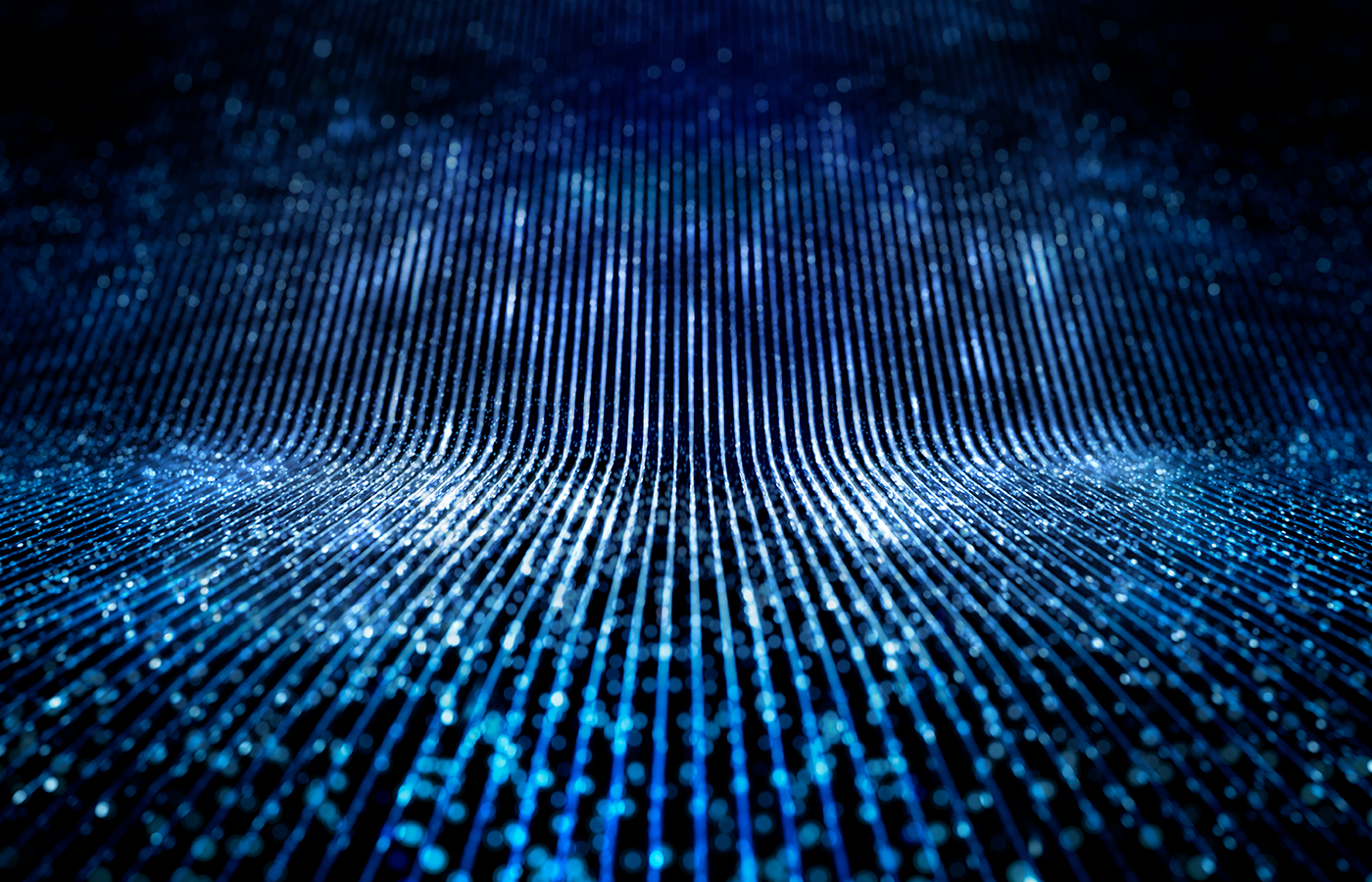
Where is artificial intelligence? It can appear to be everywhere – note all the rage surrounding ChatGPT and generative AI – and yet it is far from the maturity and ubiquity that its visionaries say is its world-changing destiny.
