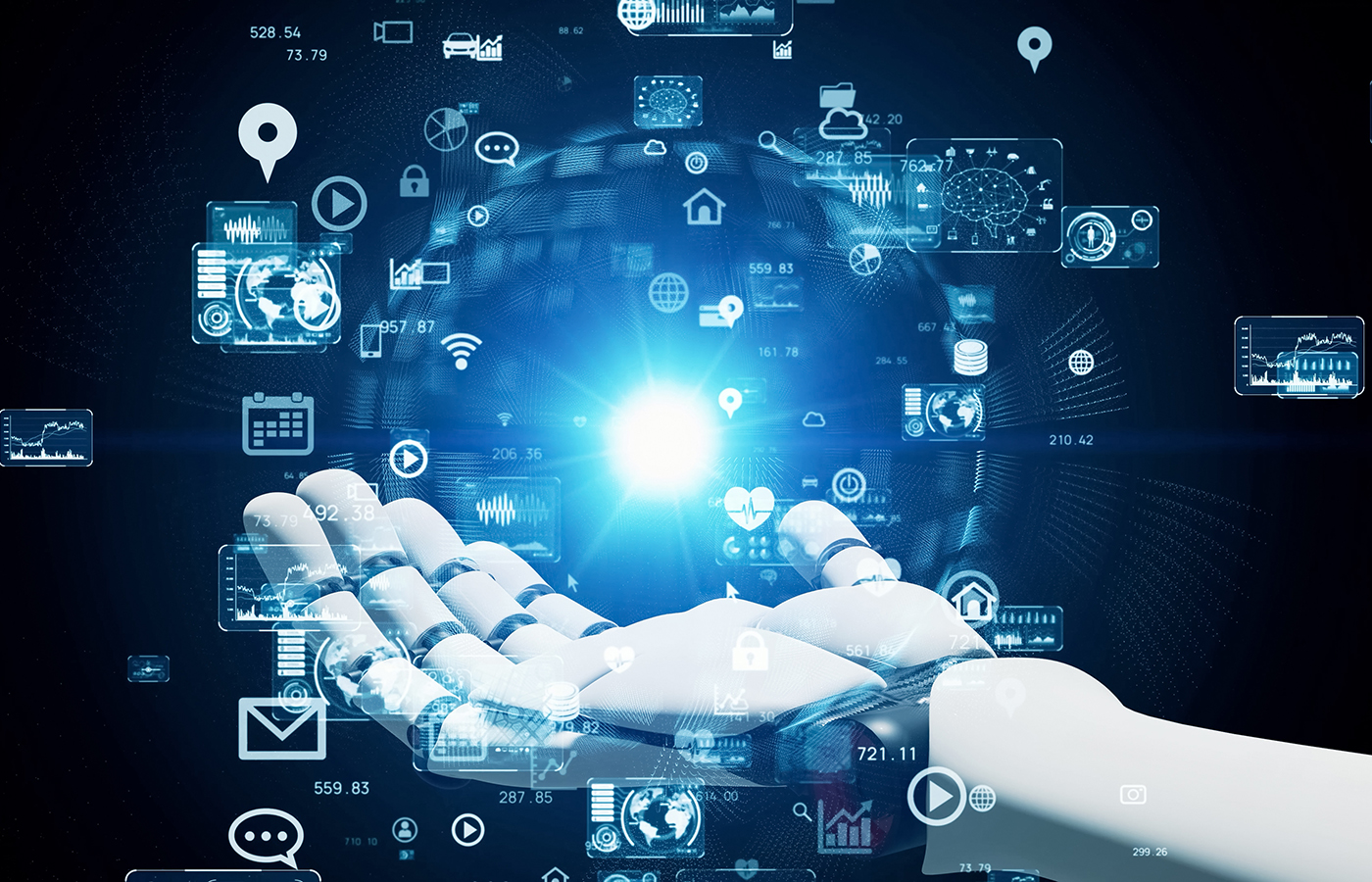
Machine learning (ML) is one of today’s hottest topics within the financial services modeling community. This technology holds great promise to expand credit availability, reduce losses and increase process efficiency. At the same time, the complexity and lack of transparency of ML techniques pose significant challenges to companies looking to harness their power.
Clearly, the era of the data scientist is upon us – but how do we avoid overreliance on technology, or something I like to call the risk mechanic syndrome in ML? To start, we need to understand that ML-driven models require specialists equipped with the expertise to develop them properly. Moreover, we can apply lessons learned from other fields where mechanics work.
The Data Science Overreliance Dilemma
The other day, I took my 1966 Mustang in to have an oil change and transmission pan gasket replaced. A relatively simple task, or so I thought. My mechanic, who has been a master technician at a major automotive dealership for 20 years, told me that while the service was complete, he couldn’t get the car to run any more.
