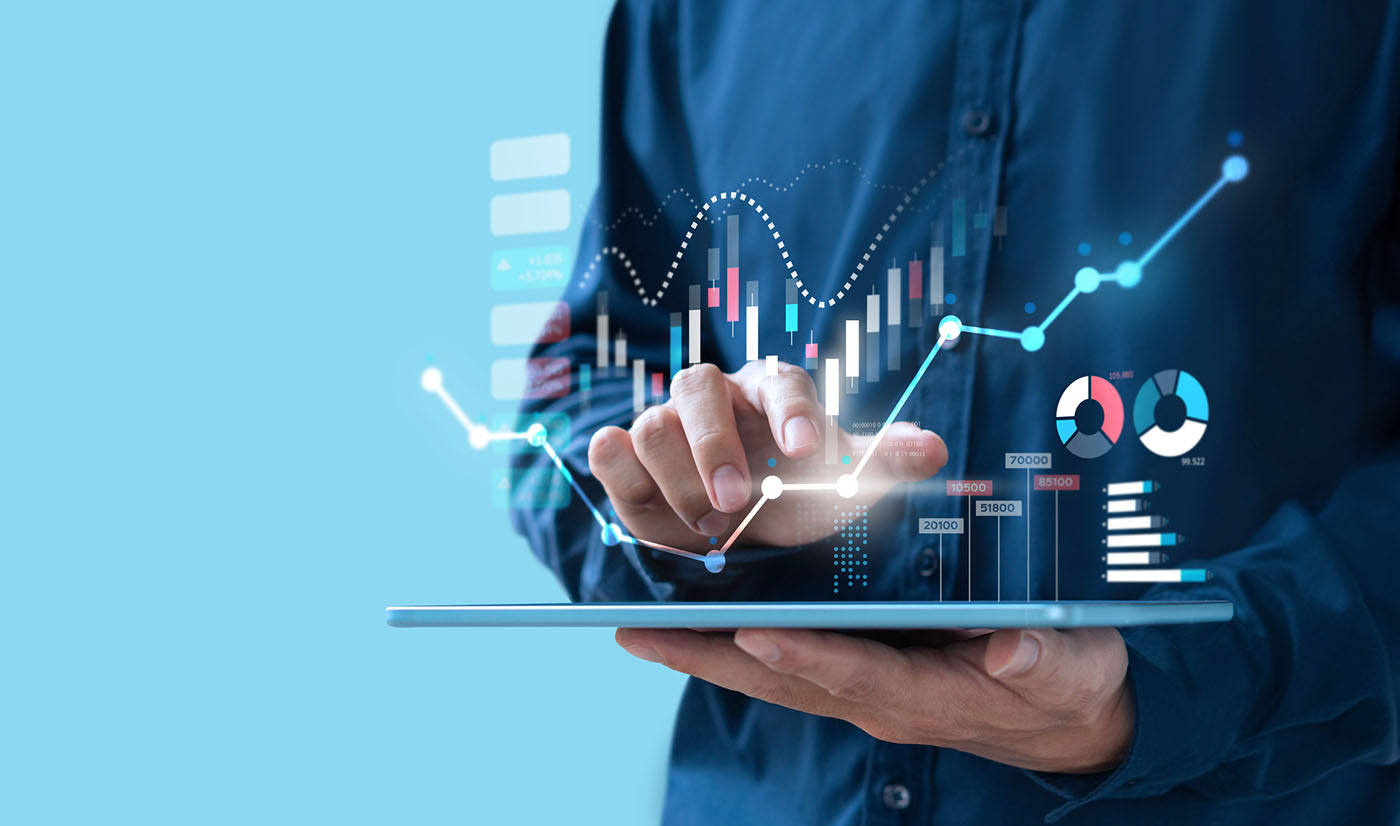
How do machine-learning (ML) models stack up against more traditional credit risk approaches?
Just a few weeks ago, the EBA released a discussion paper on the use of ML techniques for the calculation of regulatory capital. Banks have been wary of adopting such tools for this purpose, mainly because of difficulties associated with model interpretation.
