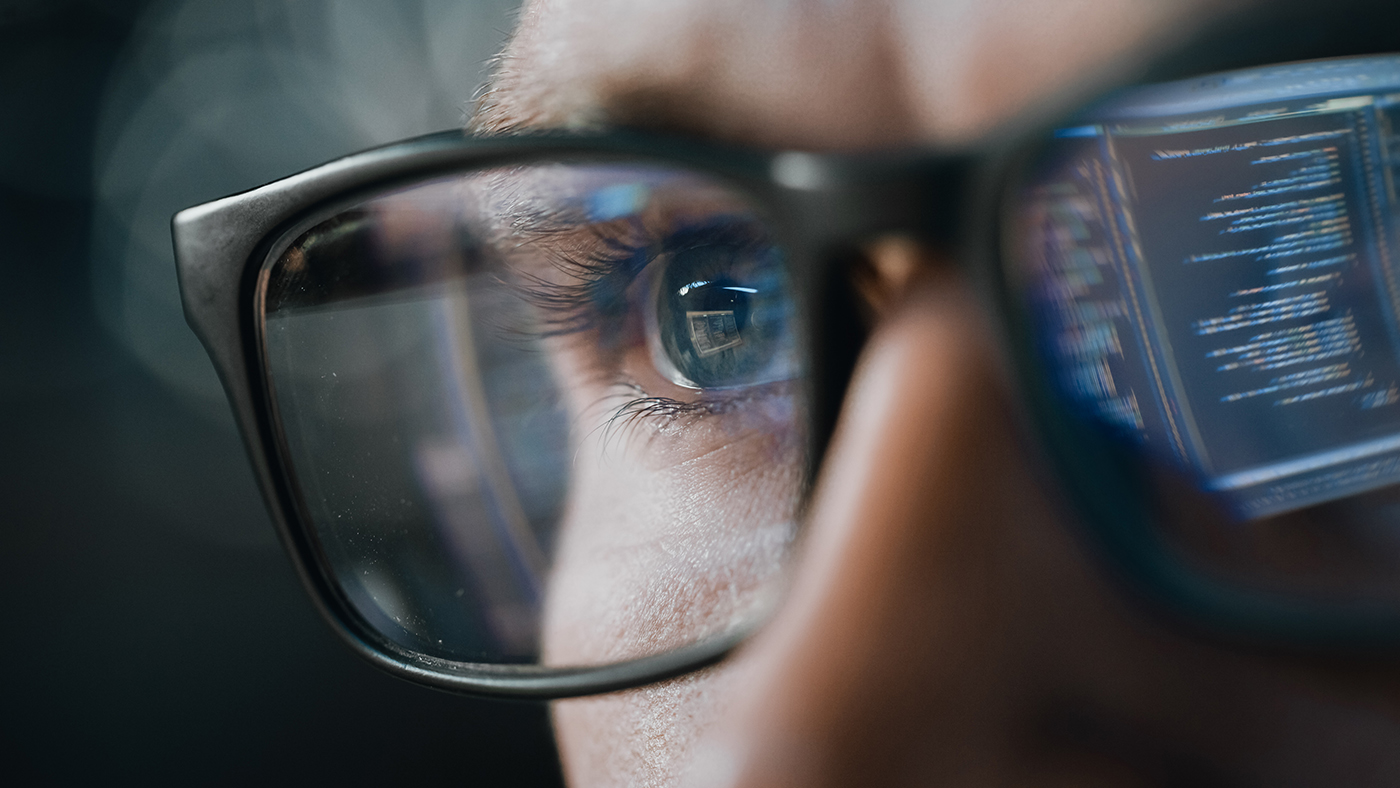
Today, we find our profession at a critical inflection point with the rise of “Big Data” and artificial intelligence applications. Indeed, thanks primarily to advances in computer software and hardware technology, analytic models have become a mainstay for risk managers
Increasingly, we hear how these analytical capabilities will make us better risk managers. But technology-driven models are not without their flaws, and if we fall into a model narrow-mindedness quagmire, there is a real danger that just the opposite will happen.
Risk Modeling: ML Pros and Cons
The acceleration of analytical tools and data science methods to quantify risk is one of the biggest trends in the risk management profession. Through machine-learning tools that can detect subtle but important behavioral and purchasing patterns, we have greatly enhanced our ability to identify and measure risks (e.g., fraud) in a manner that only a decade ago or so would have been difficult – if not impossible – to accomplish.