After two weeks of testing, he finally found the culprit: a bad neutral-safety switch, which was something I had asked about at the onset of the diagnosis. My mechanic explained that he wasn’t used to seeing these kinds of issues, since he primarily works on cars where he can use a scanning tool and read a code to diagnose the problem.
With advances in automotive mechanical diagnosis, we’ve effectively atrophied any critical-thinking skills from that profession. Importantly, we need to avoid doing the same in relying on data science in managing risk.
Don’t get me wrong: data science is an incredibly important endeavor that requires extraordinarily talented people to develop these next-generation, ML-driven analytical tools. However, it is rare to find a data scientist with significant formal training in finance or economics – fields that form the foundation of any useful risk or financial model.
“Shiny Object” Bias, and the Best/Worst Fit for ML Modeling
Companies are now scrambling to recruit data scientists into their organizations, with the expectation that they will revolutionize modeling in banking. But there are practical considerations in how and where to leverage these talents.
Financial services companies have historically suffered from a type of cognitive bias toward complex analytical models – something I refer to as “shiny object” bias. Whether it was value-at-risk (VaR) models that assumed a normal distribution, or Gaussian copula models that assumed static correlations, management teams leading up to the 2008 financial crisis became overly enamored with the latest shiny object.
Unfortunately, modeling marvels don’t always live up to the hype, since these tools after all are only representations of behavior; markets and conditions can and will change, rendering most deployed models inaccurate at some point.
ML models are the latest shiny object. To be effective, they require close monitoring; a heavy dose of credible challenge by boards; and model risk oversight teams and staff trained in methods, applications and risk management.
Areas where data science can have immediate impact in organizations are on functions that are business-process related. Fraud detection is a great example of a process in which advances in ML and artificial intelligence have reshaped the way companies can proactively and analytically manage their fraud risk.
However, when it comes to, say, lending decisions, particularly for consumers, data science needs to be guided by risk management principles, business expertise and financial theory.
Clifford Rossi
Data science is all about using sophisticated tools to identify patterns in large datasets, where standard parametric models are unable to detect important nonlinear or interaction effects between variables of interest. The problem is that unless you have formed some theoretical rationale for why certain variables should be in a model and what their relationship is to the outcome of interest (such as mortgage delinquency, which at its foundation is based on option theory), then you basically have technicians developing models with little understanding of the principal drivers of default. That exposes your organization to substantial model and regulatory and compliance risk.
Fair-lending testing is a difficult process, but the relative lack of transparency of ML amplifies that difficulty. While advances in diagnostic inference tools that can discern the effect of important features in ML models have been made, they have yet to be perfected.
This leaves these methodologies vulnerable to supervisory scrutiny in the future. Indeed, models with far greater transparency and statistical diagnostics have already been subject to intense regulatory review.
Risk Staff: The Need for Education, Integration and Cross-Training
Most companies have begun creating blended model development organizations where data scientists, economists and statisticians work alongside each other. That’s a good starting point at firms where limited crossover skills between employees exist.
One step further would be for companies to cross-train their data scientists in the fundamentals of economics and risk management – and likewise train their economists and statisticians in basic data science concepts. Ideally, such organizations should look to universities that offer finance and risk management degrees, emphasizing the use of ML techniques in business applications. This would cut down on the need to train data scientists in finance and economics, leading to greater hiring efficiency.
When I was a CRO, I recall how difficult it was at the time to find modelers with excellent data skills, so we tended to hire specialists in each of those areas, which was inefficient.
Today, programs exist where graduate students in finance receive extensive training and practice at developing and interpreting the output from ML models. An emphasis on interpretation is key to building a team of ML-savvy risk analysts, rather than risk technicians.
Parting Thoughts
While the financial services industry has lagged a bit in adopting ML technologies, it is rapidly ascending the curve, as the benefits of these tools become more widely accepted. Understanding where data science can have the greatest immediate impact, while simultaneously reducing model risk and compliance risk, is key to leveraging these powerful capabilities.
Process-oriented activities with substantial structured and unstructured data are terrific candidates for ML and data scientists. On the other hand, in areas requiring extensive business and risk domain expertise (such as consumer lending), companies need to ensure their ML modeling teams are dominated by well-rounded risk analysts, rather than mechanics who are overreliant on technology.
Clifford Rossi (PhD) is a Professor-of-the-Practice and Executive-in-Residence at the Robert H. Smith School of Business, University of Maryland. Before joining academia, he spent 25-plus years in the financial sector, as both a C-level risk executive at several top financial institutions and a federal banking regulator. He is the former managing director and CRO of Citigroup’s Consumer Lending Group.
Article Updated: January 20, 2023