Tony Hughes
While the EBA's paper provides an excellent discussion of the opportunities and challenges of using ML methods, and should encourage banks to explore ways to harness the improved accuracy that these techniques often provide, there are a couple of important issues that the regulator overlooked. They both relate to possible weaknesses in the way models are normally validated across the industry, regardless of whether they are ML-based or "traditional" parametric specifications.
Though I'm aware of recent efforts to improve the interpretability of ML approaches, for the purpose of this discussion, I'm going to assume that ML models are purely algorithmic and completely opaque in nature.
Given these conditions, attempts to truly understand all the implications of ML models will generally be futile, even as users try to rationalize the output. Indeed, the results of such validation efforts may offer some pearls of wisdom - but will also likely yield a lot of uncertainty.
In short, the aspects of a ML model that are most relevant, from a business risk point of view, are usually difficult to discern. This is one of the issues that was not directly addressed in the recent EBA paper.
For ML models, rather than trying to understand implications, a more productive approach to model validation involves the end user attempting to comprehend the rationale behind the estimation algorithm.
Validation efforts should not focus on the final specification, per se, but on the selection process that generated it. If it is repeatable and consistent, it is reasonable to infer that any model that emerges from a faithful application of the procedure will be trustworthy.
This notion of assessing the process, rather than the output, is common in statistical theory - but less common in industry practice. Revalidation is, in fact, necessary every time even minor changes are made to a traditional model's structure, because banks are typically required to sign off on such models. Lamentably, the associated costs of these efforts discourage banks from frequent model upgrades.
If no one understands the output an ML model, but it produces consistent, highly accurate predictions, revalidation serves very little purpose. An often-overlooked advantage of ML, therefore, is that it may reduce the administrative burden of model risk management.
I have often wondered whether traditional risk models could be validated in a similar (less burdensome) fashion to ML models. The answer, generally speaking, is “no.”
The problem is that traditional parametric approaches combine art and science, in contrast to the purely algorithmic number-crunching of ML. Since the more artsy aspects of model selection that cannot be faithfully repeated, traditional models really have to be individually validated every time the specification changes.
The Importance of Evaluating Hits and Misses
The other aspect overlooked in the EBA paper pertains to prior forecast appraisal. Leafing through the history books, and seeing how previous live forecasts performed in the field, is an informal process in most banks. Formal validation teams tend to focus on the next model; consequently, they rarely look back and compare the performance of the current model cohort to that which it replaced several years ago.
Some will say that this type of appraisal is what backtests do, but the process is subtly different. When building a challenger model on a training data set, modelers unconsciously borrow information that would not have been available to someone making predictions in real time.
It's easy to hide this information from an algorithm, but not from a human modeler, who may gain an advantage in the backtest that won't translate into the real world. Therefore, the only "pure" contest occurs when different forecasts are produced in real time and later compared against actual outcomes.
Risk analysts, strangely, tend not to reminisce about past forecasting exploits. I find this this quite odd, because you can learn a lot by examining hits and misses, and then using them to identify possible blind spots and hidden strengths.
Suppose a bank, for example, can access 15 years of records of past portfolio loss forecasts.
If they wanted to compare these against a ML challenger, they could do this by mimicking previous live forecasts generated from the algorithmic process. If the ML model defeats the bank's champion model in, say, 12 out of 15 prior years, and if the ML approach also wins three out of four recession-tainted contests, you may conclude that the more technical model is simply better at forecasting.
In such a situation, the contest output could be translated into associated capital costs. You could then crunch the numbers and determine, with considerable precision, the opportunity cost of model interpretability.
I suspect this number would be quite high for many portfolios. This then begs the question: How much is model transparency actually worth? It’s not infinite. If you found a hefty price tag, it would be difficult to argue that the traditional, interpretable approach was still worthwhile.
Parting Thoughts
Everything has a price. I suspect that most bankers value model interpretability quite highly and therefore view the promise of ML as slightly overblown. But it's hard to reach this conclusion without considering the costs (money and resources) of validation and without appraising model forecasts.
The price of interpretability may be low or high; either way, it would be a good number to get to know.
Tony Hughes is an expert risk modeler for Grant Thornton in London, UK. His team specializes in model risk management, model build/validation and quantitative climate risk solutions. He has extensive experience as a senior risk professional in North America, Europe and Australia.