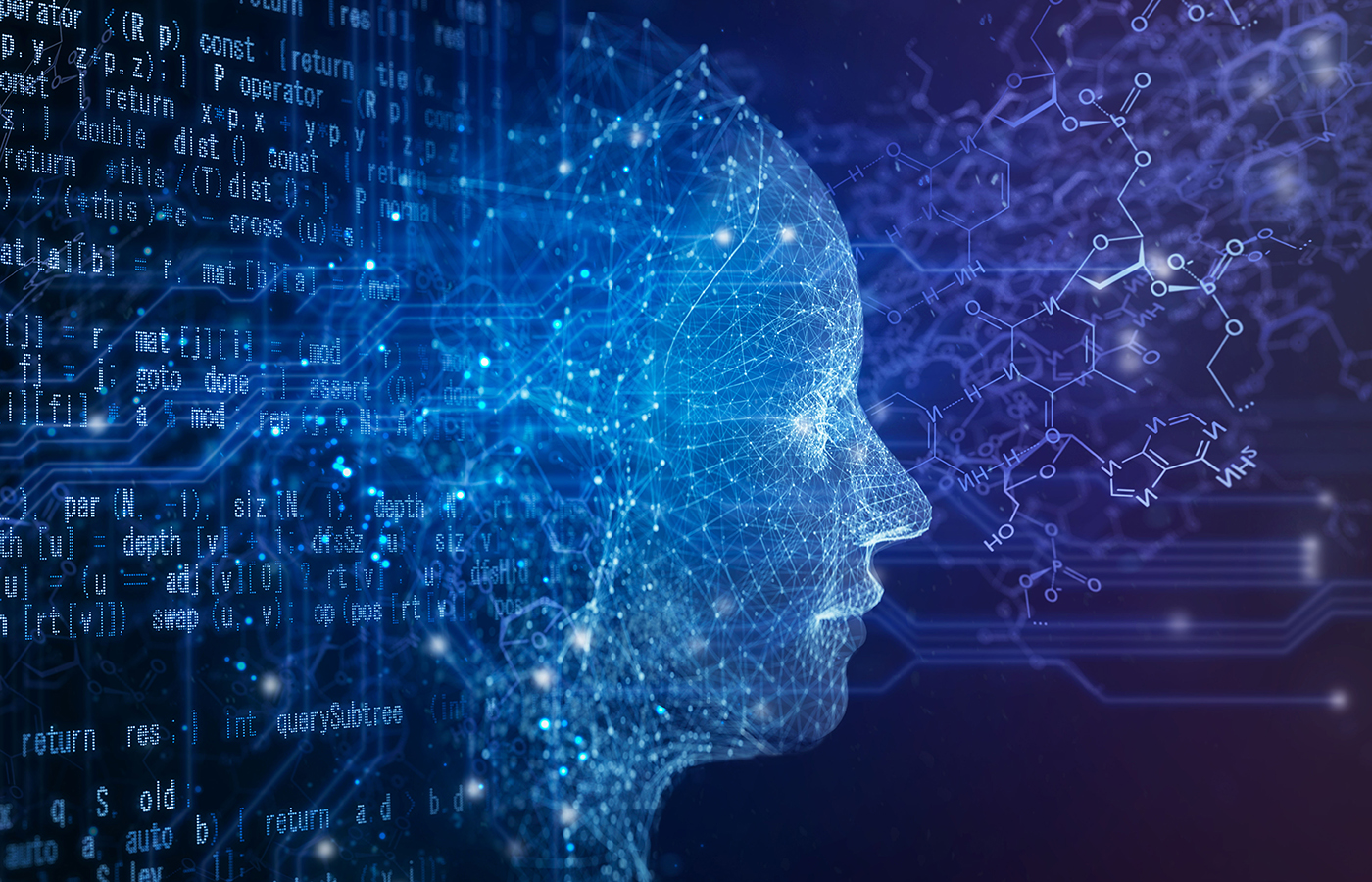
Artificial intelligence is touted as a productivity enhancer and labor saver, but how solid is the evidence? Far away from the big financial centers and the institutions that invest most heavily in technology, Carter Bank and Trust makes a convincing case.