Likewise in finance, an early-adopting sector where “AI already is being used for call centers, account openings, compliance programs, trading algorithms and sentiment analysis, among others,” Securities and Exchange Commission Chair Gary Gensler remarked in recent congressional testimony. “It’s also fueled a rapid change in the field of robo-advisers and brokerage apps,” where predictive data analytics can “optimize for investor interests,” if properly programmed and governed.
AI and machine learning (ML) are assisting and accelerating high-throughput surveillance functions, such as in trading and anti-money laundering compliance, and are adept at scanning and sorting corporate announcements and news feeds.
“AI Can Write a Song, but It Can’t Beat the Market,” blared an April 12 Wall Street Journal headline, suggesting that high-stakes, risk-aware judgments as critical as securities selection and portfolio construction are as yet out of reach.
That hasn’t been for lack of trying, and there is movement.
Funds (left axis) and their assets under management (right axis, in billions of euros) using AI or ML, according to publicly available documents. Source: ESMA
“AI appears to be used mostly as a tool to execute specific tasks that leverage large amounts of data,” said Artificial Intelligence in EU Securities Markets, a European Securities and Markets Authority (ESMA) report published in February. “In fact, the ability to extract information efficiently from a wide range of large numerical and textual datasets with minimal human supervision is driving AI adoption – in at least some form – by an increasing number of funds.”
Demonstrating Predictability
In January, asset manager Amundi published a study in collaboration with AI research vendor Causality Link of the predictability of near-term stock price movements from textual news signals. Their sample encompassed more than 1.7 million signals for 4,460 U.S. stocks between January 2014 and December 2021. Variously categorized returns, such as average daily, short portfolio and long portfolio, depending on timeframe, ranged from 0.04% to 2.0%.
Putting it another way, Causality Link co-founders Pierre Haren and Eric Jensen wrote in RealClear Markets that “these signal-driven portfolios outperformed the market significantly, averaging an overall excess return of +1.3% per day . . . a powerful endorsement of using news media sentiment to drive investment decisions.”
In an experiment by finder.com, reported this month in the Financial Times, a theoretical portfolio of 38 stocks chosen by ChatGPT rose 4.9% over eight weeks. That “compared with an average loss of 0.8% for the 10 most popular funds on U.K. platform Interactive Investor.”
Examples of predictive AI and machine learning applications are on the increase.
Financial data marketplace and workflow platform S3 Partners in December announced a partnership with Boosted.ai enabling investors to use S3 short interest, financing rate, crowded score and squeeze risk data “to find alpha and mitigate herd risk.”
“With [ML platform] Boosted Insights and Hedge Baskets, Boosted.ai has developed a platform that the world’s most sophisticated institutional investors can use to improve their long and short positions through machine learning,” said S3 managing partner and CEO Bob Sloan. “The addition of S3 data further improves those outputs – revealing herd risk and sources of unexpected volatility while ensuring that the machine’s recommendations work well in practice.”
Seeking an Edge
Igor Halperin, an AI researcher and group data science leader at Fidelity Investments, has published on the subject of “combining reinforcement learning and inverse reinforcement learning for optimal asset allocation.” “We show,” according to a research abstract, “that our method is able to improve over the performance of individual fund managers.”
“Deep reinforcement learning models” are a hallmark of alternative asset manager Rosetta Analytics, which was co-founded in 2016 by Julia Bonafede and Angelo Calvello and says on its website that its advanced AI plus “the right team, partners and culture . . . rather than data will deliver a persistent investment edge.”
“AI modeling techniques share many similarities with classic statistical modeling techniques,” explains Margin Optimization AI, a paper by Overbond. “Statistics provides the building blocks upon which the machine learning that drives AI is built, and both use large amounts of data. But statistics is purely mathematical and primarily descriptive with some ability for inference. AI adds additional programming, made possible with modern computing power, to move one step beyond statistics and become predictive.”
David Curtis of bondIT
Investment technology company bondIT says it combines “data science, explainable AI and advanced technologies with fixed-income investment know-how” for accelerated credit-analytic insights. “While rising credit risk may present challenges, investors can still find opportunities to generate strong returns by effectively managing their exposures and building well-diversified portfolios,” David Curtis, head of global client business, said in presenting a recent quarterly forecast. “Anticipating directional credit changes early, regular risk monitoring and rebalancing, can help investors to stay on track towards their investment goals.”
