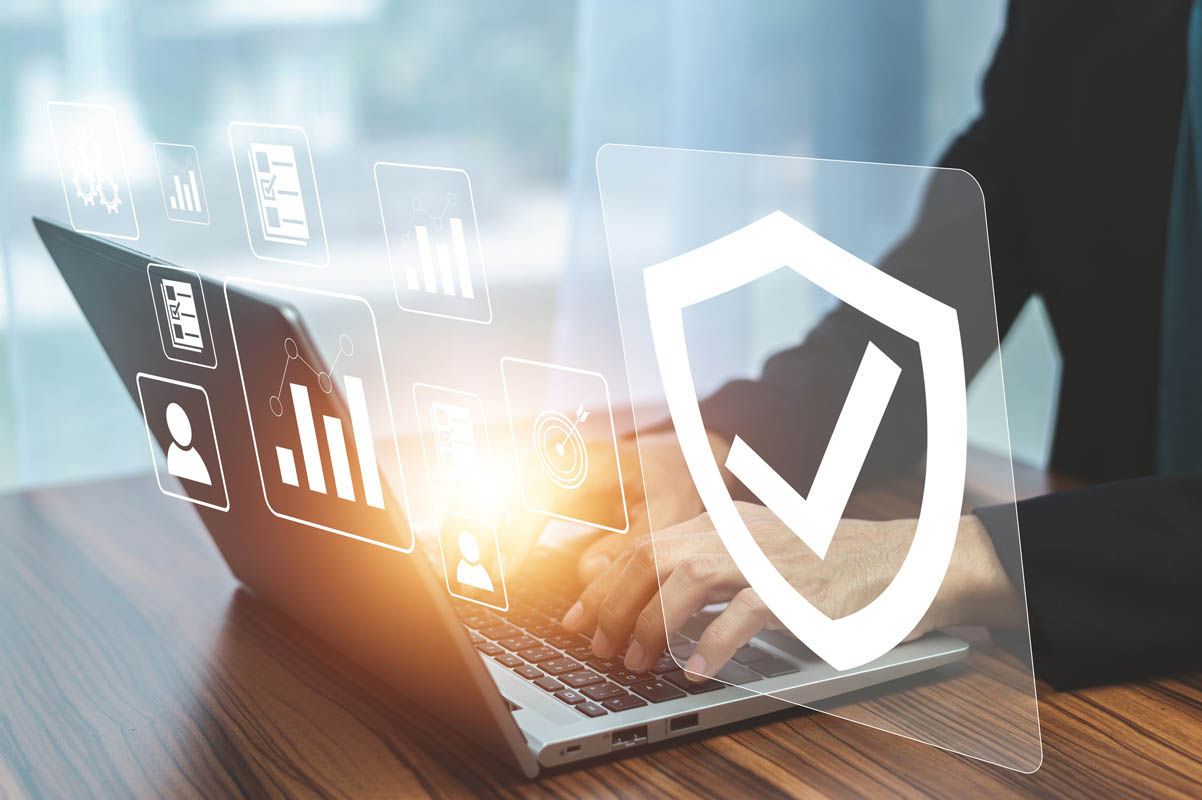
Prescribing the wrong risk management framework may inadvertently exacerbate the risks posed by artificial intelligence. For that reason, the possible “downfall of humanity” is an idea that should be taken seriously.
To explain: AI enables computers and machines to simulate human intelligence. That is the “mental quality that consists of the abilities to learn from experience, adapt to new situations, understand and handle abstract concepts, and use knowledge to manipulate one’s environment.”
Automated intelligence and generic predictive data analytics are outside scope if the computer or machine is not performing functions to “simulate the mental quality of humans.” However, AI does not need to be autonomous to be within scope. Confining AI risks to covering only artificial general intelligence (AGI) would be too narrow of a scope.
Limit the Harms
To truly understand AI risks, one should first refer to Isaac Asimov’s Three Laws: A machine “[1] may not injure a human being, or through inaction allow a human to come to harm; [2] must obey the orders given it by human beings, except where such orders would conflict with the First Law; [3] must protect its own existence as long as such protection does not conflict with the First or Second Law.” (A “Fourth or ‘Zeroth’ Law” came later.)
Kelvin To: A call for “unconventional wisdom.”
The second and third laws depend on the first one regarding the safety of a human. Due to ethical complexity, the zeroth law put emphasis on the broader humanity, rather than the individual. A bright-line test, therefore, is whether the disobedience, action or inaction of AI would impair the livelihood of human(s), exacerbate the downfall of humanity, or pose existential threats to human(s).