The community bank, headquartered in Martinsville, Virginia, identified more than 200 processes to hand off to AI and machine learning (ML) in such areas as customer onboarding, anti-money laundering (AML) and Know Your Customer (KYC) compliance. Within a year of deploying the “digital workforce” capabilities from its vendor, WorkFusion, Carter tallied up to $3 million in cost savings and freed up 40 employees to perform higher-value, instead of repetitive manual, tasks, according to a WorkFusion blog.
“They realized the most business impact in AML/KYC processes by automating account opening reviews, identity verification, adverse media monitoring, and fraud due diligence,” the company said. Although its customers include top-tier financial services companies, and it claims to have saved users more than $100 million in total, WorkFusion can point to the $4 billion-in-assets Carter Bank & Trust as a microcosm of the converging pressures of operational complexity and regulatory scrutiny and accompanying cost increases.
Those are compounded by exposure to penalties for compliance shortfalls. Client lifecycle management company Fenergo said regulators’ enforcement fines totaled $4.2 billion in 2022; that was down 22% from 2021, but those for AML-related breaches jumped by 52%.
Over-Stretched Staffs
Russia’s invasion of Ukraine was a turning point, at least where AML and sanctions compliance are concerned.
Daniel Hazel of WorkFusion
Looking back from the one-year mark, WorkFusion vice president of strategic accounts Daniel Hazel said, “Prior to February 24, 2022, AML teams within banks and financial institutions were already overburdened, overstressed and under-motivated. Once the war began, these stressors were compounded tenfold. And, adding fuel to the fire, these overburdened teams now had to deal with pressures from executive teams and boards as sanctions governance became a boardroom agenda item.”
It brought heightened demand for the company’s Tara and Evelyn AI screening solutions.
AI-assisted or not, such applications must meet ongoing anti-fraud and -financial crime challenges.
Established system providers like NICE Actimize (see its recent release of an AI Money Mule Defense Solution) and the London Stock Exchange Group’s Refinitiv have stepped up accordingly. The latter in 2020 acquired U.S.-based anti-fraud, ID and transaction verification provider GIACT and integrated it with risk and compliance offerings World-Check, Qual-ID and Enhanced Due Diligence. GIACT this year partnered with Mastercard Open Banking for secure account verification.
Quantifind of Palo Alto, California, on March 1 announced a $23 million fundraise, with Citi Ventures and S&P Global among the investors, to expand and enhance its AI-powered financial crimes investigation, continuous customer monitoring, alerts triage and supply chain risk screening solutions.
In addition to signing four of the world’s biggest banks last year as customers, Quantifind quoted a testimonial from a top Canadian bank: “Quantifind is an essential part of our risk management strategy. We see upwards of 40% productivity gains for investigations and 75% of high-risk cases automatically triaged.”
Accumulated Experience
Machine learning models have been used in the payments fraud area for more than 20 years, says SAS financial crimes and compliance expert David Stewart. “Supervised learning methods like neural networks have proven to be effective at identifying known types of fraud, especially card fraud and digital payments,” he points out. “For anti-money laundering, customer due diligence and sanctions compliance, we are seeing more financial institutions adopt machine learning methods.”
Dhanum Nursigadoo of Sentinels
In AML and KYC, ML can be used to fine-tune transaction monitoring and risk-rating models and can improve entity matching techniques along with natural language processing (NLP), another branch on the AI tree.
“We believe that human-only operations are losing the fight against financial crime,” says Dhanum Nursigadoo, senior content manager at AML transaction monitoring company Sentinels, which Fenergo acquired last year. The United Nations Office on Drugs and Crime in 2011 found “that 2% to 5% of global GDP per year was illicit cash moving through the financial system, as much as $2 trillion. And less than 1% of that is ever detected or caught.”
