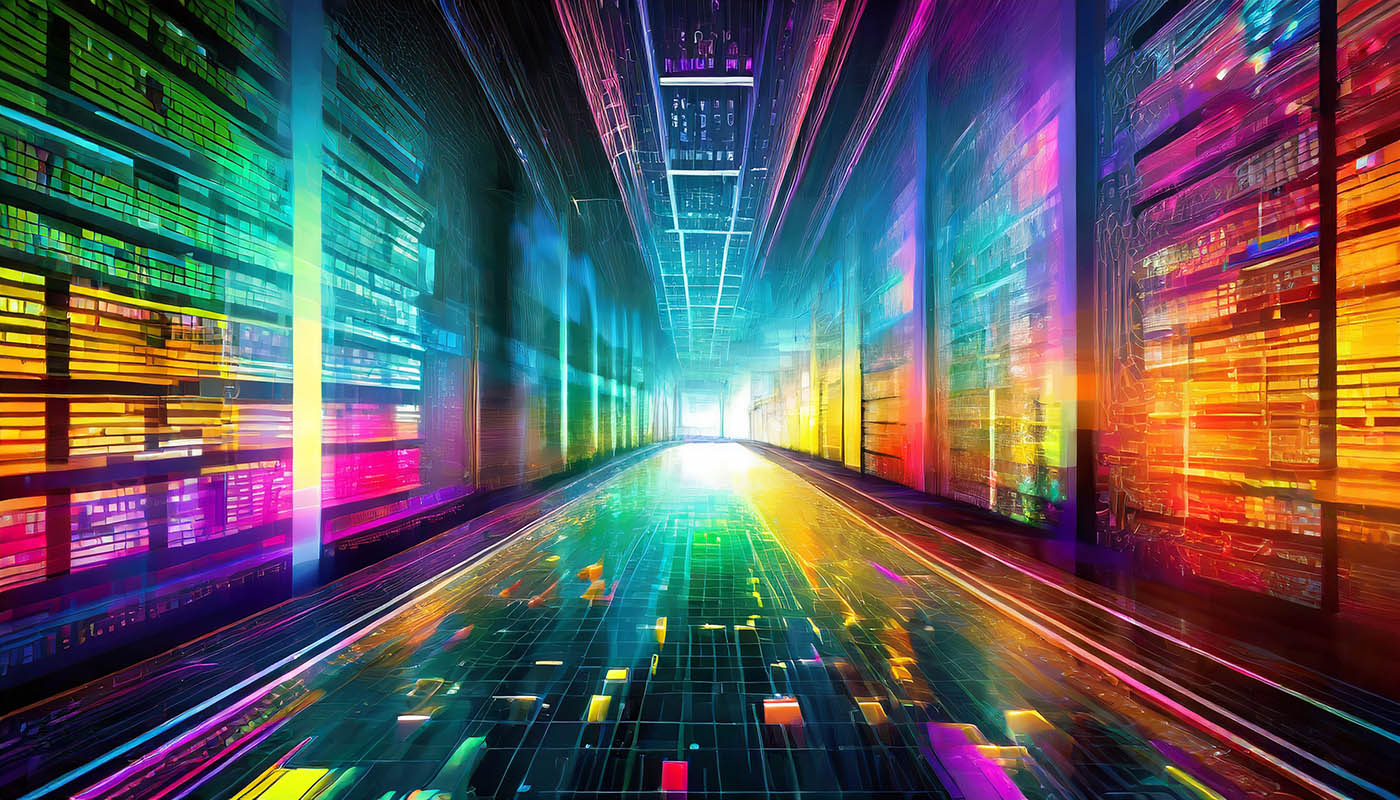
Developing sector-driven correlation matrices is no small task for risk modelers. Traditionally, these modelers have used a combination of equity return data and expert judgment to measure correlations between different sectors – but this approach is imperfect.
Fortunately, thanks to the emergence of generative AI, there is now an alternative for building correlation matrices. ChatGPT, for example, can already provide relevant information on sector correlations, as well as technical assistance for the necessary “cleaning up” of the matrix.
Dr. Marco Folpmers
Generative AI is not yet fully evolved, but when it matures, it has the potential to significantly improve the process of building sector-driven correlation matrices – via eliminating, or at least decreasing, the current over-reliance on expert judgment.