Rather than commenting on whether AI risks are remote or not, humankind should develop an urgency towards learning and adapting to an AI-filled environment where humans can master over it. Following are AI risk examples:
- AI drains significant energy, analogous to mining crypto, such that it could potentially bring down the energy grid. Underwater cooling and other innovative approaches could deal with unprecedented demand of data centers given the growth of AI.
The efficiency of AI should be embedded in its design. Finding a needle in a haystack to rely on “black box,” neural-network deep learning from a gigantic, centralized data vault, such as the securities market Consolidated Audit Trail, is highly inefficient. Decentralized/federated learning and analysis directly from data sources is a much better approach from cybersecurity, privacy and resource-conservation perspectives.
- AI molding people into machines or “couch potatoes.” Reinforcement models, ad-optimizing algorithms or learning methods that lead to addictive, herd and/or polarizing behaviors should be closely scrutinized. If we are against human slavery, then we should watch out for authoritarians trying to use AI to exploit or destroy humans’ abilities to think independently. Indeed, there are civic concerns about massive government surveillance.
- AI can recall every bit of big data to optimize and rationalize for speedy and accurate decisions in ways humans cannot. The irony is, if AI mimics humans like in Nobel laureate Daniel Kahneman’s book Thinking Fast and Slow – where the division between System 1 (fast, intuitive and automatic) and System 2 (slow, effortful and logical) minimizes effort and optimizes performance – then would AI have the same fallacies influenced by “loss aversion, certainty and isolation effect”?
AI has driven modern society towards the risk of hyper-optimization. Do we want consistency and act rationally every time to undermine humans’ unique ability to think laterally and/or to selectively forget things? These mental qualities reflect our human imperfections, including the usefulness of useless knowledge. So, before you wish AI to give consistent and rational answers (output reliance), or not, be careful.
- AI can exacerbate 21st-century challenges: a rebellious insurgent with a war chest to orchestrate a market-wide shake-up, global decoupling, and foreign adversaries wanting to see the U.S. engage in unhealthy competition to possibly erode its market position. In the cyberpunk era, be mindful of the gaps and differences between DeFi and CeFi. Rather than punishing all tech innovations, the ability to delineate good and bad actors is essential to mitigate this risk.
- AI is like the news media. “There are multiple versions of truth. The news, while attempting to inform, often selectively highlights certain aspects rather than recording everything in its entirety.” (See Alain de Botton’s The News: A User’s Manual.) AI “bias” can mean different models have different tradeoffs between tractability and realism. Empirical research this year by Rensselaer Polytechnic Institute and U.S. Office of the Comptroller of the Currency co-authors explored the relationship between increased model complexity and information asymmetry in financial markets. Nemil Dalal wrote in 2017 that the biggest threat to democracy is not fake news (hallucination), but rather is selective facts.
A Data Provenance Initiative has been launched to address concerns about legal and ethical risks in the AI community. What constitutes fair, reasonable, and non-discriminatory? I recommend assessing the divergence between private rights and social costs.
Regulate with Purpose
Don’t get me wrong. As an inventor of patented solutions in signal processing, ensemble learning, trading, etc., I understand why policymakers around the world are scrambling to regulate big tech and AI. Deepfake imposter scams are driving a new wave of fraud. Disinformation and privacy issues should be a concern for society and government.
If the regulatory policy goal is to promote explicability that provides appropriate contexts of AI and ensures it is fit for purpose, then there is merit in establishing relevant guidelines. However, if allowing the incapable to manage the capable and subjectively judge if AI’s “opaque or overly complex training techniques make it difficult to understand how predictions are made, which poses risks for issue-root-cause analysis and for interactions with regulators and other interested parties,” then it would be a disaster.
The foundation of responsible AI is NOT about how well an individual can articulate or reveal the secret ingredients of an AI to others. Indeed, with government gathering of information, the more people know about these AI secret ingredients, the greater the risk (e.g. function creep) will be for society.
AI can deploy countless “agents” to avert hackers. Can a “virus” to overflow the system be used as a last-resort method to prevent an AI conflict with Asimov’s laws? Or should every AI be mandated a kill-switch or circuit breaker? Stephen Hawking was one who warned of AI as an existential risk. As the technology races ahead, it will take unconventional wisdom for a “eureka” moment. Embrace difficult challenges to – in Alvin Toffler’s words – learn, unlearn and relearn in order to address AI and the risks it poses.
Kelvin To (kelvin.to@databoiler.com) is a big data and financial technology platform innovator and founder and president of Data Boiler Technologies.
Topics: Risks & Risk Factors