
For example, within investments, the signal-to-noise ratio is very low, and existing AI tools have difficulty addressing these types of problems. As a result, our industry needs to adapt the tools to make them useful for our investment teams. Without a true understanding of our industry and the associated issues and workflows, a CAIO would be left with very powerful tools, but little impact.
Topics: Data & AI Model Governance