Nursigadoo maintains that significant strides have been made in the years since those calculations.
“Automation, improved workflows, pattern identification and stricter regulations have all had a real impact in making it harder for criminals to move money illicitly,” he states. “But it’s not enough. For the past several years the industry has looked to AI as a powerful tool in our ability to fight financial crime.”
Machines Versus Humans
Despite the demonstrable progress of AI, Nursigadoo stops short of believing that it will replace human compliance staff.
Jeremy Tilsner, a senior director in Alvarez & Marsal’s Disputes and Investigations practice, says that to measure the effectiveness of AI tools, one should ask: How do the judgments of predictive models compare to those of human counterparts, and is model performance stable over time?
Jeremy Tilsner of Alvarez & Marsal
On the first part of the question, “standard practice should include regular, second-pass reviews of a sample of outputs (e.g., communications surveillance alerts) by experienced human reviewers,” Tilsner explains. “Significant divergences between human and AI judgments are a sign of trouble and should garner the interest of compliance and risk managers. This is of particular importance in niche domains, like fraud detection, that frequently lack robust training data and in which human subject matter experts’ input is critical.”
On the second question, he goes on, “large, rapid shifts in model outputs are potential signs of poor model design. Model outputs should be monitored for volatility at all times and should be further stress-tested by the regular input of large volumes of test data. Test data should reflect a wide variety of potential real-world conditions.”
Satisfying Regulators
Carl Case, U.S. financial crimes technology consulting leader at EY, points to the need to validate the advanced tools in order to satisfy the expectations of regulators and their compliance oversight.
“AI tools or models are generally subject to periodic model validation,” Case notes. “This validation tests the accuracy of the inputs, processing, and outputs for any system, and typically requires robust documentation of the AI’s development, implementation and use. Additionally, model transparency and explainability have become standard practice, allowing end users to better understand, and ultimately defend, the recommendations and decisions made by an AI solution. Put another way, the days of proprietary, ‘black box’ AI in the compliance space are largely past.”
Fred Curry, AML and sanctions client leader, Deloitte Risk & Financial Advisory, sees AI improving AML compliance and outcomes. However, it’s important to note that regulators have been critical of organizations that rush into AI solutions without recognizing that they demand skilled teams, including data scientists, along with necessary governance, oversight and risk assessments.
Fred Curry of Deloitte
“Within data quality needed for AI application to AML efforts are a need for effective customer identification and verification, due diligence, and ongoing customer information refresh, as well as standardized data collection and structures and consistent AML operating standards and practices,” Curry says.
Data Quality and Trust
The quality of the underlying data and the processes applied via AI must be substantiated and transparent to those who trust in their reliability.
“Documentation is key," says Jeffrey Feinstein, vice president of global advanced analytics strategy, LexisNexis Risk Solutions. "A responsible AI program includes human oversight and documentation so that analytic solutions can be explained to users and regulators.
“This is particularly important when generating model variables and scores, testing variables and scores for bias, reducing false positives and ensuring that the outcomes are transparent and explainable. It’s also important to develop a review and oversight function separate from the development function to oversee and confirm that these processes are being adhered to.”
"No system is perfect, which is why many financial institutions face compliance deficiencies in routine exams," says Kennyhertz Perry partner Braden Perry. "Monitoring transactions becomes difficult with practices like trade-based money laundering. This makes it necessary for financial institutions to adopt better technologies to help compliance and risk teams.
“Many technology trends are changing the way institutions gather, verify, screen, monitor and store customer information, and a proper system can make regulators more comfortable as data increases,” Perry adds.
“Both companies and regulators must understand that automation is the key to the future,” the attorney continues. “But automation can only go so far, and traditional ‘boots on the ground’ compliance will always be a key to a proper program. There is no one-size-fits-all approach, and a custom compliance plan designed to efficiently manage risk can be presented to regulators in a way [that] uses technology and human intel in a novel way to trust and verify.”
Topics: Data & AI Model Governance