Clearly, disruptive technologies are critical in advancing our profession’s ability to measure risks, both observed and unobserved. However, the recent pandemic and the associated fiscal and monetary response – coupled with severe supply-chain disruptions and a war raging on the European continent – have placed risk managers in an uncomfortable position.
Essentially, they have to rely on historical-data-driven models (which lack relevant information on current and prospective economic conditions) to quantify risk outcomes.
This is where the danger lies for risk managers. To augment models, situational risk awareness, judgment and experience are still needed. Few modelers, however, have lived through a period of high inflation.
The temptation is therefore great and natural to the analytically oriented risk professional to gravitate to sophisticated algorithms for explanations to complex issues – including problems that may be outside of the modeler’s experience.
Clifford Rossi
However, there are two flaws in this approach. The first is that some of these new techniques, such as machine learning, are black boxes. While these tools are extremely useful at assessing nonlinear relationships in data, and may make you a good risk modeler, they will not necessarily make you a good risk manager or analyst.
Effective modeling goes beyond understanding data structure and model algorithms; one must also comprehend the business suitability of the data, and develop hypotheses for key risk relationships based on this data.
Shiny Object Bias
The second issue is one that we all, in varying degrees, suffer from: a bias toward analytical models.
Many hiring managers today would agree that recruiting for risk modeling talent has become a highly competitive and challenging process, due to the demand for such staff across industries. The technical level of expertise required to build and deploy risk models is high, and often requires an advanced degree in some quantitative methods field.
Teachers of these advanced degree programs (myself included) typically school students in the mathematics and techniques needed to master these models, leaving it to employers to fill in the details on interpreting model results from business and risk management perspectives. Taking this into account, it’s helpful to step back and consider how machine-learning models stack up with more traditional methodologies.
Historically, we’ve relied on statistical models to measure various risks. These models have the benefit of allowing the modeler to apply intuition and experience, based on established theoretical relationships, to their model specification.
Take, for example, the development of a model to measure the default risk of a borrower on a mortgage. We expect such factors as a borrower’s willingness to pay, ability to pay, and equity stake (down payment) to be important drivers of default. We can then customize the model in a way to represent these and other factors in a manner that reflects historical patterns in the data, which can be used to generate estimates of borrower default.
One of the benefits of machine learning is that some of its methods (e.g., decision trees) can identify combinations of attributes that may be difficult to surface in a statistical model. A tradeoff, however, is that while machine-learning models can provide insight on what factors are important to the results, they are not well-suited to understanding the specific impact a risk factor has on an outcome of interest, such as default.
In a statistical model, I can directly understand, for example, that the risk of a 620 FICO borrower is X times riskier than a 720 FICO borrower, holding all other factors constant. That is not something that is readily understandable in a machine-learning algorithm.
I fear that even the term “machine learning” conveys some notion of unimpeachable analytical integrity. Ultimately, this can yield a form of cognitive bias, which I refer to as “shiny object bias.”
We have been down this road before. During the years preceding the 2008 financial crisis, for example, we embraced the technical elegance of value-at-risk (VaR) models, only to be betrayed by their assumptions of normality and their implications for tail risk. This type of bias affects both model developers and users, and is something that must always be considered.
Cultivating a Culture of Responsible Risk Analytics
Thanks to a multiplicity of physical, geopolitical and economic risk drivers affecting markets, the current risk landscape appears to be one of the most challenging risk managers have experienced in decades. Understanding how these factors will affect risk outcomes is critical, placing enormous pressure on risk managers to come up with analytical innovations to quantify risks accurately in a dynamic environment.
Risk analysts must creatively develop models that have a solid economic and business rationale. What’s more, to better understand the limitations of their models, they must develop a healthy degree of objectivity toward model outcomes.
While it’s important to harness artificial intelligence to navigate all of the uncertainty in today’s markets, the real key to success is ensuring that your organization not only has the technical expertise but is also cultivating a culture of responsible risk analytics.
Clifford Rossi (PhD) is a Professor-of-the-Practice and Executive-in-Residence at the Robert H. Smith School of Business, University of Maryland. Before joining academia, he spent 25-plus years in the financial sector, as both a C-level risk executive at several top financial institutions and a federal banking regulator. He is the former managing director and CRO of Citigroup’s Consumer Lending Group.