We’ll examine the modeling benefits of generative AI more closely in a minute. First, though, let’s provide a bit of background about the obstacles risk modelers face in the traditional process for developing sector-based correlations.
The Sector-Driven Correlations Challenge
For many financial risk management applications, risk modelers need forward looking scenarios. These are often broken down per sector and per country. For the future performance of, say, a wholesale portfolio, a bank can assess how the sectors to which it is exposed (e.g., agriculture, mining, financial services) will perform in the coming years.
These performance outcomes, per sector, are then used to model the systematic risk component of the probability of default (PD) of the individual obligors. If, say, the agricultural sector is projected to have a difficult time, this will increase all PDs of the individual farming clients, because of their systematic risk component. (The PDs do not necessarily increase by the same amount, because modelers also consider idiosyncratic risk.)
The big difficulty for risk modelers is to generate scenarios, per sector, in which the outcomes have the appropriate correlations. Sector performance is highly correlated, because of strong interlinkages between the sectors and the economy at large.
The traditional FRM problem is how to measure these correlations between sector performances. Usually, modelers resort to equity returns. However, these equity returns are not always available for each sector and each country. Consequently, banks often look at a combination of data-driven statistics and expert assessment.
The Imperfections of Expert-Based Correlations
Before one can work with expert-based correlations, they need to be cleaned up. More specifically, the correlation matrix needs to have internal consistency. If, for example, sectors A and B are both highly correlated to sector C, one would also expect a nonzero correlation between A and B.
Technically speaking, the correlation matrix must be positive definite. We can illustrate this with the help of an example. Suppose that we have six sectors that are subsequent stations of a supply chain – e.g., mining of raw materials, refinement, manufacturing, distribution, wholesale and retail. Our experts may say that the financial performance of each sector is highly correlated by the performance of sectors that are immediately upstream and downstream. Otherwise, the correlations are zero.
The correlation matrix would then look like the one on the left-hand side in the graph (Figure 1) below.
This matrix in Figure 1, left side, is both logically and mathematically flawed. We need to run a procedure to transform our matrix into a “proper” one – i.e., to make it positive definite. ChatGPT can not only explain this routine to you but also give you the relevant code in R.
Removing the Experts
The real added value for ChatGPT, of course, is that it potentially removes the experts. Though generative AI has not yet fully evolved, experience tells us that it gets you more than halfway there with respect to building proper correlation matrices.
It understands the principle of a correlation matrix for sector performance based on financial performance and realizes that this may be established with the help of a mix of equity return statistics and expert judgment. Moreover, it shares considerations about why a pair of sectors is highly or only moderately correlated, pointing toward economic factors that impact both industries at the same time.
According to ChatGPT, pairs of highly correlated sectors include technology and semiconductors; consumer discretionary and consumer staples; healthcare and pharmaceuticals; and energy and materials. When asked to expand on, for example, the high correlation between consumer discretionary and consumer staples, the system mentions reasonable drivers such as consumer confidence and interest rates.
However, the quantitative element of generative AI is not yet fully developed. When asked for quantitative correlations between sectors (values between -1 and 1), it is hesitant and often only provides broad ranges (between -0.5 and 0.5).
It is only a matter of time, though, before generative AI will have larger and more diverse databases to sift through to find the correct answers. Undoubtedly, future generations of this technology will give the credit risk modeler the information he or she needs.
Parting Thoughts
Generative AI will, over time, become more important to modelers. It will help them, for example, establish expert-based parameters (like sector correlations), while also providing the appropriate justification for these values.
It is still necessary for the risk modelers to be able to think as an economist. One needs to have the basic economic understanding on questions like how correlations between sectors may diversify risk or establish concentration risk. However, generative AI will provide more and more expert-like outcomes for traditional modeling questions, with the sector correlation matrix as a typical example.
As this innovative technology grows and becomes more accepted, it will improve the efficiency of the risk modeler. But there is one caveat: the risk modeler must adapt as this technology evolves.
To assess generative AI’s output properly, he or she must, for example, mobilize sector experts, collect the estimated correlations and clean up the correlations matrix, using both the code developed by generative AI and the parameters and justifications it establishes for sector-based correlations.
Dr. Marco Folpmers (FRM) is a partner for Financial Risk Management at Deloitte the Netherlands.
Article Updated: March 26, 2024
Topics: Tools & Techniques