Morgan Stanley Wealth Management described a strategic initiative with OpenAI, the ChatGPT creator, as a “key milestone . . . on the heels of several AI projects in recent years.” The intent is “to access, process and synthesize content to assimilate MSWM’s own expansive range of intellectual capital in the form of insights into companies, sectors, asset classes, capital markets, and regions around the world.”
Fintica AI, a “next generation autonomous AI” startup developing multi-asset “predictive analytics and risk decision-support tools for investment managers, financial markets infrastructure and digital stakeholders,” underscored its institutional intentions by appointing former SIX Swiss Exchange global head and Bank of New York managing director Thomas Zeeb as chairman of its advisory board.
“Compelling Opportunities”
“In recent months, we have seen a step change of technological innovation in artificial intelligence,” Nasdaq Chair and CEO Adena Friedman commented in presenting first-quarter earnings. “Nasdaq’s investments in proprietary data, and migrating our markets and SaaS solutions to the cloud, uniquely positions us to harness the potential of advanced AI to improve the liquidity, transparency and integrity of the financial system in the coming years.”
The exchange operator, which has applied AI in its anti-financial crime business, sees “compelling opportunities to leverage broader AI models” in such areas as deep reinforcement learning, predictive control and computer vision, Friedman added.
Nasdaq CEO Adena Friedman
Proponents of AI in investing point to the State Street Global Advisors (SSGA) SPDR S&P Kensho New Economies Composite ETF as an established success. The fund uses natural language processing to scan corporate regulatory filings and prospectuses. AI matches that information against defined standards for innovation to determine what is a “buy,” Matt Bartolini, SSGA head of SPDR Americas Research, told Bloomberg.
Through April, the ETF has delivered a return exceeding 9% since its October 2018 inception. Bartolini said AI was notably productive in analysis of large numbers of stocks and particularly small caps.
One who believes the future is here, or close, is David Csiki, managing director and president of investment management software company INDATA. “Recent developments have created significant momentum in AI,” Csiki told Markets Media. “This is sure to find its way into the specific systems and processes that are widely used on the buy side.”
He added: “Much like the cloud and data analytics, AI-based tools will become ubiquitous on the buy side, and early adopters will have a competitive advantage.”
Limited Penetration
ESMA’s survey suggested how far AI has to go. Only 65 of 22,000 EU-domiciled funds that it screened said they were using the technology. One explanation may be that AI is primarily being used in limited ways, rather than at broader strategy or policy levels. And the agency acknowledged that firms getting more involved with AI may not have been ready to disclose or discuss it.
ESMA called attention to operational, governance and policy issues raised by AI adoption, such as the possibility that proficiency could become concentrated in a few elite firms; the interpretability of models; and the hazards of feeding inaccurate, unreliable or outdated information into models.
Although AI is not yet widely disrupting investment management and trading, ESMA concluded, it can realistically speed up business and decision-making processes. Governance, regulation and supervision, among other factors in flux, could effectively hold it back.
Keeping Up with the News
Dan diBartolomeo, president of analytics provider Northfield Information Services, offers a more nuanced perspective on AI’s investment-industry acceptance and limitations. A Northfield product, Risk Systems That Read, was designed to “quantif[y] risk based on the quantity and type of news, providing a more accurate and rare understanding of risk. It delivers the stability of longer-term model forecast enhanced by rapid adaptation to changing market conditions and broad asset coverage.”
Large institutional investors seeking AI-assisted insights from news signals run up against the fact that their trades can have market-moving impact.
“It’s rather easy to predict share price movements from news,” diBartolomeo says. “The problem is that the speed and size of the price movements is such that once you factor in the transaction costs experienced by large investors, you can’t usually make material profits.
“A big institution would have to execute a big trade over the course of a day or more, and the price impact of their own trades reduces the profits. As an institution, if I think a stock is going up, I can only buy a fraction of the amount I want immediately – the supply is limited – which drives the price up before I can buy the remainder of the number of shares I want.”
What’s more, news signals can appear after an event has been priced into a stock. And stock picking generally attempts to incorporate a wider net of data beyond news signals. But now there are indications that AI-aided stock picking will result in better and more predictable investment performance.
Article Updated: May 22, 2024
Topics: Tools & Techniques